CFM: a convolutional neural network for first-motion polarity classification of seismic records in volcanic and tectonic areas
FRONTIERS IN EARTH SCIENCE(2023)
摘要
First-motion polarity determination is essential for deriving volcanic and tectonic earthquakes' focal mechanisms, which provide crucial information about fault structures and stress fields. Manual procedures for polarity determination are time-consuming and prone to human error, leading to inaccurate results. Automated algorithms can overcome these limitations, but accurately identifying first-motion polarity is challenging. In this study, we present the Convolutional First Motion (CFM) neural network, a label-noise robust strategy based on a Convolutional Neural Network, to automatically identify first-motion polarities of seismic records. CFM is trained on a large dataset of more than 140,000 waveforms and achieves a high accuracy of 97.4% and 96.3% on two independent test sets. We also demonstrate CFM's ability to correct mislabeled waveforms in 92% of cases, even when they belong to the training set. Our findings highlight the effectiveness of deep learning approaches for first-motion polarity determination and suggest the potential for combining CFM with other deep learning techniques in volcano seismology.
更多查看译文
关键词
deep convolutional neural networks,automatic classification,machine learning,self-organizing maps,volcanic and tectonic earthquakes,first-motion polarity
AI 理解论文
溯源树
样例
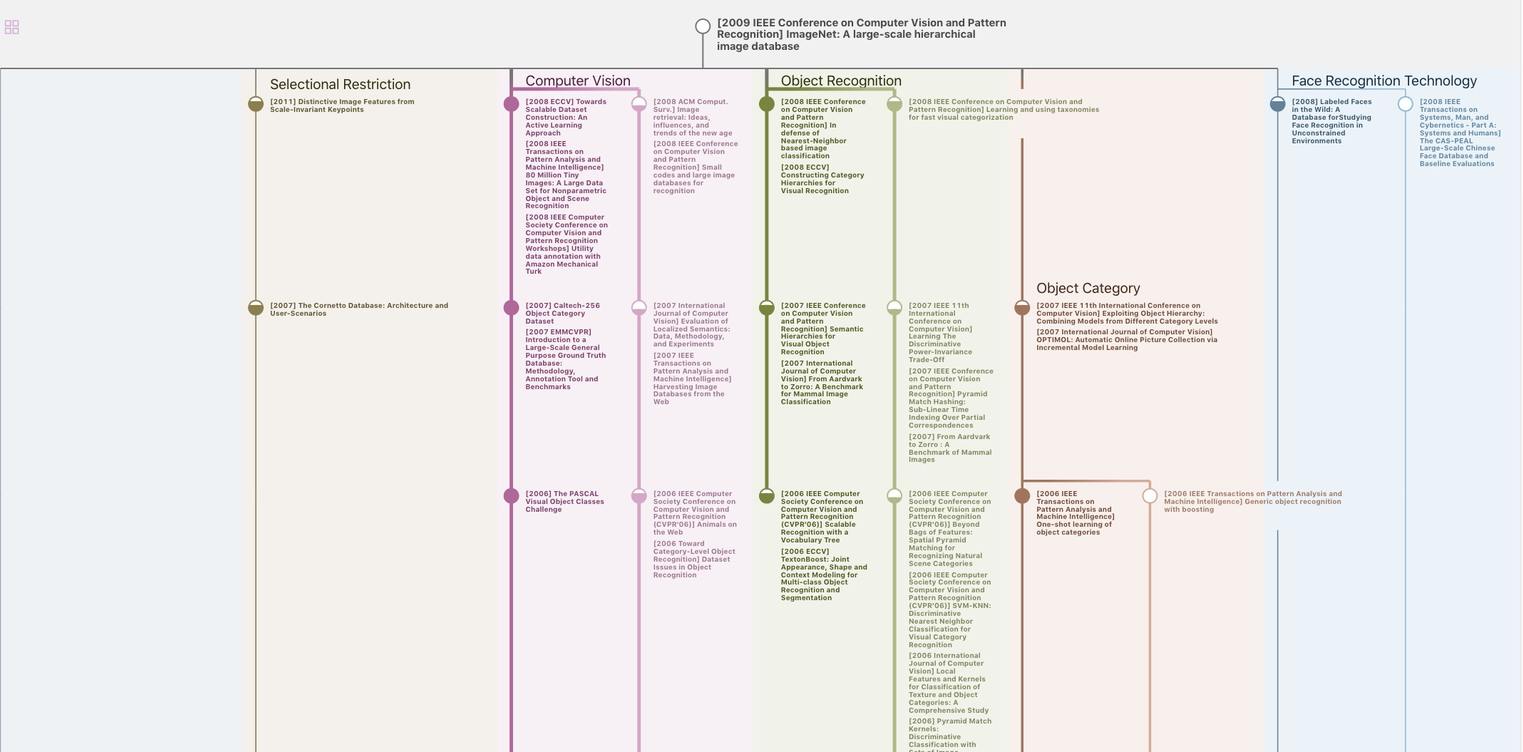
生成溯源树,研究论文发展脉络
Chat Paper
正在生成论文摘要