Artificial neural network based correction for reduced order models in computational fluid mechanics
COMPUTER METHODS IN APPLIED MECHANICS AND ENGINEERING(2023)
摘要
In this paper we propose a reduced order model (ROM) for incompressible flows based on proper orthogonal decomposition (POD) and having a finite element approximation as full order model (FOM). The main idea is to start from a purely POD-based ROM, projecting the equations onto the ROM space, and then add a nonlinear correction that depends on the ROM unknowns to enhance the final ROM model. This correction is based on the fact that we do have some available high fidelity data, namely, the snapshots. Thus, the correcting term is built as an artificial neural network (ANN) constructed with the snapshots as the training set. This correction is then introduced in the fully discrete ROM system to achieve more accurate solutions. A further feature of our approach is that we construct both the ROM and the FOM using the variational multi-scale (VMS) concept, and this allows us to understand the correction term as an approximation to the scales that are lost when passing from the FOM to the ROM. The resulting corrected ROM has a significant higher accuracy than the original one.
更多查看译文
关键词
Reduced order models,Proper orthogonal decomposition,Variational multi-scale,Artificial Neural Networks,Correction models
AI 理解论文
溯源树
样例
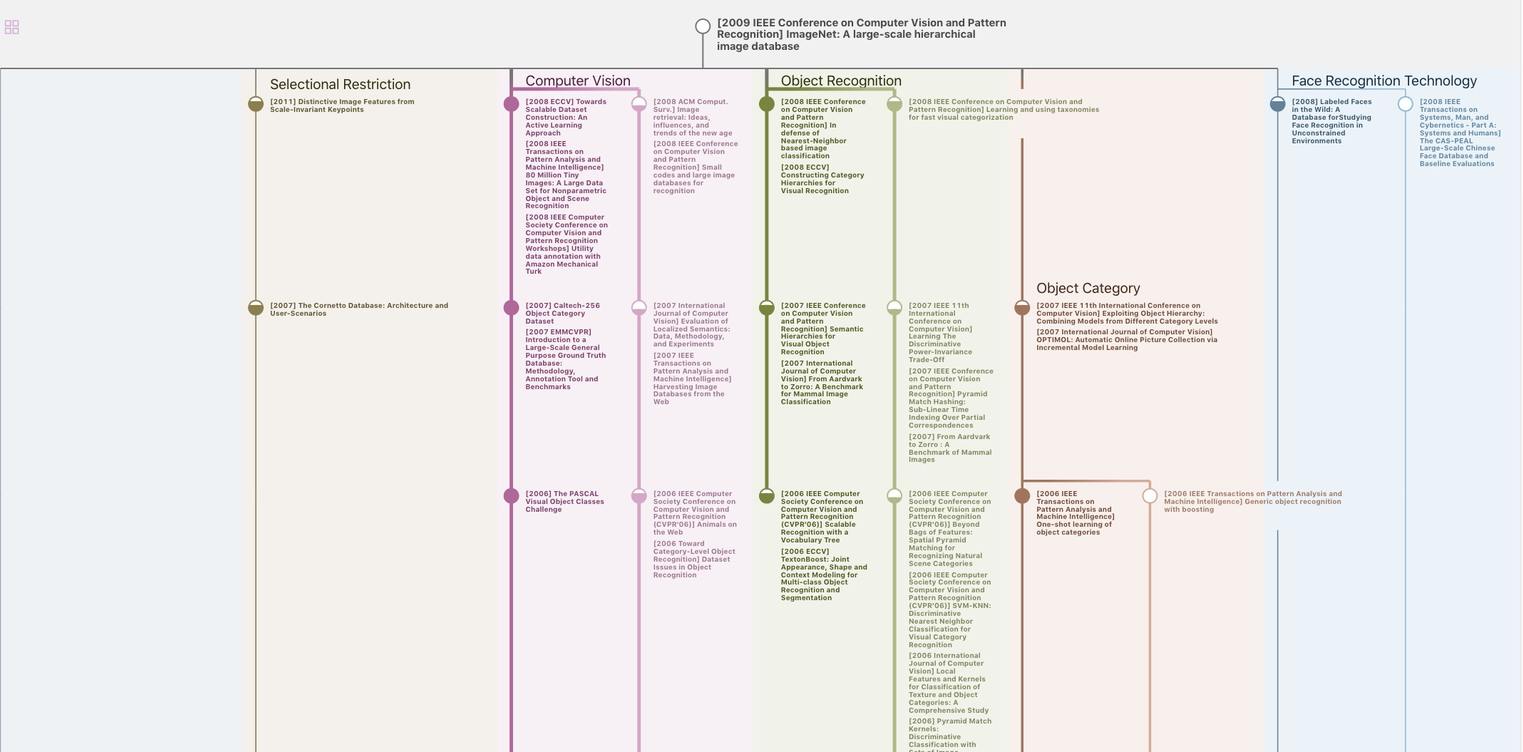
生成溯源树,研究论文发展脉络
Chat Paper
正在生成论文摘要