Light-Weight Learning Model with Patch Embeddings for Radar-based Fall Event Classification: A Multi-domain Decision Fusion Approach
2023 IEEE RADAR CONFERENCE, RADARCONF23(2023)
摘要
With the increasing morbidity and mortality rate in older adults above 65 years of age due to accidental fall, privacy-preserving radar-based fall event detection is becoming crucial. Deep learning algorithm like vision transformers (ViT) for human fall-event detection using different radar domain representation have shown excellent fall-detection accuracy. However, such techniques are computationally very expensive and unsuitable when training datasets are small. Patch-based learning models such as Multi-Layer Perceptron-Mixer (MLP-Mixer) and Convolutional-Mixer (ConvMixer) models have been developed as alternatives to ViT. In this work, the decision outputs of lightweight ConvMixer models with different domain representations of radar returns as inputs are fused for classifying the events as fall or non-fall. This proposed approach of event classification utilizes supplementary information present in different domains for enhancing the classification accuracy. Evaluation done on publicly available dataset shows an improved performance of the multi-domain ConvMixer model over ViT and MLP-Mixer. This further justifies the choice of light weight ConvMixer as a preferred learnable model when only limited training dataset is available.
更多查看译文
关键词
Radar, Fall detection, Patch-based learning, Dempster Shafer fusion, Multi-domain, Vision transformer, Multi-layer Perceptron, ConvMixer
AI 理解论文
溯源树
样例
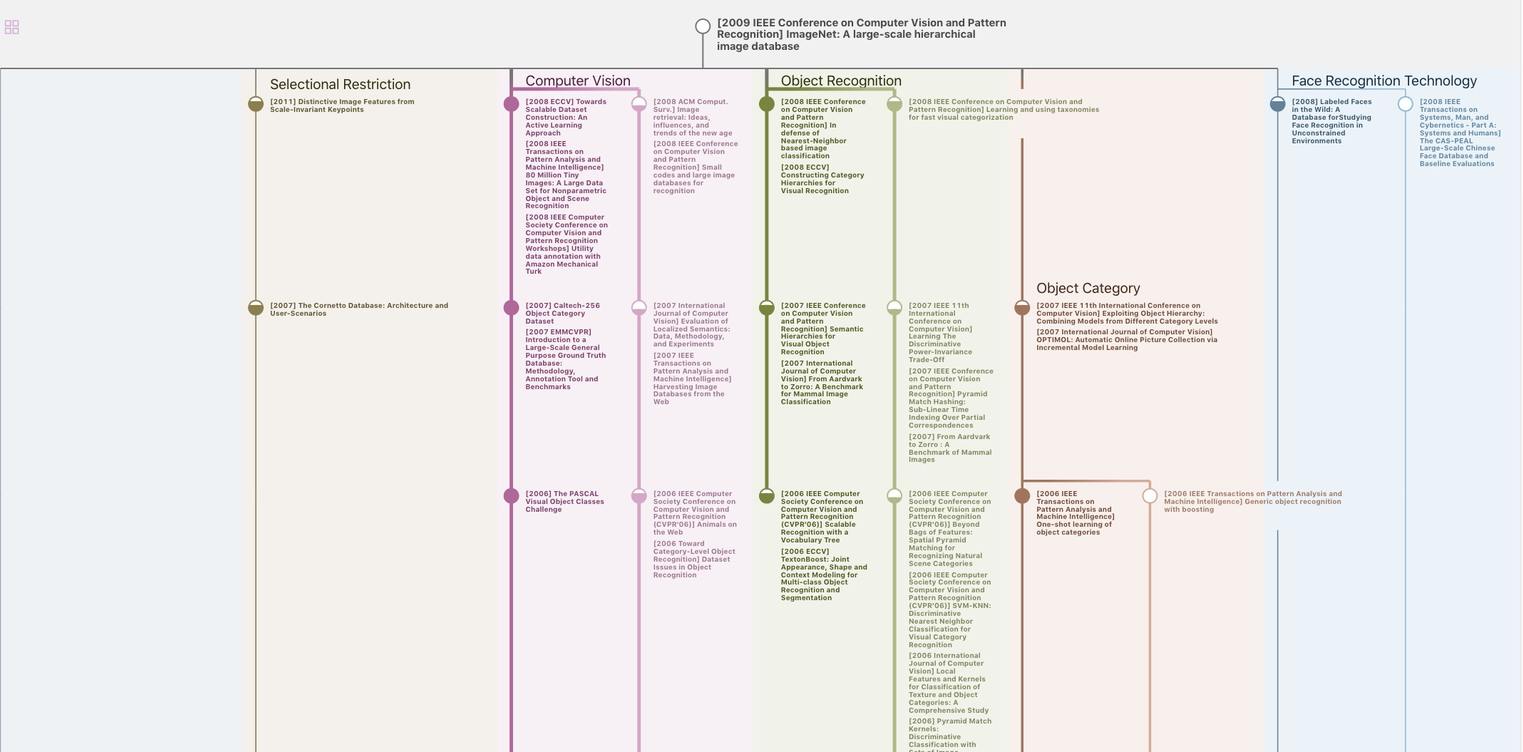
生成溯源树,研究论文发展脉络
Chat Paper
正在生成论文摘要