Learning-Based Risk-Bounded Path Planning Under Environmental Uncertainty
IEEE TRANSACTIONS ON AUTOMATION SCIENCE AND ENGINEERING(2023)
摘要
Building a general and efficient path planning framework in uncertain nonconvex environments is challenging due to the safety constraints and complex configuration. Traditional avenues usually involve convexifying obstacles and presume Gaussian distribution, which are not universal. Meanwhile, the fast convergence of high-quality solutions is not guaranteed. Therefore, we develop a novel neural risk-bounded path planner to quickly find near-optimal solutions that have an acceptable collision probability in the complex environments. Firstly, we retrieve the nonconvex obstacles with arbitrary probabilistic uncertainties in the form of a deterministic point cloud map. A neural network sampler encodes it into a latent embedding and is trained with sufficient expert demonstrations, predicting states in the potential subspace. We construct a neural cost estimator to select the best informed state from those samples. Then, we recursively use the simple yet effective neural networks to march toward the start and goal bidirectionally. The collision risk of the intermediate connections is verified based on sum-of-squares optimization. Simulation results show that our approach significantly saves time and resources in finding comparable solutions over the state-of-the-art methods in the seen and unseen challenging environments. Note to Practitioners-More and more robots are deployed in unstructured environments, such as forests and subterranean caves. However, uncertainty in the environment situational awareness usually causes accidents. To quickly generate safe paths without over-conservation in uncertain complex environments, we propose a neural risk-bounded sampling-based path planner. Conventional methods consume lots of computation time and resources to generate satisfactory results. Our learning-based risk-bounded path planning framework can efficiently find paths with a guaranteed risk tolerance avoiding uncertain nonconvex static obstacles. It imitates the expert to generate informed states in a subspace that potentially contains the optimal solution. In practice, we need to formulate the observed uncertain obstacle at a grid map into the polynomial containing random variables and determine their probability distributions.
更多查看译文
关键词
Sampling-based algorithm,deep learning methods,risk-bounded path planning
AI 理解论文
溯源树
样例
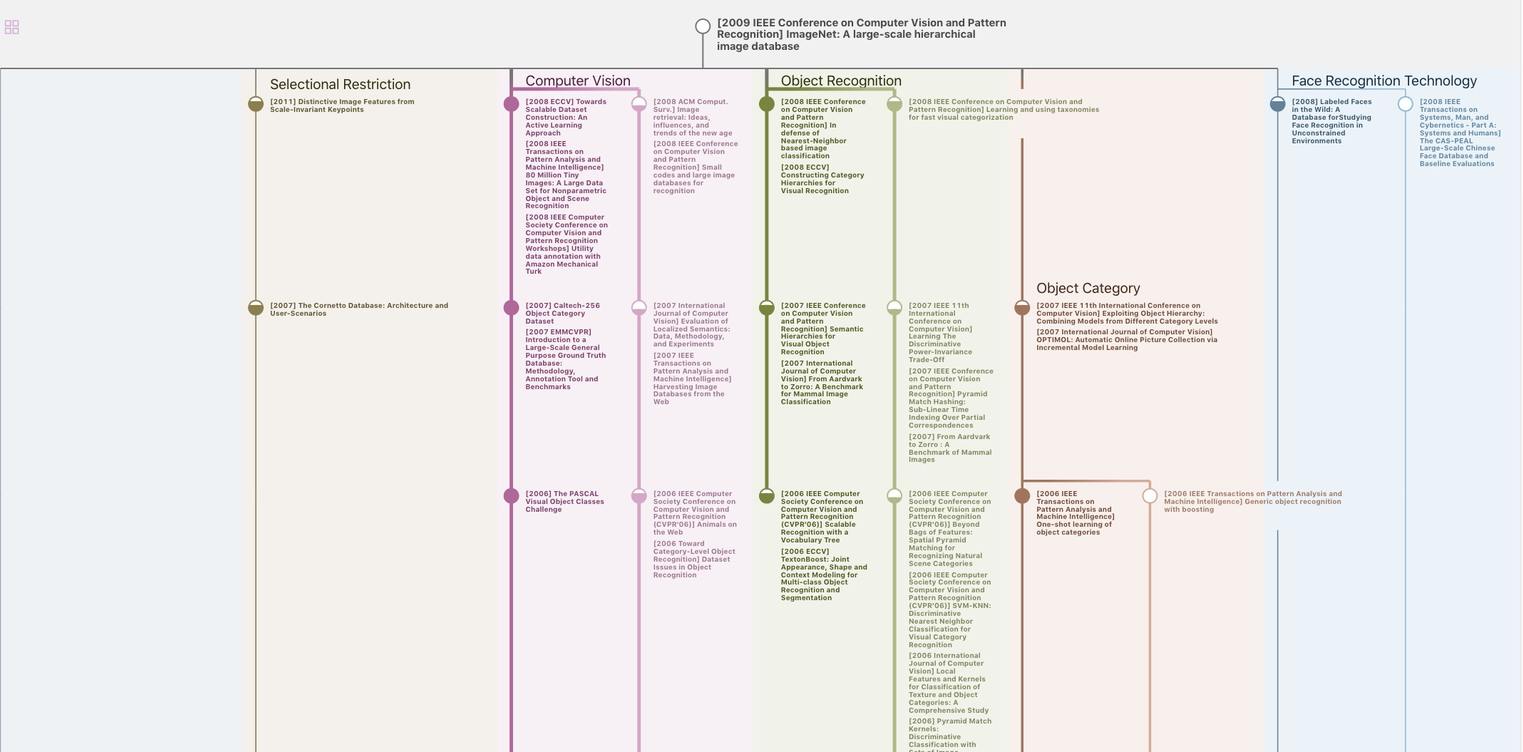
生成溯源树,研究论文发展脉络
Chat Paper
正在生成论文摘要