Variable Selection and Oil- Gas- Bearing Evaluation Using Multicomponent Seismic Data and AMPSO-ML Approaches
SPE JOURNAL(2023)
摘要
Several challenges exist in the application of machine learning (ML) algorithms to reservoir prediction, such as the low accuracy of the reservoir prediction model, long training time, and complicated parameter adjustment. A good optimization algorithm is one of the keys to successfully training an ML model. Therefore, this study focuses on improving the ability of ML models to obtain rich reservoir characteristic information from multicomponent seismic data through an intelligent optimization algorithm and uses this information to predict the oil and gas distribution accurately. Adaptive mutation particle swarm optimization (AMPSO) was used to train the ML models [artificial neural network (ANN) and least- squares support vector machine (LSSVM)] and obtain intelligent prediction models (AMPSOANN and AMPSO- LSSVM) for multicomponent seismic reservoir prediction. First, a hyperparameter analysis of the ML models was conducted to determine their structure, and the parameters of the two models were searched globally by AMPSO to obtain an intelligent prediction model for reservoir distribution prediction. The two prediction models were then applied to synthetic reservoir prediction data to confirm their reliability and validity. Finally, they were applied to real data for multicomponent seismic gas reservoir distribution prediction to verify their accuracy. The predictive abilities of the two intelligent models on synthetic and real data sets were compared with those of the unoptimized ANN and support vector machine (SVM) models to verify their validity. The results showed that AMPSO improved the prediction ability of the two models and the prediction accuracy of oil/gas- bearing distribution on the synthetic data and gas- bearing distribution on the real data.
更多查看译文
关键词
multicomponent seismic data,seismic data,variable selection,oil-gas-bearing
AI 理解论文
溯源树
样例
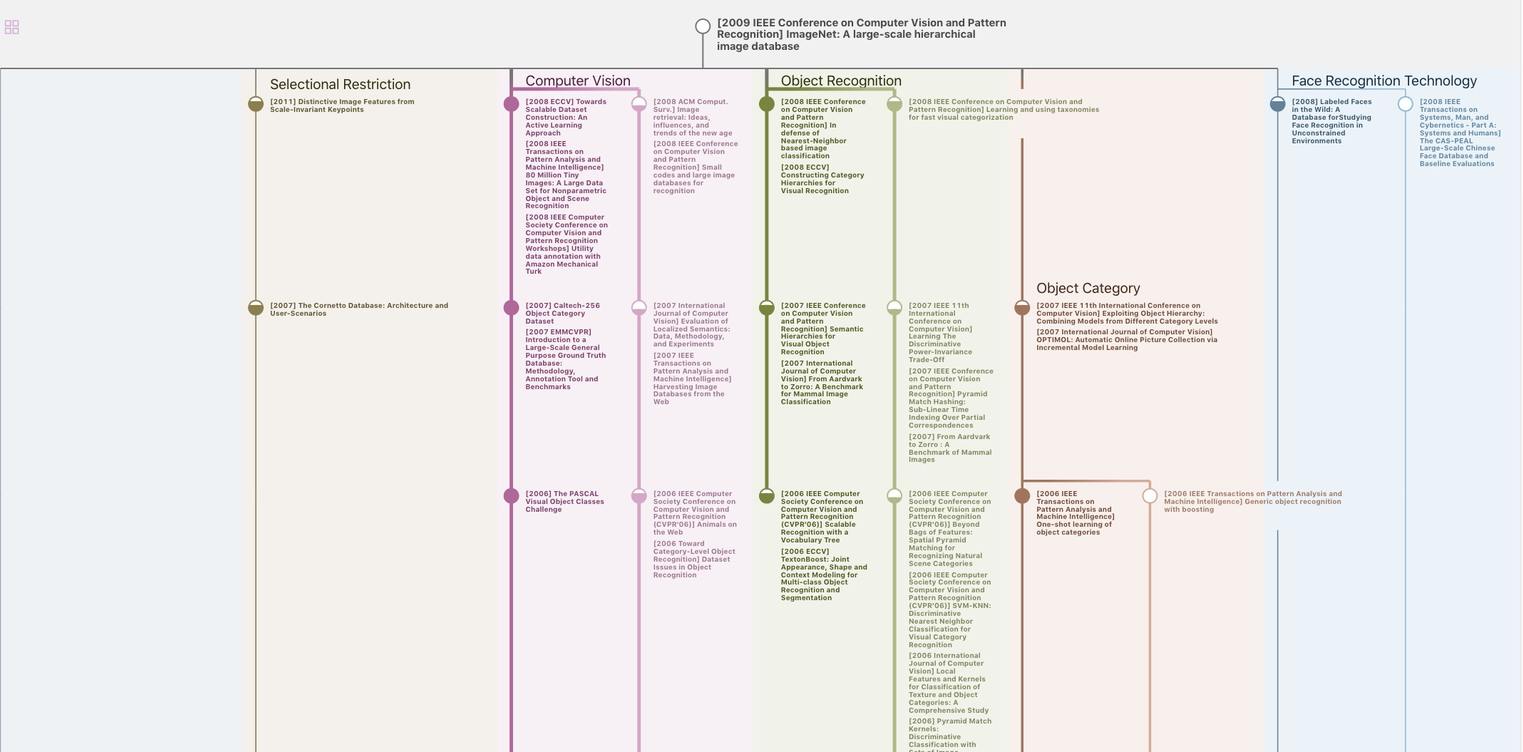
生成溯源树,研究论文发展脉络
Chat Paper
正在生成论文摘要