A Deep Learning Method for Rough Surface Clutter Reduction in GPR Image
2023 IEEE RADAR CONFERENCE, RADARCONF23(2023)
摘要
The major clutter in a typical ground penetrating radar (GPR) B-scan image is the ground surface clutter. It can severely obscure or distort the subsurface target response, especially when the surface profile variation is large and the target reflection signal is overlapped with the surface clutter. In this paper, we propose a deep auto-encoder based method for reducing the rough surface clutter in GPR images. In our method, the rough surface clutter reduction is formulated as an anomaly detection problem. The rough surface region in a Bscan image is splitted into small patches which are used as the training data set to train a deep auto-encoder. The trained auto-encoder captures the patterns of the rough surface patches. After training, the whole B-scan image is divided into small patches of the same size as the training patches and each of which is fed into the auto-encoder to compute an anomaly score. To reduce the clutter, we design a novel method that adopts a weighted sum approach to aggregate all patches based on their anomaly scores. For performance evaluation, simulation and laboratory tests are conducted, and the clutter-to-rest ratios (CRR) are computed to quantify the clutter reduction effectiveness. The CRR of the simulated B-scan image is reduced from 4.24 dB to -14.06 dB, and the CRRs of two measured B-scans are reduced from 3.71 dB to -20.45 dB, and from 8.62 dB to -8.07 dB, respectively.
更多查看译文
关键词
deep auto-encoder,rough surface clutter reduction,ground penetrating radar
AI 理解论文
溯源树
样例
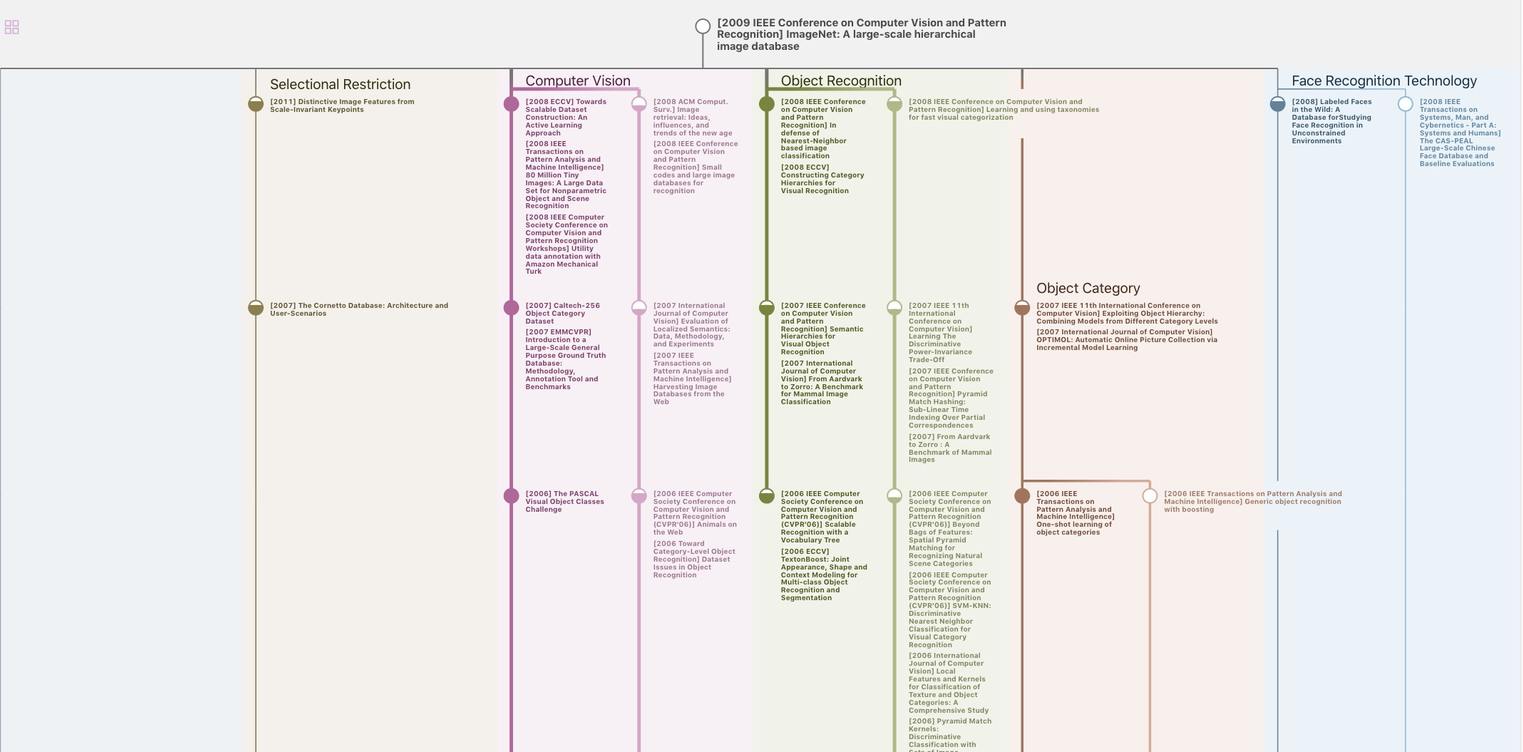
生成溯源树,研究论文发展脉络
Chat Paper
正在生成论文摘要