On Generating Synthetic Histopathology Images Using Generative Adversarial Networks
2023 34TH IRISH SIGNALS AND SYSTEMS CONFERENCE, ISSC(2023)
摘要
Interest has been growing over the past number of years in the application of Artificial Intelligence (AI) to aid in cancer diagnosis. However, the requirement for extensive training data to train these AI models presents a significant challenge. This paper proposes a viable solution to this issue by synthesising images to augment the real data in the training dataset. We investigate the potential of Generative Adversarial Networks (GANs) in generating high-quality tissue images for both colon and prostate. The datasets we use are from the TCGA repository and contain various cancerous diseases for both colon and prostate. We outline how the data was pre-processed, and quality controlled and we also delineate the relevant experiments applied. We apply some of the most recent GAN architectures in ProGAN and StyleGAN to generate synthetic colon tissue images, achieving a literature-high Fr ' echet Inception Score (FID) score of 6.019 for colon tissue images and an FID score of 3.03 for prostate tissue images. Notably, the synthetic images produced were deemed indistinguishable from real samples when assessed by a practicing consultant histopathologist. By addressing the challenge of limited training data, our approach could ultimately contribute to earlier detection and improved treatment outcomes for cancer patients worldwide.
更多查看译文
关键词
Artificial Intelligence,Generative Adversarial Networks,Progressive GAN,StyleGAN,Digital Pathology,Histopathology
AI 理解论文
溯源树
样例
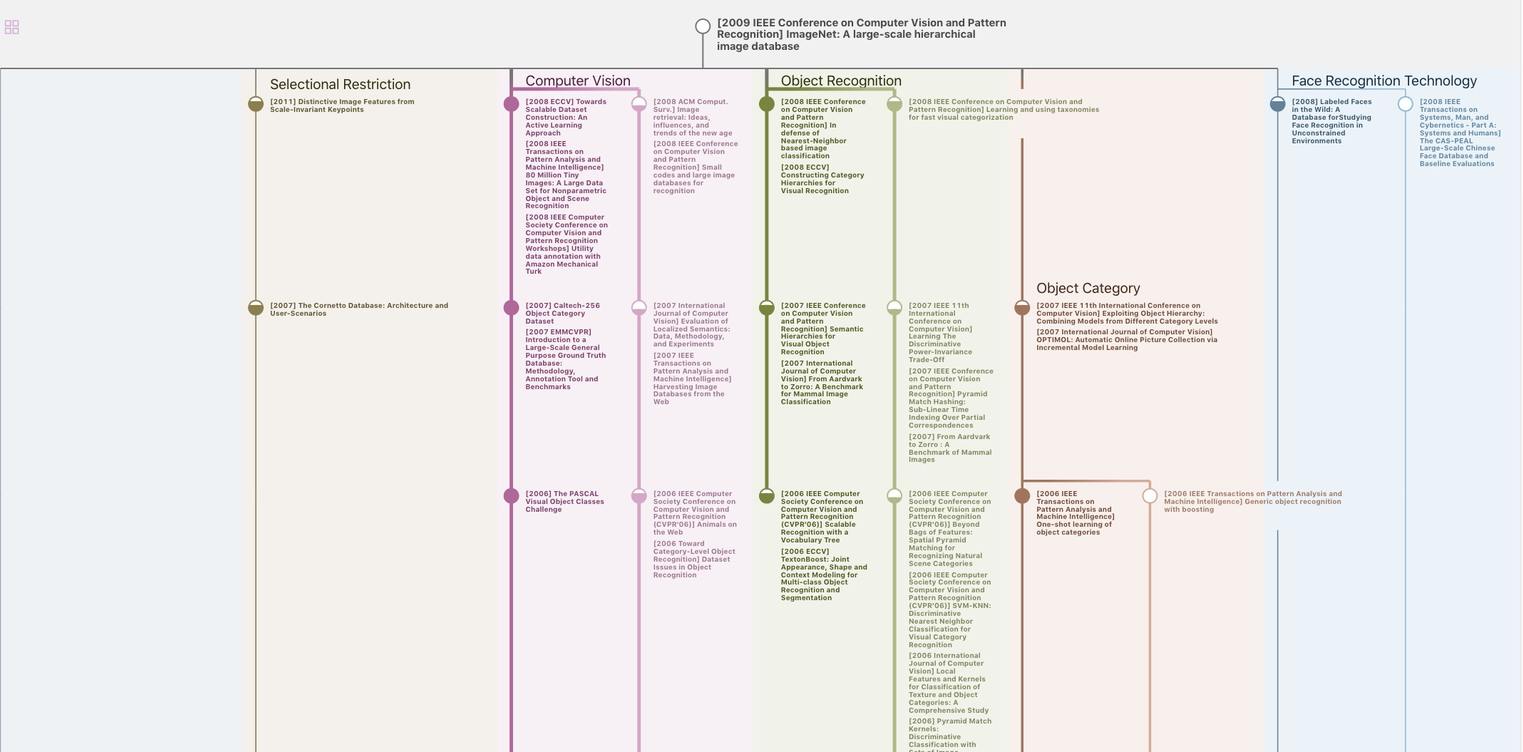
生成溯源树,研究论文发展脉络
Chat Paper
正在生成论文摘要