Prediction of Internal Temperature in Greenhouses Using the Supervised Learning Techniques: Linear and Support Vector Regressions
APPLIED SCIENCES-BASEL(2023)
Abstract
Agricultural greenhouses must accurately predict environmental factors to ensure optimal crop growth and energy management efficiency. However, the existing predictors have limitations when dealing with dynamic, non-linear, and massive temporal data. This study proposes four supervised learning techniques focused on linear regression (LR) and Support Vector Regression (SVR) to predict the internal temperature of a greenhouse. A meteorological station is installed in the greenhouse to collect internal data (temperature, humidity, and dew point) and external data (temperature, humidity, and solar radiation). The data comprises a one year, and is divided into seasons for better analysis and modeling of the internal temperature. The study involves sixteen experiments corresponding to the four models and the four seasons and evaluating the models' performance using R-2, RMSE, MAE, and MAPE metrics, considering an acceptability interval of +/- 2 degrees C. The results show that LR models had difficulty maintaining the acceptability interval, while the SVR models adapted to temperature outliers, presenting the highest forecast accuracy among the proposed algorithms.
MoreTranslated text
Key words
smart agriculture,data science,supervised learning
AI Read Science
Must-Reading Tree
Example
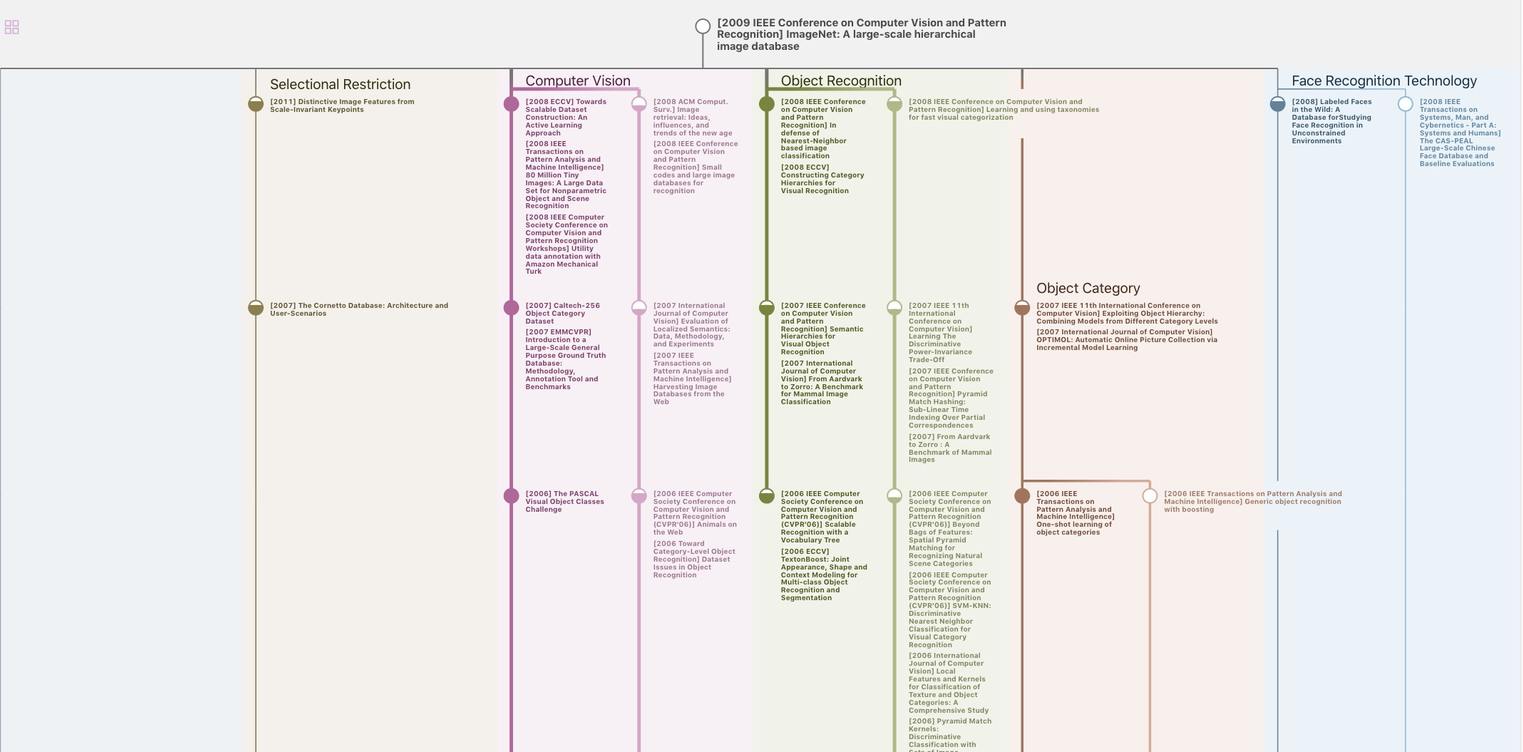
Generate MRT to find the research sequence of this paper
Chat Paper
Summary is being generated by the instructions you defined