Lite Transformer Network with Long–Short Range Attention for Real-Time Fire Detection
FIRE TECHNOLOGY(2023)
摘要
With the popularity of video surveillance network, image-based fire detection method is of great significance to reduce the loss of public life and public property caused by fire disasters. Convolutional neural networks based on deep learning have been used in the field of fire detection. However, these methods directly use the existing object detection network, so there are some problems such as low detection accuracy, slow speed and high calculation cost. In this paper, we propose a cost-efficient neural network based on long-short range attention for real-time fire detection. First, we design a light-weight backbone network to extract multi-scale fire features with lower computational cost. Secondly, we introduce the transformer module into the convolution layer and construct an long-short range attention block, which can extract the global attention independent of distance to assist the network in identifying fire and background. Finally, the feature fusion module is constructed to process the multi-scale features extracted by backbone, and improve the detection effect of different size fires, especially early small-size fires. The experimental results show that our network can accurately detect fire with very fast speed and very low calculation cost, and the false alarm rate is lower. At the same time, it also has significant advantages for the detection performance of early small-size fire.
更多查看译文
关键词
transformer,fire,long–short range attention,detection,real-time
AI 理解论文
溯源树
样例
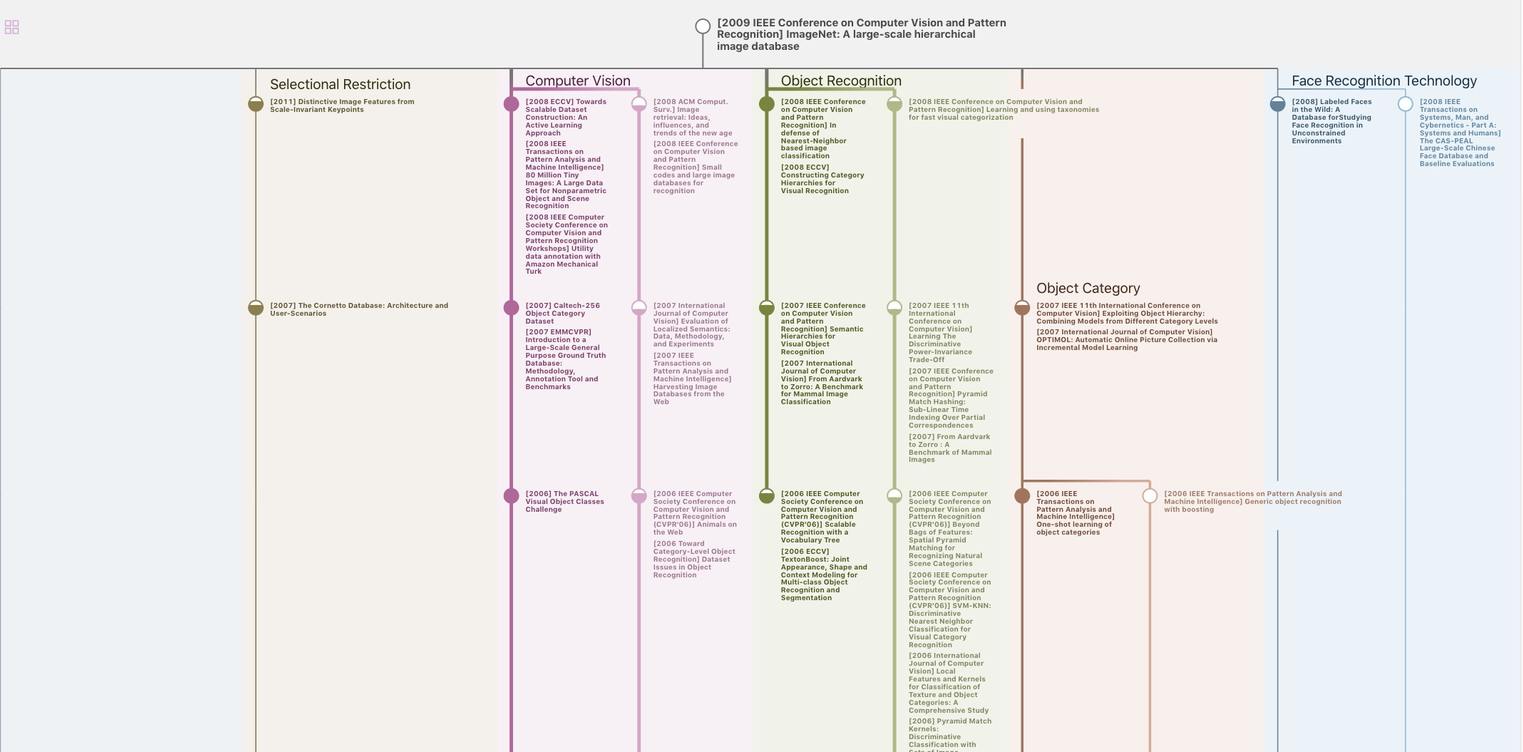
生成溯源树,研究论文发展脉络
Chat Paper
正在生成论文摘要