CSSRS: Pore segmentation method of sandstone cast thin section images based on weak supervised learning
GEOENERGY SCIENCE AND ENGINEERING(2023)
摘要
Semantic segmentation based on deep learning has been gradually applied to digital rocks, but the amount of training sets and the fineness of data labelling affect the accuracy of the final segmentation, as well as the pixellevel annotation would cost a lot of human effort and be accompanied by certain errors. To address the problem of high data labelling cost of sandstone cast thin section images and segmentation accuracy affected by manual bias, this paper proposes a new method combining Smoothed Score Class Activation Mapping (SSCAM) and Restricted Similarity Dual Contrast Learning (RSimDCL), and we named it CSSRS. Using an image-level annotated dataset, the study applied CSSRS to the images with pore segments (pore images) presented and those without (background images). CSSRS utilized SSCAM to obtain the general location of the pores from the cast thin section map, adopted RSimDCL to obtain the fine boundaries of the pore area, and combined these two methods to generate more accurate pore segmentation of sandstone cast thin section images. Finally, pore segmentation was performed on the same test dataset using CSSRS and RSimDCL, and the segmentation results were compared with various Class Activation Mapping (CAM) algorithms based on weakly supervised learning and various Generative Adversarial Networks (GAN) algorithms. Results showed that CSSRS demonstrated the highest Precision of 79.35% (18.65% higher than the second best method RSimDCL). In addition, the study applied Mean Intersection over Union (MIoU), Dice, Pixel Accuracy (PA), False Positive Rate (FPR) comparison metrics and found that CSSRS consistently demonstrated a better performance of 5%-6% improvement than the second best method in each metric. In conclusion, the CSSRS method based on weakly supervised learning can be applied to pore segmentation of sandstone cast thin section images, which significantly reduces the data annotation workload, avoids the manual bias, and also increases the segmentation effect to a greater extent.
更多查看译文
关键词
Cast thin section,Semantic segmentation,Smoothed score-CAM,Generative adversarial networks (GAN)
AI 理解论文
溯源树
样例
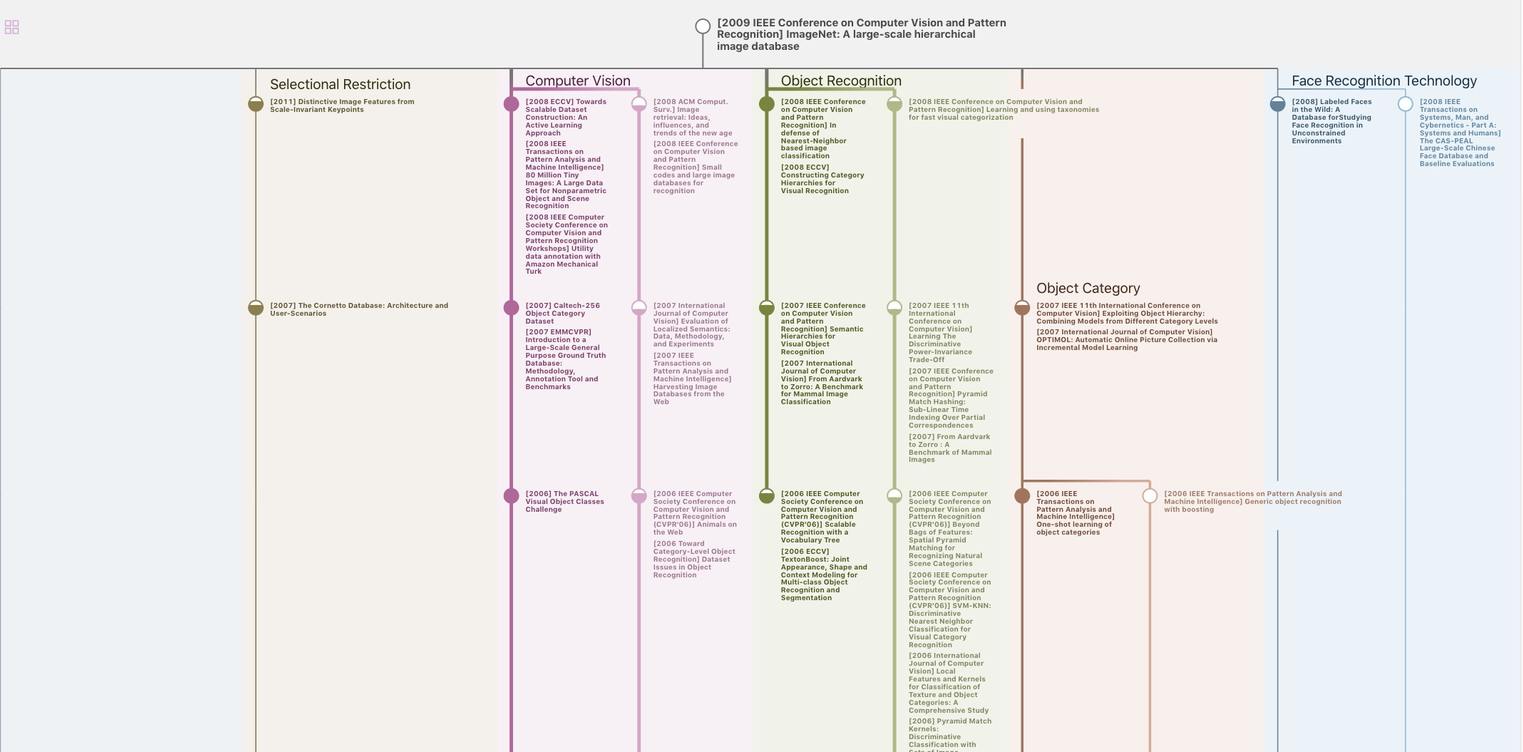
生成溯源树,研究论文发展脉络
Chat Paper
正在生成论文摘要