Multi-scale Contrastive Learning with Attention for Histopathology Image Classification
MEDICAL IMAGING 2023(2023)
摘要
Whole slide images (WSIs) in histopathology naturally provide multi-scale information. Several previous studies have shown that leveraging such multi-scale information in histopathology image analysis is effective to improve performance. Here, we propose making use of recent advances in contrastive learning and self-attention techniques in multi-scale WSIs for cancer subtype classification using weak labels. The proposed method is based on a Siamese architecture to share a common encoder network for images on different scales to reduce the model size and training cost. In addition, we propose a variant of the self-attention module specifically designed for multi-scale WSIs so that the network can focus on important textural features across different image scales. We assess the efficacy of the proposed method via an ablation study on a real intrahepatic cholangiocarcinoma dataset. The result confirms that our method outperforms conventional multi-scale models with fewer model parameters.
更多查看译文
关键词
Multi-scale, Contrastive learning, Attention, Digital pathology
AI 理解论文
溯源树
样例
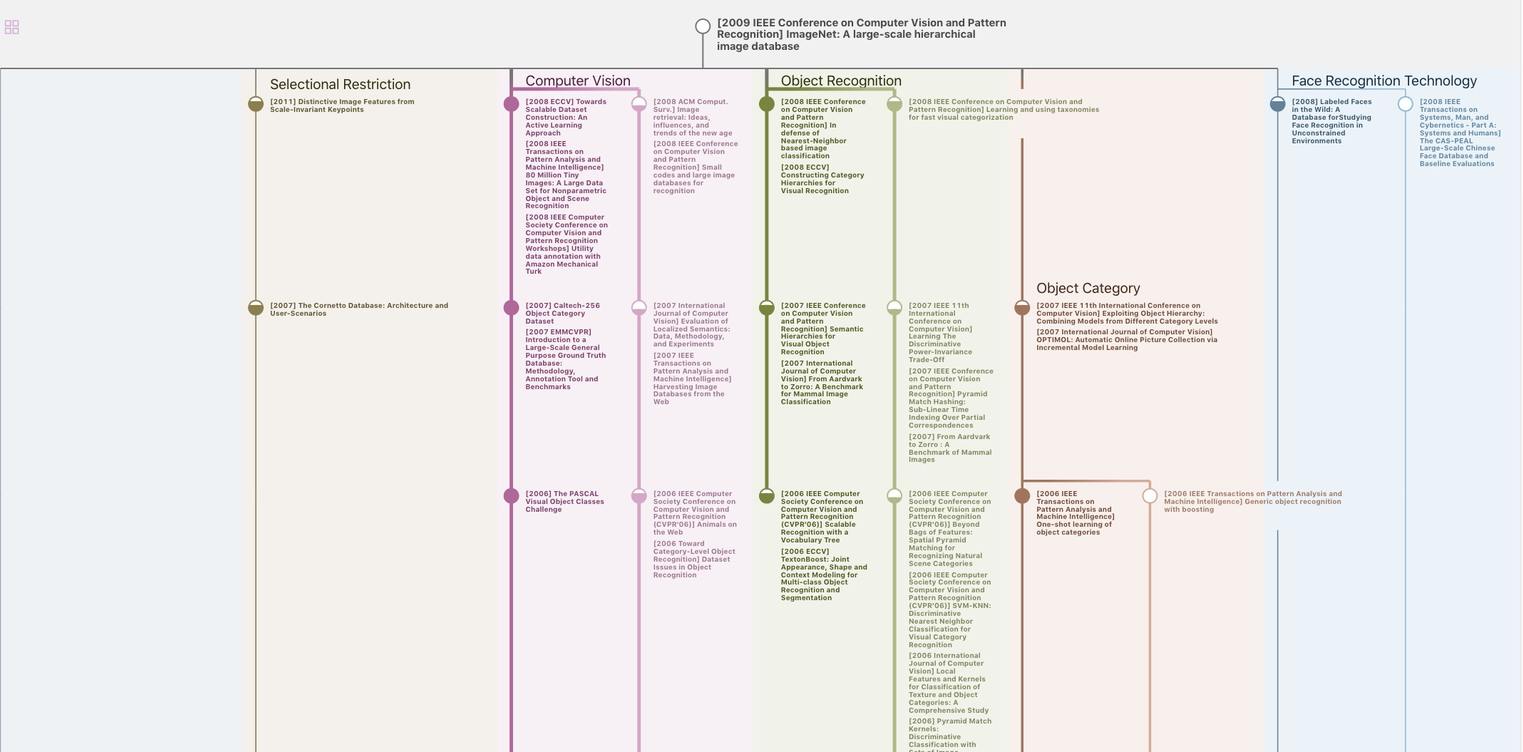
生成溯源树,研究论文发展脉络
Chat Paper
正在生成论文摘要