Unsupervised Time Series Anomaly Detection Based on Adversarial Interpolation and Pseudo-anomaly Calibration
2023 9TH INTERNATIONAL CONFERENCE ON CONTROL, AUTOMATION AND ROBOTICS, ICCAR(2023)
摘要
Existing work on unsupervised time series anomaly detection relies on clean training datasets (free of anomalies), which is often violated in practice. In this work, we propose an unsupervised time series anomaly detection method based on adversarial interpolation and pseudo-anomaly calibration for training an anomaly detector under data contamination. Specifically, the normality description learned from the model is improved by using the following two proposed calibration methods: (1) by enforcing adversarial interpolation to learn an effective normality description from the typical samples rather than the edge or abnormal samples, and (2) by discriminating the original samples from the simulated pseudo-anomaly samples to learn the exact normality description boundary. These two calibration ways result in the normality description that prevents data contamination. Experimental results compared with baselines on public time series datasets demonstrate the effectiveness of our proposed method.
更多查看译文
关键词
Time series anomaly detection, Data contamination, Adversarial interpolation, Pseudo-anomaly
AI 理解论文
溯源树
样例
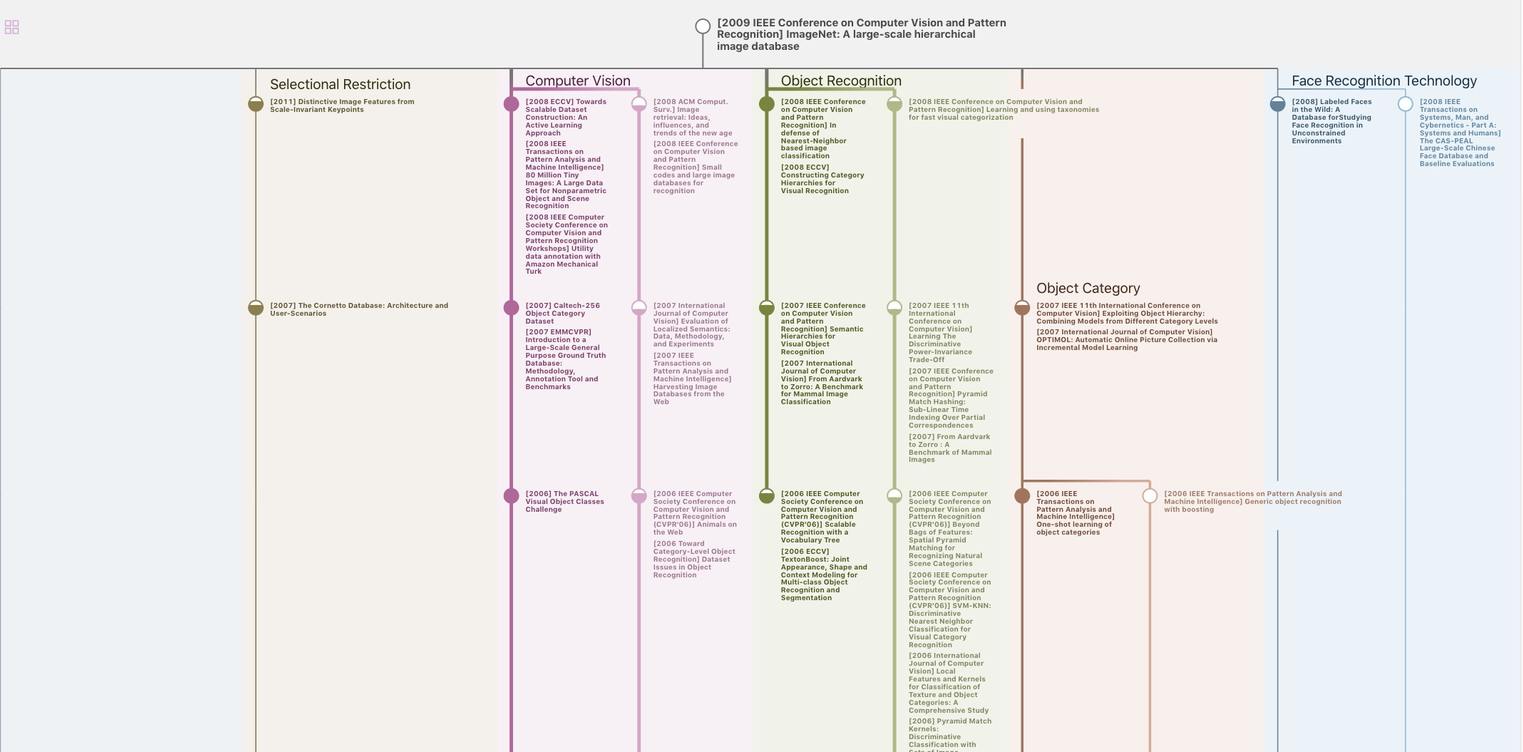
生成溯源树,研究论文发展脉络
Chat Paper
正在生成论文摘要