Differential evolution algorithm supported random forest classifier for effective feature selection and classification of power quality disturbances
UNIVERSITY POLITEHNICA OF BUCHAREST SCIENTIFIC BULLETIN SERIES C-ELECTRICAL ENGINEERING AND COMPUTER SCIENCE(2023)
摘要
The rise in the usage of power electronic equipment, which induces a deviation in the voltage and current waveforms, has raised the relevance of power quality (PQ) issues. It is important to classify them accurately in order to address the PQ problems. One of the most effective methods to classify PQ problems is to combine Machine Learning (ML) algorithms with efficient feature extraction and feature selection techniques. In the present work, the S-transform-based feature extraction technique is adopted and extracts around 20 features from the PQ disturbance signal. And then, Differential Evolution (DE) based feature selection technique is utilized for feature selection and five optimized features are selected and given as input to the ML classifiers. It is a method that is essential in data mining in order to reduce dimensionality and enhance the performance of ML classifiers. Eight different ML classifiers namely the K-Means, Adaptive Boosting (AdaBoost), K- nearest neighbor (KNN), Naive Bayes, Recursive Support Vector Machine (R-SVM), Linear SVM, Decision Tree, and Random Forest (RF) algorithms have been employed in this work. The effectiveness of the ML Classifiers is measured in terms of various performance evaluation measures namely precision, accuracy, F1-score, recall, and specificity. The results revealed that the DE-supported RF classifier is more efficient when compared with other classifiers in classifying the PQ disturbances even in environments with noise inference.
更多查看译文
关键词
Power Quality Disturbances,S-Transform,Feature Selection,Differential Evolution,Random Forest
AI 理解论文
溯源树
样例
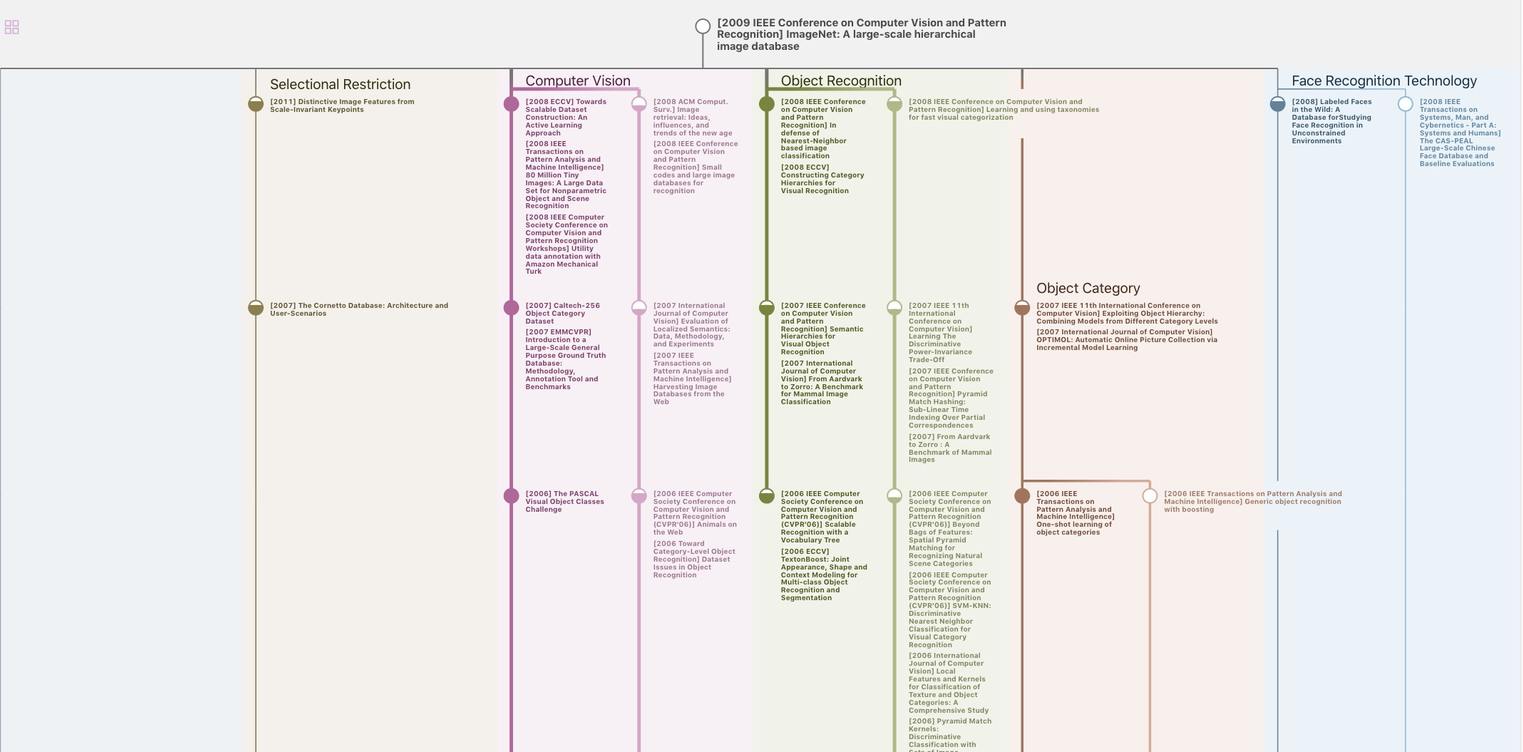
生成溯源树,研究论文发展脉络
Chat Paper
正在生成论文摘要