Data-Driven Hospital Admission Control: A Learning Approach
OPERATIONS RESEARCH(2023)
摘要
The choice of care unit upon admission to the hospital is a challenging task because of the wide variety of patient characteristics, uncertain needs of patients, and limited number of beds in intensive and intermediate care units. The care unit placement decisions involve capturing the trade-off between the benefit of better health outcomes versus the opportunity cost of reserving higher level of care beds for potentially more complex patients arriving in the future. By focusing on reducing the readmission risk of patients, we develop an online algorithm for care unit placement under the presence of limited reusable hospital beds. The algorithm is designed to (i) adaptively learn the readmission risk of patients through batch learning with delayed feedback and (ii) choose the best care unit placement for a patient based on the observed information and the occupancy level of the care units. We prove that our online algorithm admits a Bayesian regret bound. We also investigate and assess the effectiveness of our methodology using hospital system data. Our numerical experiments demonstrate that our methodology outperforms different benchmark policies.
更多查看译文
关键词
online learning, bandit, regret analysis, data-driven admission control, readmission
AI 理解论文
溯源树
样例
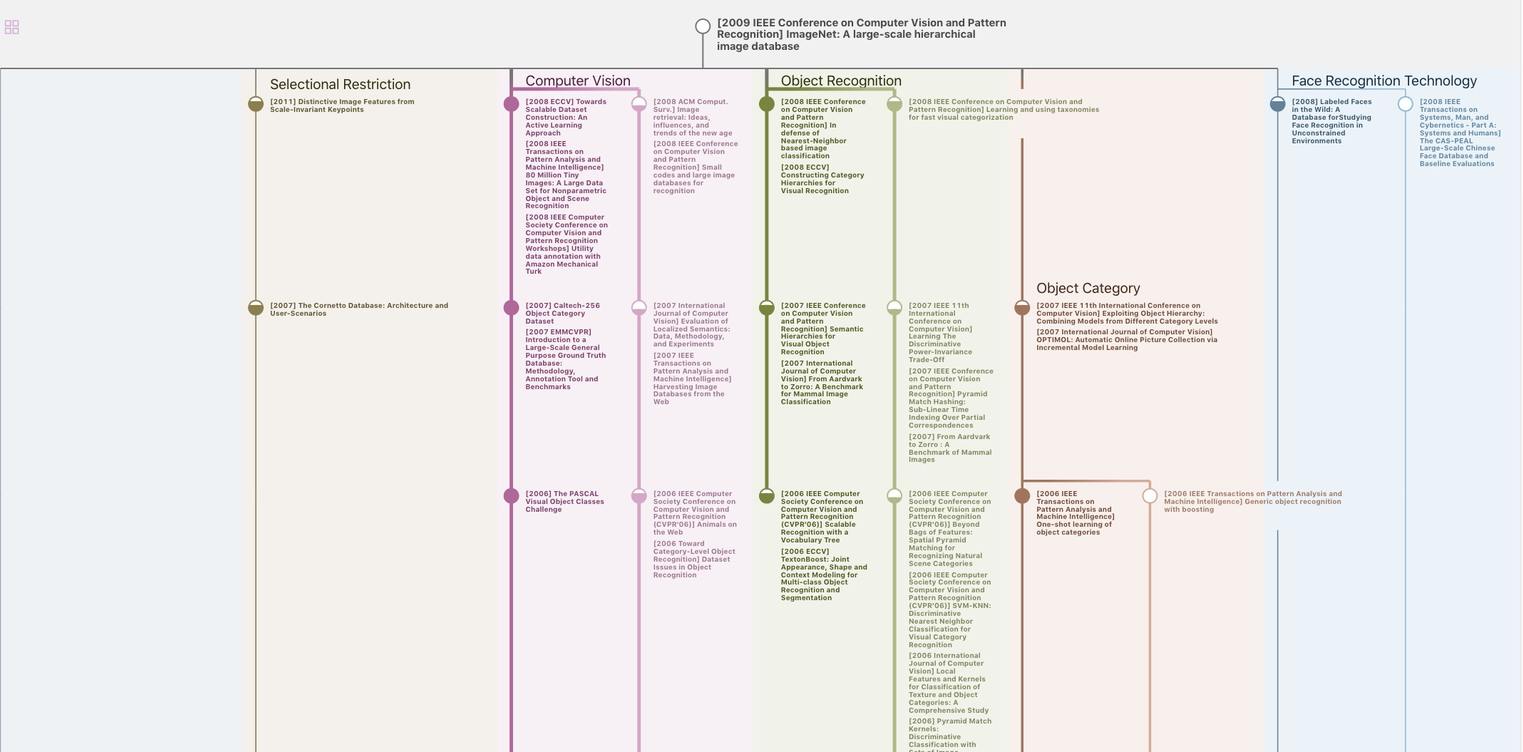
生成溯源树,研究论文发展脉络
Chat Paper
正在生成论文摘要