An attention-based neural network model for automatic partition of abdominal lymph nodes in CT imaging
QUANTITATIVE IMAGING IN MEDICINE AND SURGERY(2023)
摘要
Background: Abdominal lymph node partition is highly relevant to colorectal cancer (CRC) metastasis, which may further affect patient prognosis and survival quality. In the traditional diagnostic process, medical radiologists must partition all lymph nodes from the computed tomography (CT) images for further diagnostics. The manual interpretation of abdominal nodes is experience-dependent and time-consuming, especially for node partition. Therefore, automated partition methods are desirable to make the diagnostic process more accessible. Automatic abdominal lymph node partition is a challenging task due to the subtle morphological features of the nodes and the complex relative position information of the abdominal structure. Methods: In this paper, a node-oriented dataset containing 6,880 nodes with partition labels was constructed by seasoned professionals through 2-round annotation due to there being no dataset with node-oriented labels to perform the partition task. In addition, specific masking strategies and attention mechanisms were proposed for the primary deep neural networks (DNNs). The specific masking strategy could utilize the positional and morphological information more substantially, which intensively exploits prior knowledge and hones the relative positional information in the lower abdomen. The comprehensive attention mechanism could introduce direction-aware information to enhance the inter-channel relationship of features and capture rich contextual relationships with multi-scale kernels. Results: The experiments were based on the node- oriented dataset. The proposed method achieved superior performance [accuracy (ACC): 89.74%; F1 score ( F1): 85.95%; area under the curve (AUC): 88.23%], which is significantly higher than the baseline model with several masking strategies (ACC: 62.05-86.16%; F1: 51.77-80.86%; AUC: 60.44-83.94%). For exploration of attention, the proposed method also outperformed the state-of-the-art convolutional block attention module (CBAM; ACC: 88.90%; F1: 84.09%; AUC: 86.86%) with the same proposed input form. Conclusions: Experimental results indicate that the innovative method performs better in experimental metrics than other prevalent methods. The proposed method is expected to be introduced in future medical scenarios, which will help doctors to optimize the diagnosis workflow and improve partition sensitivity.
更多查看译文
关键词
Abdominal lymph node partition,colorectal cancer (CRC) metastasis,deep neural network (DNN),attention mechanism,masking strategy
AI 理解论文
溯源树
样例
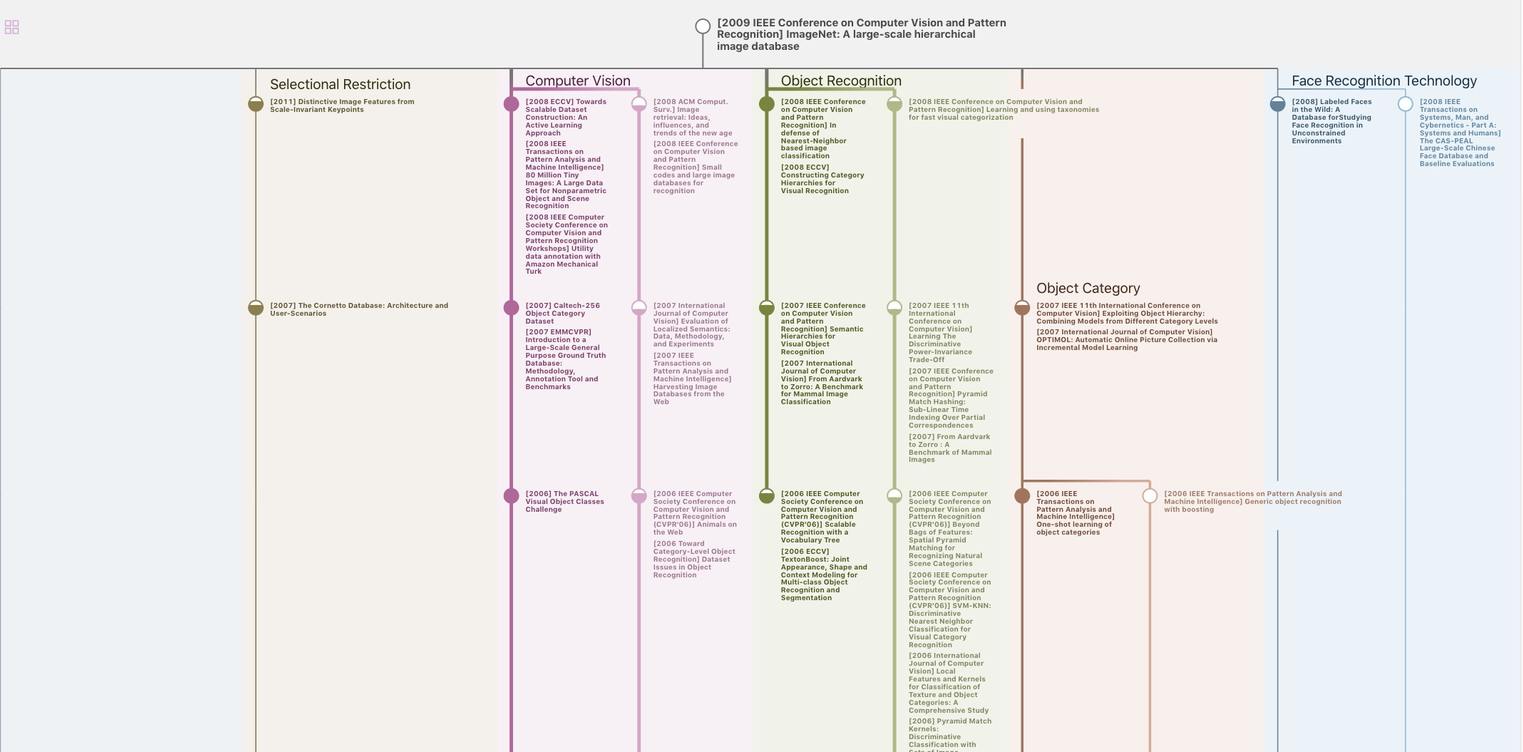
生成溯源树,研究论文发展脉络
Chat Paper
正在生成论文摘要