Image Restoration under Cauchy Noise: A Group Sparse Representation and Multidirectional Total Generalized Variation Approach
TRAITEMENT DU SIGNAL(2023)
摘要
Owing to the ill-posed problem of image restoration, how to find an effective method to obtain image prior information is still challenging. The total generalized variational model has been successfully applied to image denoising and/or deblurring. However, the high -order gradient of the image is described by using L1 norm in the traditional total generalized variational denoising and deblurring model, which can not effectively describe the local group sparse priors of the image gradient. As a result, the traditional total generalized variational model has some limitations in the ability to suppress the staircase artifacts. In order to solve this problem, one new model is proposed to restore images corrupted by Cauchy noise and/or blur in our paper, where the non-convex data fidelity term is combined with two regularization terms: group sparse representation prior and multi-directional total generalized variation. We use group sparse representation prior information to obtain the nonlocal self-similarity information of similar image block for preserving the details and texture features of the discontinuous or uneven region of the image. At the same time, the noise is fully removed in the uniform region, which improves the image visual quality. Moreover, the gradient information in multiple directions is calculated by the multi-directional total generalized variation regularization term, which can better preserve the edge information of the image. The model is divided into several sub-problems by split Bregman iteration, and each sub-problem is solved efficiently. The experimental results show that this model is superior to other existing models both in terms of visual quality and some image quality evaluation.
更多查看译文
关键词
image restoration,sparse representation,multidirectional total generalized variation,Cauchy noise group,dictionary learning
AI 理解论文
溯源树
样例
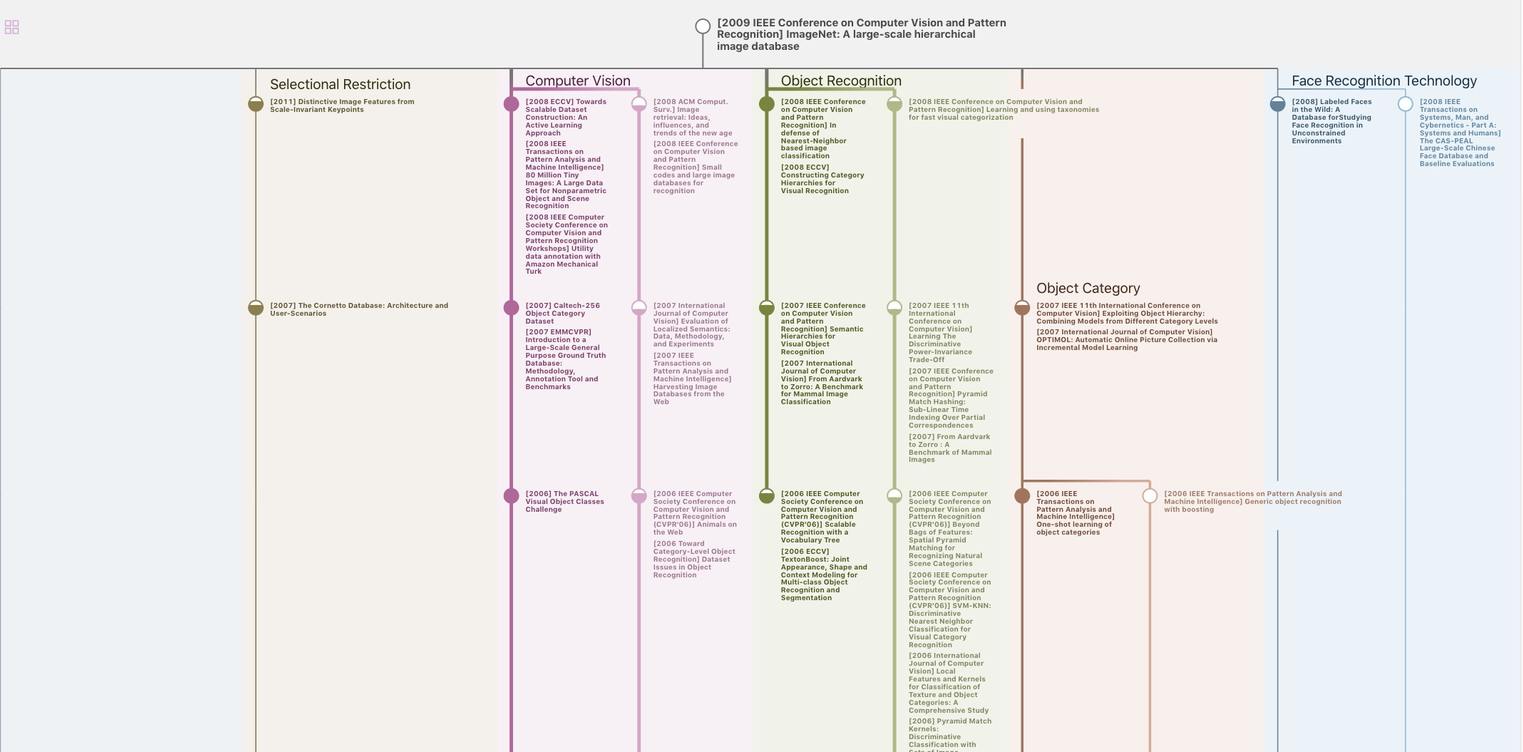
生成溯源树,研究论文发展脉络
Chat Paper
正在生成论文摘要