Analysis and Design of Precision-Scalable Computation Array for Efficient Neural Radiance Field Rendering
IEEE TRANSACTIONS ON CIRCUITS AND SYSTEMS I-REGULAR PAPERS(2023)
摘要
Neural Radiance Field (NeRF), a disruptive method for 3D representation and rendering, is extremely popular in the field of computer graphics and computer vision in the past three years. The most distinctive feature of NeRF models is their scene representation property, making it possible to quantize the models according to the complexity of the representing scenes. This paper proposes a novel approach to improve the efficiency of NeRF rendering by adopting precision-scalable computation. We first analyze and validate the idea of scene-dependent quantization for NeRF models. Based on that, we further propose look-up table (LUT) processing element (PE)-based precision-scalable computation unit designs. To evaluate the performance of different precision-scalable computing units, we implement these designs and compare the corresponding area, power, speed and energy efficiency. We also compare the proposed designs with existing approaches as well as the fixed precision approach for NeRF rendering tasks. The comparison results show that energy efficiency can be significantly improved by using precision-scalable computation for NeRF.
更多查看译文
关键词
Index Terms-Neural radiance field (NeRF), precision-scalable, energy-efficient, neural rendering
AI 理解论文
溯源树
样例
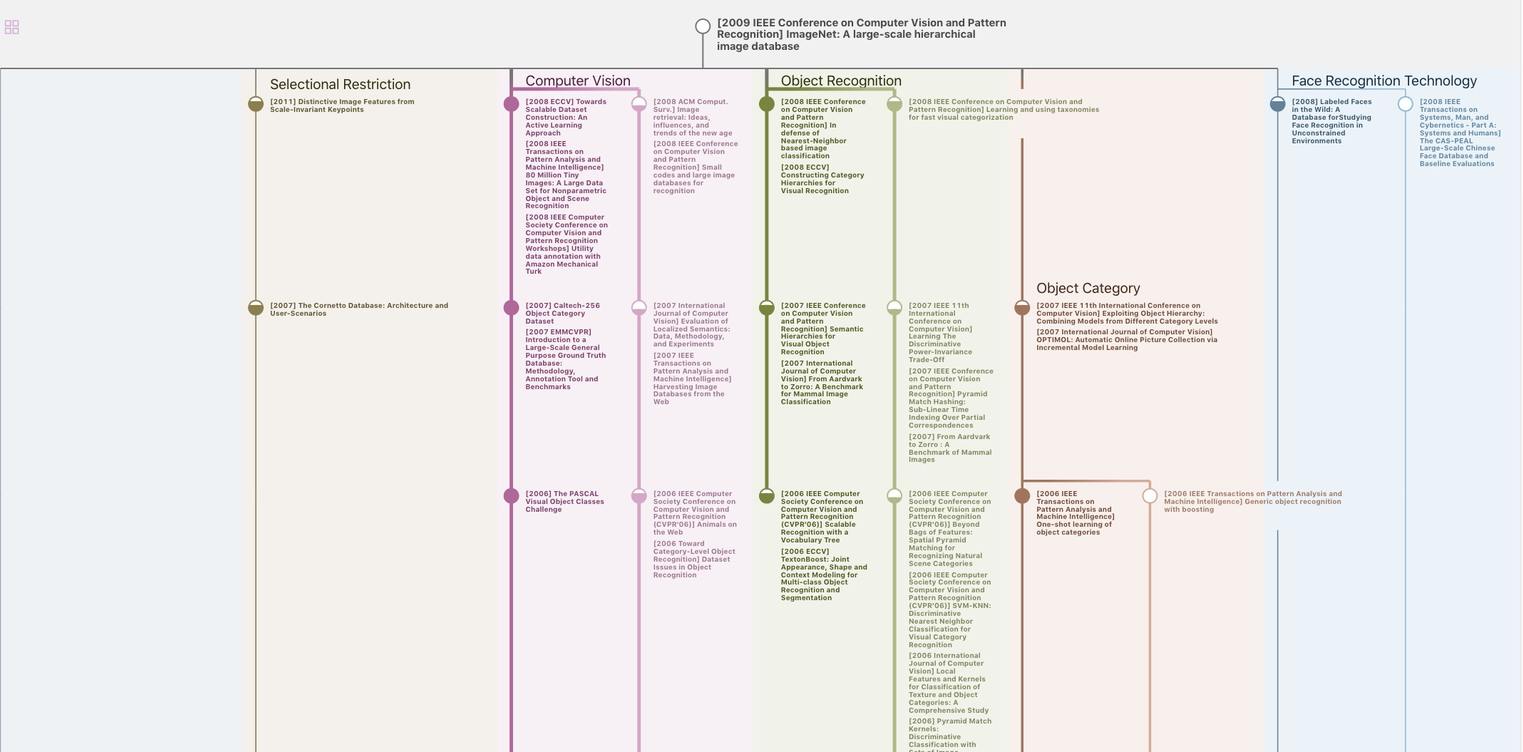
生成溯源树,研究论文发展脉络
Chat Paper
正在生成论文摘要