Driving Style Feature Extraction and Recognition Based on Hyperdimensional Computing and Semi-Supervised Twin Projection Vector Machine
IEEE TRANSACTIONS ON INTELLIGENT TRANSPORTATION SYSTEMS(2023)
Abstract
Driving style recognition is one of the most crucial requirements for human-centric autonomous and assistive driving systems. Existing studies either require a large amount of labeled data or suffer from hand-crafted features, thus hindering the practical application of these methods. To address the above challenges, this paper proposes a driving style recognition approach from the perspective of brain-inspired hyperdimensional computing and semi-supervised learning. Specifically, raw sensor signals are treated as multivariate time series which are encoded by hyperdimensional computing, thus generating a large holistic feature representation. Then, considering the high dimension of the resulting representation, we put forward a semi-supervised twin projection vector machine (SSTPVM) model that can take full advantage of unlabeled data and jointly optimize multiple projection directions tailored for dimension reduction. In addition, a heuristic method based on particle swarm optimization is developed for the parameter selection of SSTPVM. Finally, extensive experiment comparisons with other related methods are performed on naturalistic driving style data. The results show that hyperdimensional computing is rather suitable for semi-supervised learning while our proposed SSTPVM can significantly improve recognition performance even with a small portion of labeled data.
MoreTranslated text
Key words
Index Terms-Driving style recognition, semi-supervised learn-ing, hyperdimensional computing, twin support vector machine
AI Read Science
Must-Reading Tree
Example
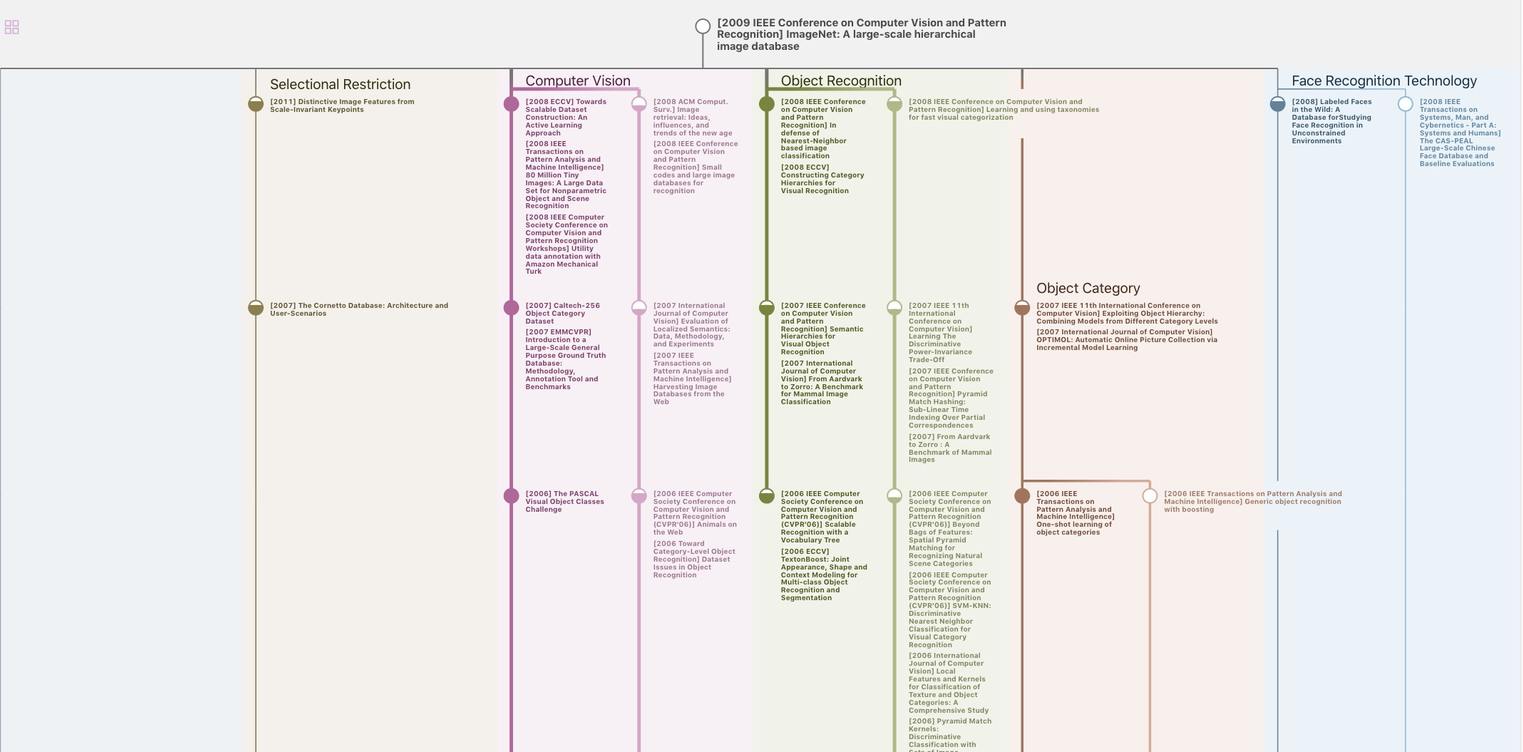
Generate MRT to find the research sequence of this paper
Chat Paper
Summary is being generated by the instructions you defined