Retinal Vessel Segmentation via Self-Adaptive Compensation Network
ACTA OPTICA SINICA(2023)
Abstract
Objective Human eye is a crucial component of vision, but the number of patients suffering from ocular illnesses is growing every year. It has been discovered that the morphological characteristics of retinal blood vessels are strongly associated with several ocular conditions including diabetic retinopathy and glaucoma, and they are frequently employed in clinical diagnosis. Therefore, precise segmentation of retinal blood vessels based on color fundus images is crucial for the diagnosis of ocular illnesses. However, the fundus image itself displays noise, poor contrast, and an unbalanced distribution of blood vessels and background pixels. Additionally, morphological information gathering is challenging due to the delicate, highly curved, and multi-scale properties of retinal blood vessels. The time-consuming, difficult, and subjective nature of doctors' manual segmentation makes it ineffective for providing a large number of patients with a speedy diagnosis. To achieve precise automatic segmentation of retinal blood vessels from end to end, we propose the self-adaptive compensation network (SACom). Methods SACom employs the U-shaped network as its fundamental structure. First, deformable convolution is incorporated into the encoder to enhance the model's capacity to learn information about morphological structures of retinal blood vessels. An adaptive multi-scale aligned context (AMAC) module is then developed at the bottom of the U-shaped network to extract and aggregate multi-scale context information and align the context features produced by pooling. It can adaptively extract context features according to the input image size and utilize the image context information correctly. Finally, a collaborative compensation branch (CCB) is proposed to fully leverage the feature layer in the decoder and high-level semantic features at the bottom of the network. Its multi-level outputs are helpful for positioning the overall structure of the blood vessel to fine details. Then they are fused with the output feature layer of the decoder end through the feature layer averaging adaptive fusion to improve the mapping capability of the model. Results and Discussions The segmentation accuracy of retinal vessels can be effectively improved by the proposed SACom model. Each module is beneficial to improve segmentation performance according to the ablation experiment. Compared with the baseline model, SACom just adds a small number of extra parameters (Table 3). The proposed approach can thoroughly detect both thick blood vessels and thin blood vessels, and the connectedness of blood vessels is also more ideal, according to the visualization results of the segmentation (Fig. 6). Subsequent investigation reveals that there are microscopic blood vessels in the SACom segmentation results that are not labeled by experts but exist in fundus images (Fig. 7). It is clear that SACom has a good ability to segment blood vessels and identify blood vessel pixels more accurately, thereby addressing strong subjectivity in manual labeling. SACom performs better than other state-of-the-art methods generally (Table 5), with high sensitivity. The accuracy reaches 0. 9695, 0. 9763, and 0. 9753, the sensitivities are 0. 8403, 0. 8748, and 0. 8506, and the respective AUC values are 0. 9880, 0. 9917, and 0. 9919 for DRIVE, CHASE_DB1, and STARE datasets, respectively. Conclusions An effective automatic segmentation algorithm called SACom is put forth to achieve precise segmentation of retinal vessels in fundus images. SACom integrates deformable convolution into the encoder based on the network architecture of U-Net to improve the learning capacity of vascular structural information. The bottom of the U-Net is constructed with an AMAC module that can collect and aggregate multi-scale aligned context information to adapt to the multi-scale issue of retinal blood vessels. Finally, a CCB is proposed. Its multi-level outputs calculate loss respectively and conduct backpropagation to improve the accuracy of each branch's output result. The outputs of the CCB are averaged and then adaptively fused with the output feature map of the decoder for accurate segmentation. The experimental results on three datasets reveal that the method has excellent generalization capability for different pixel classifications, especially for blood vessel pixels, and its comprehensive segmentation performance is better than other state-of-the-art algorithms. In addition, the proposed algorithm does not need too much computation load, which makes it easy to deploy in clinical applications.
MoreTranslated text
Key words
image processing,retinal vessels,deformable convolution,context alignment,feature adaptive fusion
AI Read Science
Must-Reading Tree
Example
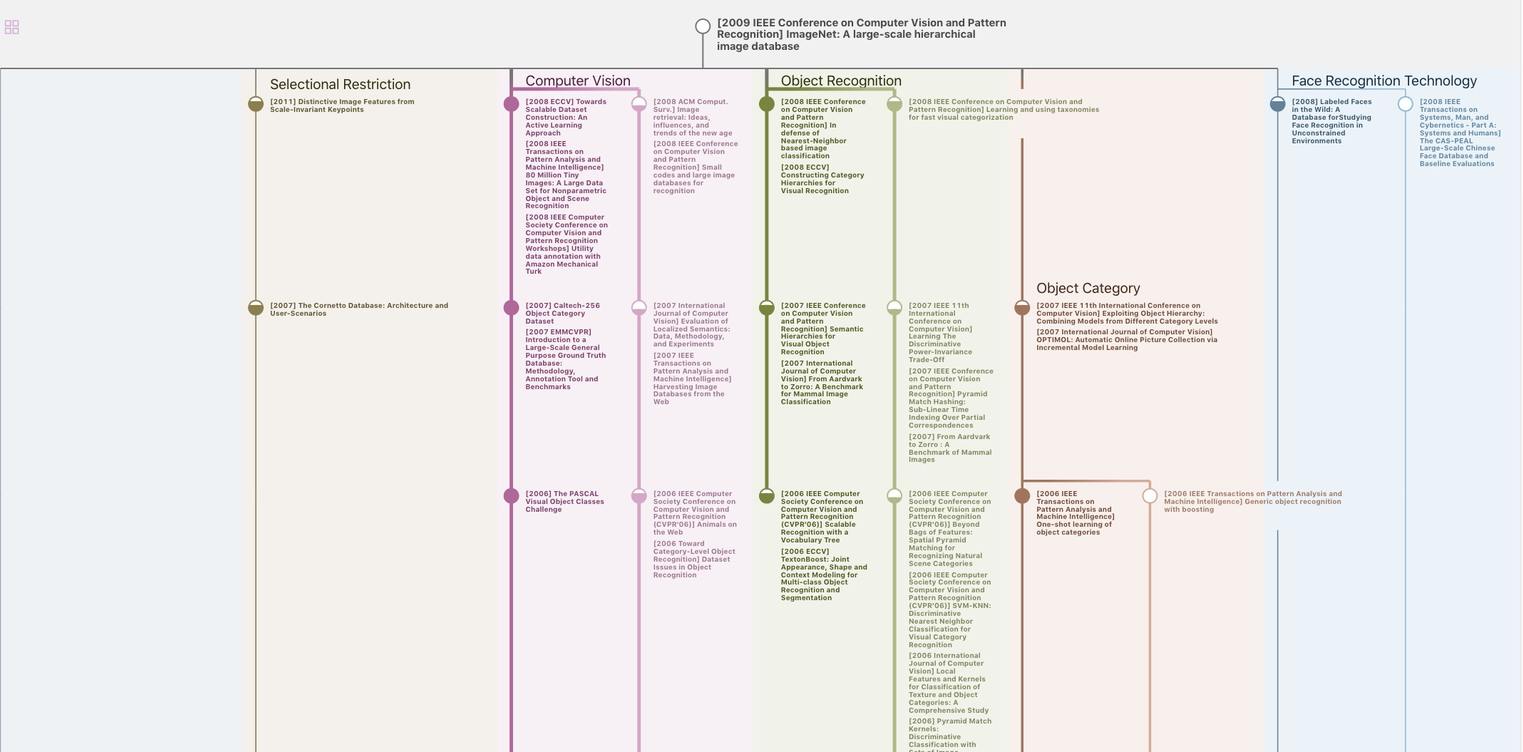
Generate MRT to find the research sequence of this paper
Chat Paper
Summary is being generated by the instructions you defined