A 0.05-mm2 2.91-nJ/Decision Keyword-Spotting (KWS) Chip Featuring an Always-Retention 5T-SRAM in 28-nm CMOS
IEEE JOURNAL OF SOLID-STATE CIRCUITS(2024)
摘要
This article reports a keyword-spotting (KWS) chip for voice-controlled devices. It features a number of techniques to enhance the performance, area, and power efficiencies: 1) a fast-sampling convolutional neural network (FS-CNN) that eliminates the power-hungry feature extractors and reduces the decision latency; 2) an always-retention 5T-SRAM that features word-voltage switches to reduce the leakage power and single bitline (BL) operation to halve the SRAM read power compared to the typical 6T-SRAM; and 3) a high-resolution sparsity-aware computing (HR-SAC) unit that enhances the precision and output swing of the multiply-accumulate (MAC) computation. Benchmarking with the state-of-the-art, our KWS chip prototyped in 28-nm CMOS scores a >90% accuracy for the 11-class Google speech command dataset (GSCD) at 2.91 mu W, which corresponds to a 2.91-nJ energy/decision. The achieved latency is 2 ms/decision, and the core area is 0.05 mm(2), including the full KWS model.
更多查看译文
关键词
5T-SRAM,convolutional neural network (CNN),input stationery,keyword spotting (KWS),low-leakage memory,quantization,switched-capacitor circuits
AI 理解论文
溯源树
样例
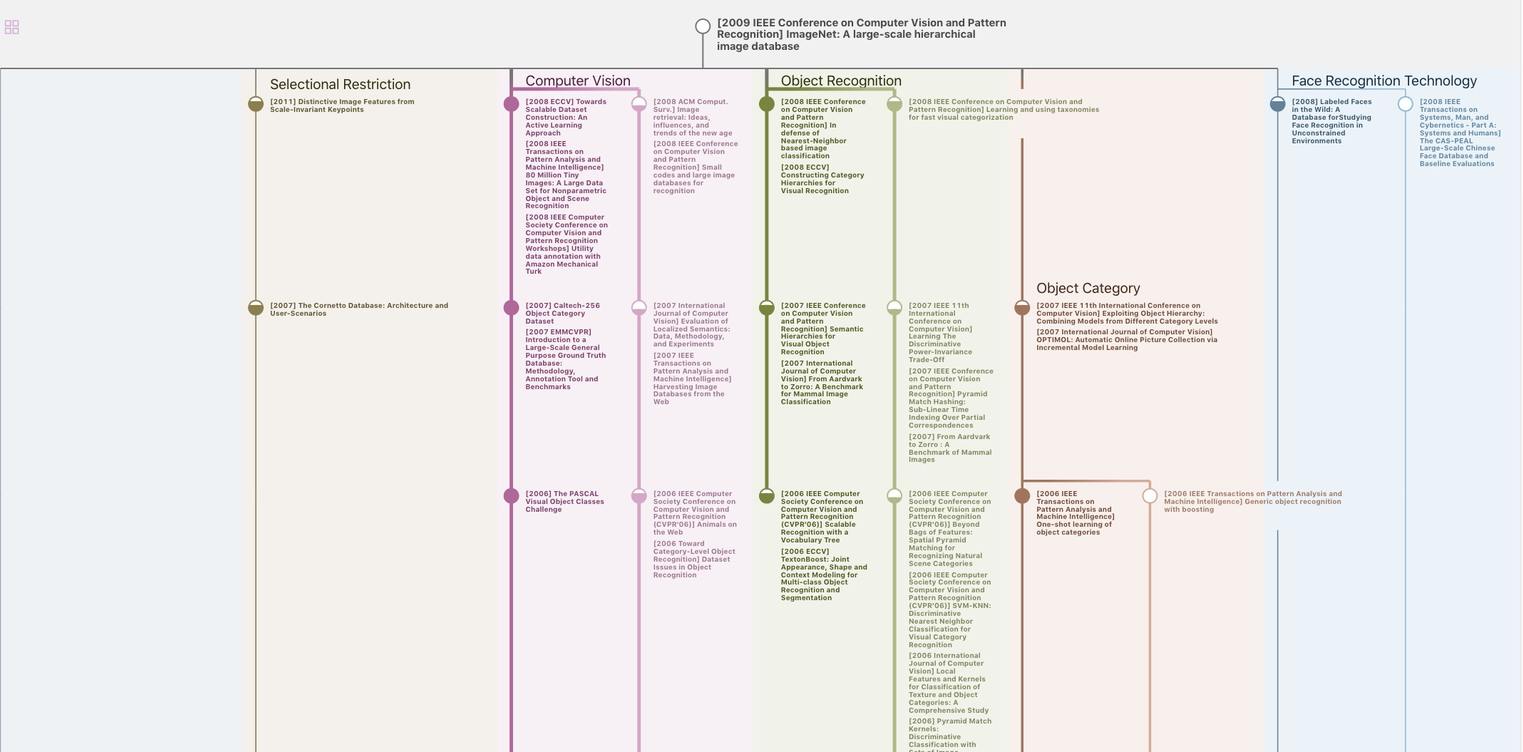
生成溯源树,研究论文发展脉络
Chat Paper
正在生成论文摘要