Multitemporal image cloud removal using group sparsity and nonconvex low-rank approximation
JOURNAL OF NONLINEAR AND VARIATIONAL ANALYSIS(2023)
摘要
Remote sensing (RS) images are widely used in environmental monitoring, urban planning, and land surface classification. However, RS images are often polluted by cloud, which leads to the loss of some important information of RS images and hinders the development of relevant applications. The existing spatial-spectral total-variational regularization can only promote the spatial-spectral continuity of cloud component, but cannot maintain the shared group sparse mode of spatial difference images of different spectral bands. To solve this problem, we add the weighted & POUND;2,1-norm to constrain the cloud component differential images, and use the nonconvex regularization term, namely the weighted nuclear norm, to replace the traditional nuclear norm, which solves the problem that the original nuclear norm violates the larger singular value. In summary, we propose a multitemporal image cloud removal model based on the weighted nuclear norm which is the nonconvex low-rank approximation and the group sparsity regularization (WNGS), where the group sparsity regularization and the weighted nuclear norm promote each other. The resulting problems are solved using the alternating direction method of multipliers. Numerical experiments both simulated and real multitemporal images demonstrate that the proposed method is superior to other advanced cloud-removal methods in different cloud-removal scenarios.
更多查看译文
关键词
Multitemporal image cloud removel,Group sparsity regularization,Weighted nuclear norm
AI 理解论文
溯源树
样例
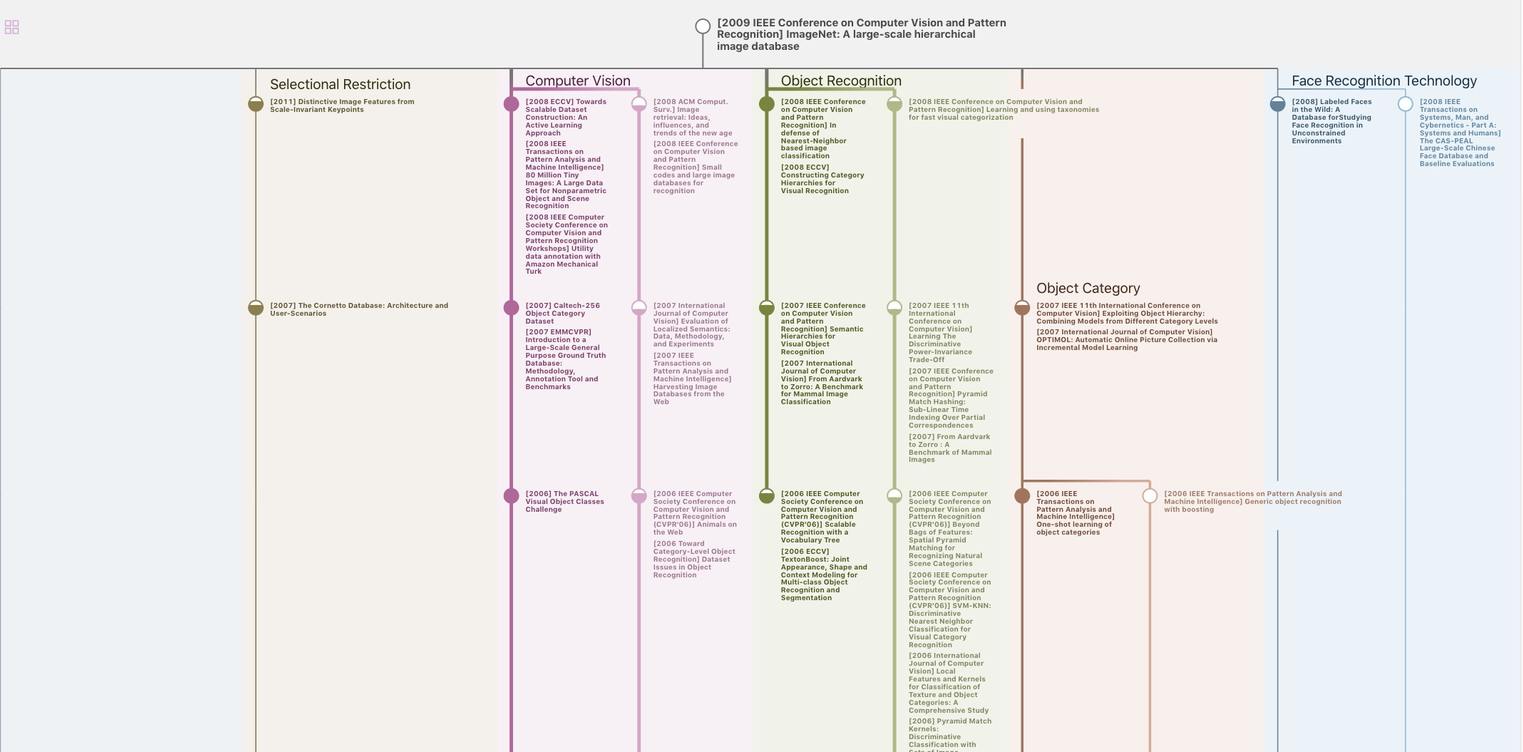
生成溯源树,研究论文发展脉络
Chat Paper
正在生成论文摘要