Data analytics accelerates the experimental discovery of Cu1-xAgxGaTe2 based thermoelectric chalcogenides with high figure of merit
JOURNAL OF MATERIALS CHEMISTRY A(2023)
摘要
Thermoelectric (TE) materials allow us to harvest energy practically from any heat source, including heat that would be otherwise wasted. This huge promise of energy harvesting is contingent on identifying/designing materials having higher efficiency than presently available ones. However, due to the vastness of the chemical space of materials, only a small fraction of potential candidates has been experimentally and/or computationally scanned thus far. By employing an artificial intelligence (AI) approach based on compressed-sensing symbolic regression analysis of experimental data in an active-learning framework, we have not only identified a trend in the materials composition for superior TE performance, but also predicted and experimentally synthesized several high-performing TE chalcogenides. In particular, p-type Cu0.45Ag0.55GaTe2 shows a very high experimental figure of merit (zT) & SIM;1.90 at 770 K using experimentally measured heat capacity (C-p). The present work demonstrates not only experimental realization of AI-predicted high-zT TE, but also the importance and potential of physically informed descriptors in material science, particularly for relatively small but well-controlled datasets typically available from experiments.
更多查看译文
关键词
thermoelectric chalcogenides
AI 理解论文
溯源树
样例
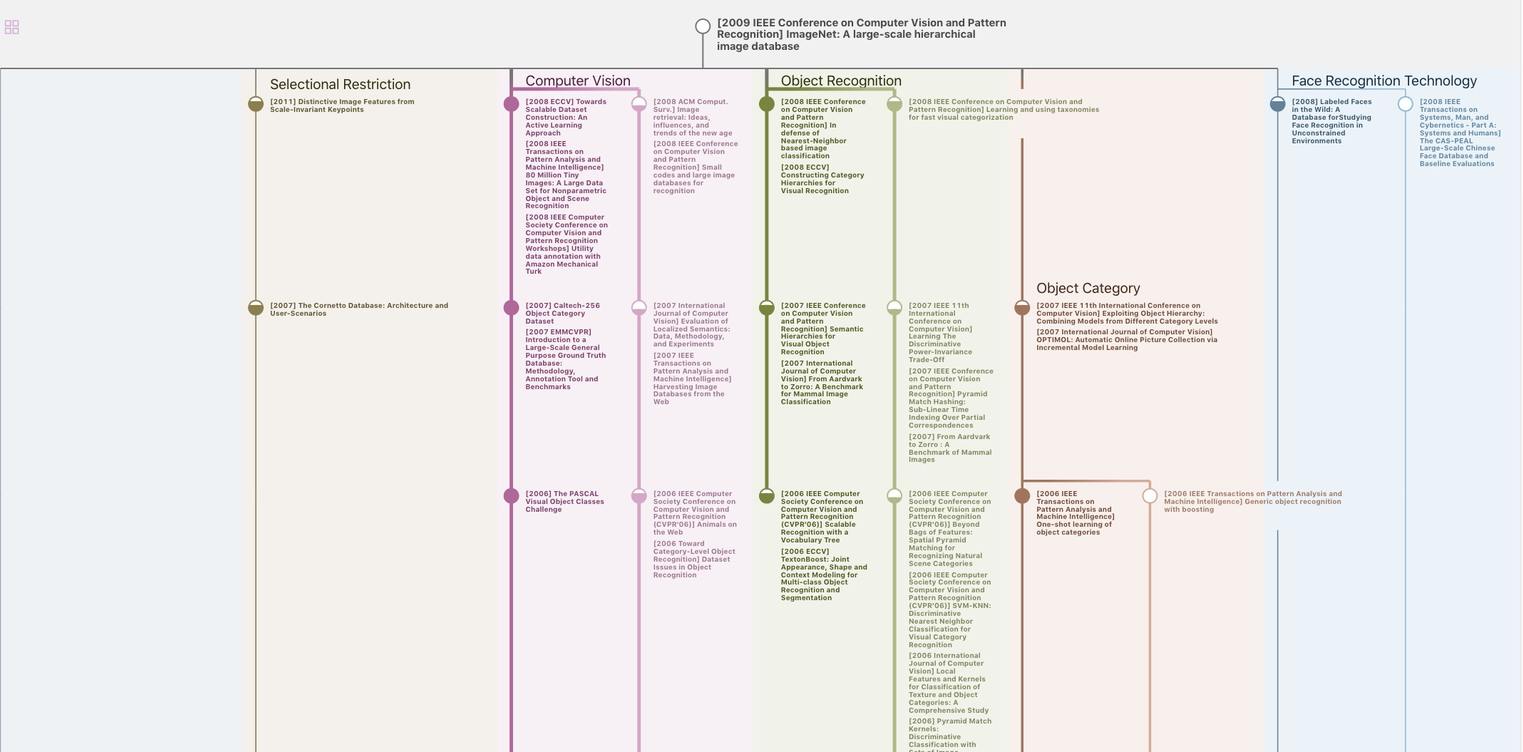
生成溯源树,研究论文发展脉络
Chat Paper
正在生成论文摘要