Edge Detection-Guided Balanced Sampling
NEURAL PROCESSING LETTERS(2023)
摘要
In anchor-based object detection algorithms, achieving a balance between positive and negative samples during training is crucial. Many improved sampling methods have been proposed to address this issue. In our work, we propose an edge-detection guided balanced sampling (EBS) method that introduces edge and contour information from objects to guide the sampling of candidate negative samples and increase the number of hard negative samples to improve sample balance. The EBS method employs a Gaussian filter convolution on the binary image in HSV space to reduce local corner information, and furtherly applies K-means++ clustering to the bounding boxes to reduce redundancy. In the hard negative sample sampling stage of anchor-based detection algorithms, the EBS model selects negative samples with higher IoU values with GT from both the normal candidate negative sample set and the special candidate negative sample set. The latter set contains more hard negative samples, which further improves the sample balance. We conducted comparison experiments using random sampling (RS) and IoU-balanced sampling (IBS) in the RPN network on MSCOCO-2017. Our EBS method achieved a 1.7-point higher higher AR_1000 than PRN. In particular, the proposed algorithm performs better on the small-target dataset, which achieves 2.4-point higher AR_S than RPN. The inference fps of RPN and EBS are 22.3 and 22.4, respectively.
更多查看译文
关键词
balanced sampling,edge,detection-guided
AI 理解论文
溯源树
样例
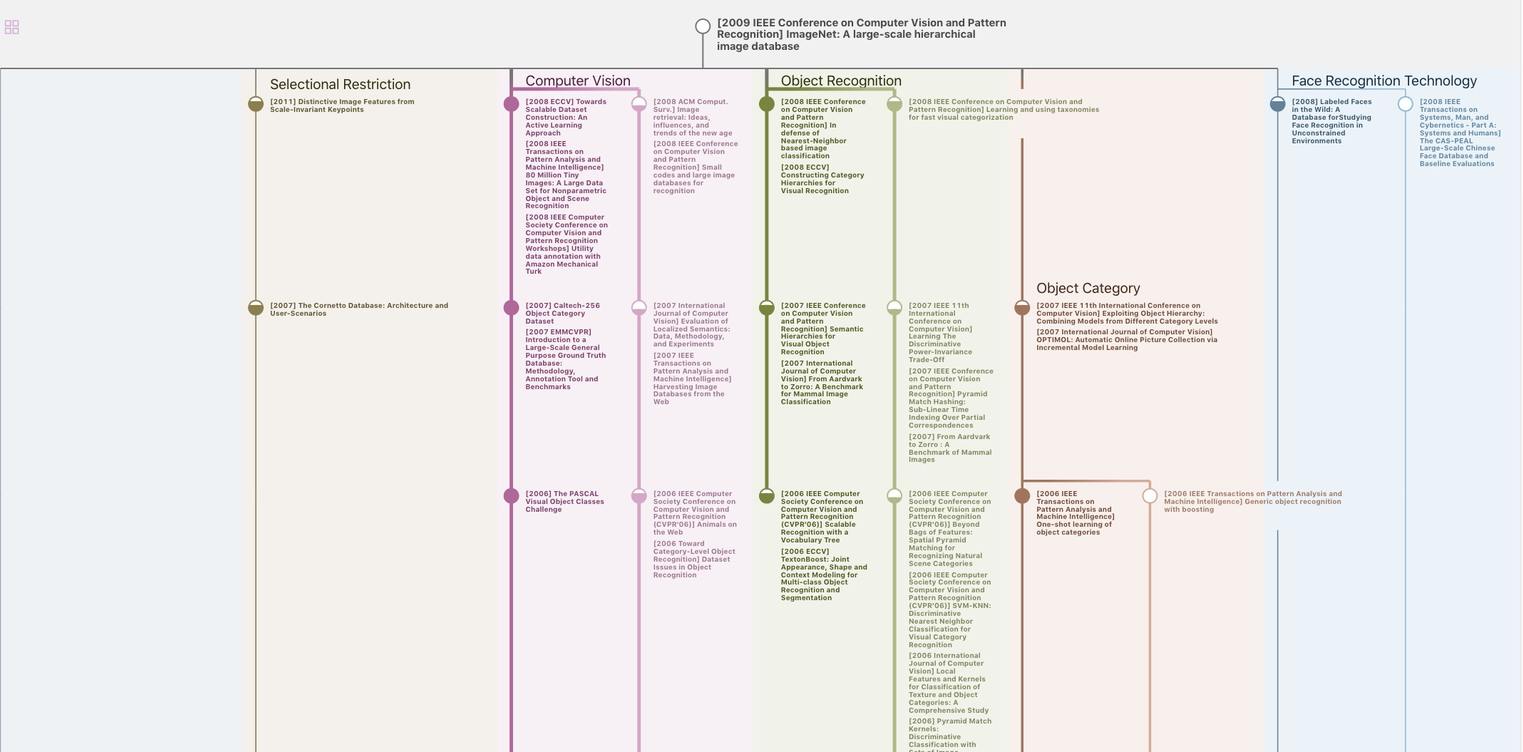
生成溯源树,研究论文发展脉络
Chat Paper
正在生成论文摘要