Air Temperature Monitoring over Low Latitude Rice Planting Areas: Combining Remote Sensing, Model Assimilation, and Machine Learning Techniques
REMOTE SENSING(2023)
摘要
Air temperature (Ta) is essential for studying surface processes and human activities, particularly agricultural cultivation, which is strongly influenced by temperature. Remote sensing techniques that integrate multi-source data can estimate Ta with a high degree of accuracy, overcoming the shortcomings of traditional measurements due to spatial heterogeneity. Based on in situ measurements in Guangdong Province from 2012 to 2018, this study applied three machine learning (ML) models and fused multi-source datasets to evaluate the performance of four data combinations in Ta estimation. Correlations of covariates were compared, focusing on rice planting areas (RA). The results showed that (1) The fusion of multi-source data improved the accuracy of model estimations, where the best performance was achieved by the random forest (RF) model combined with the ERA5 combination, with the highest R-2 reaching 0.956, the MAE value of 0.996 & DEG;C, and the RMSE of 1.365 & DEG;C; (2) total precipitation (TP), wind speed (WD), normalized difference vegetation index (NDVI), and land surface temperature (LST) were significant covariates for long-term Ta estimations; (3) Rice planting improved the model performance in estimating Ta, and model accuracy decreased during the crop rotation in summer. This study provides a reference for the selection of temperature estimation models and covariate datasets. It offers a case for subsequent ML studies on remote sensing of temperatures over agricultural areas and the impact of agricultural cultivation on global warming.
更多查看译文
关键词
remote sensing,model assimilation,temperature,rice
AI 理解论文
溯源树
样例
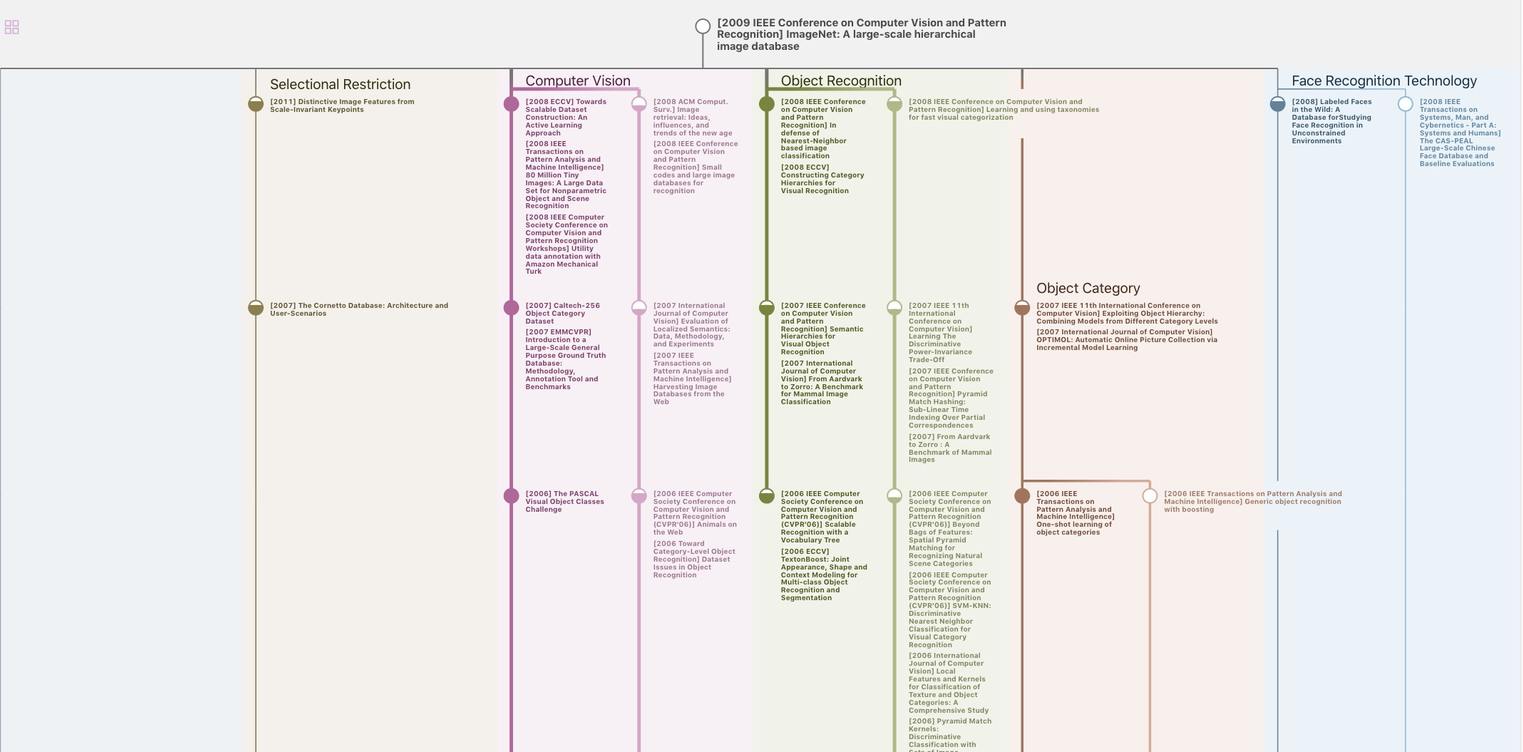
生成溯源树,研究论文发展脉络
Chat Paper
正在生成论文摘要