Classification of Traffic Signaling Motion in Automotive Applications Using FMCW Radar
2023 IEEE RADAR CONFERENCE, RADARCONF23(2023)
摘要
Advanced driver-assisted system (ADAS) typically includes sensors such as Radar, Lidar, or Camera to make vehicles aware of their surroundings. These ADAS systems are presented to a wide variety of situations in traffic, such as upcoming collisions, lane changes, intersections, sudden changes in speed, and other common instances of driving errors. One of the key barriers to automotive autonomy is the inability of self-driving cars to navigate unstructured environments, which typically do not have any traffic lights present or operational for directing traffic. In these circumstances, it is much more common for a person to be tasked with directing vehicles, either by signaling with an appropriate sign or via gesturing. The task of interpreting human body language and gestures by autonomous vehicles in traffic directing scenarios is a great challenge. In this study, we present a new dataset collected of traffic signaling motions using millimeter-wave (mmWave) radar, camera, Lidar and motion-capture system. The dataset is based on those utilized in the US traffic system. Initial classification results from Radar microDoppler (mu-D) signature analysis using basic Convolutional Neural Networks (CNN) demonstrates that deep learning can very accurately (around 92%) classify traffic signaling motions in automotive applications.
更多查看译文
关键词
Micro-Doppler, autonomy, traffic gesture classification, mmWave, ADAS, CNN
AI 理解论文
溯源树
样例
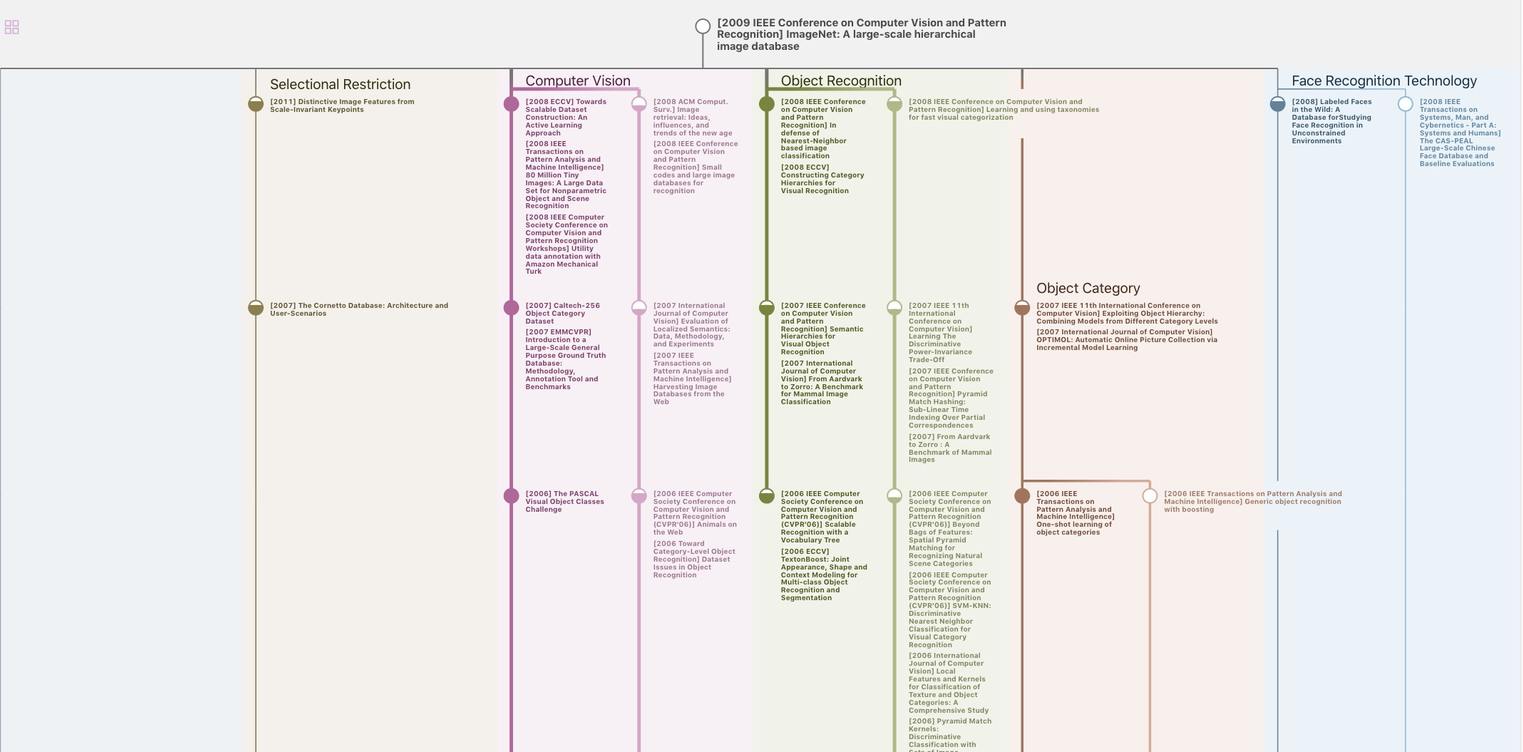
生成溯源树,研究论文发展脉络
Chat Paper
正在生成论文摘要