Self-supervised denoising using optimized blind-spot networks for real-time application in 4D-OCT
MEDICAL IMAGING 2023(2023)
摘要
As in other imaging modalities, noise decreases image quality in optical coherence tomography (OCT), which is especially problematic in real-time intra-surgical application, where multi-frame averaging is not available. In this work, we present an adapted self-supervised training approach to train a blind-spot denoising network for OCT data. With the proposed method, the stability of the method is improved, avoiding the occurrence of artifacts by increasing realism of training data. We show that using this approach, the quality of two-dimensional B-scans can be improved qualitatively and quantitatively even without paired training data. This improvement is also translated into live volumetric renderings composed of denoised two-dimensional scans, even when using only very small network complexities due to harsh time constraints.
更多查看译文
AI 理解论文
溯源树
样例
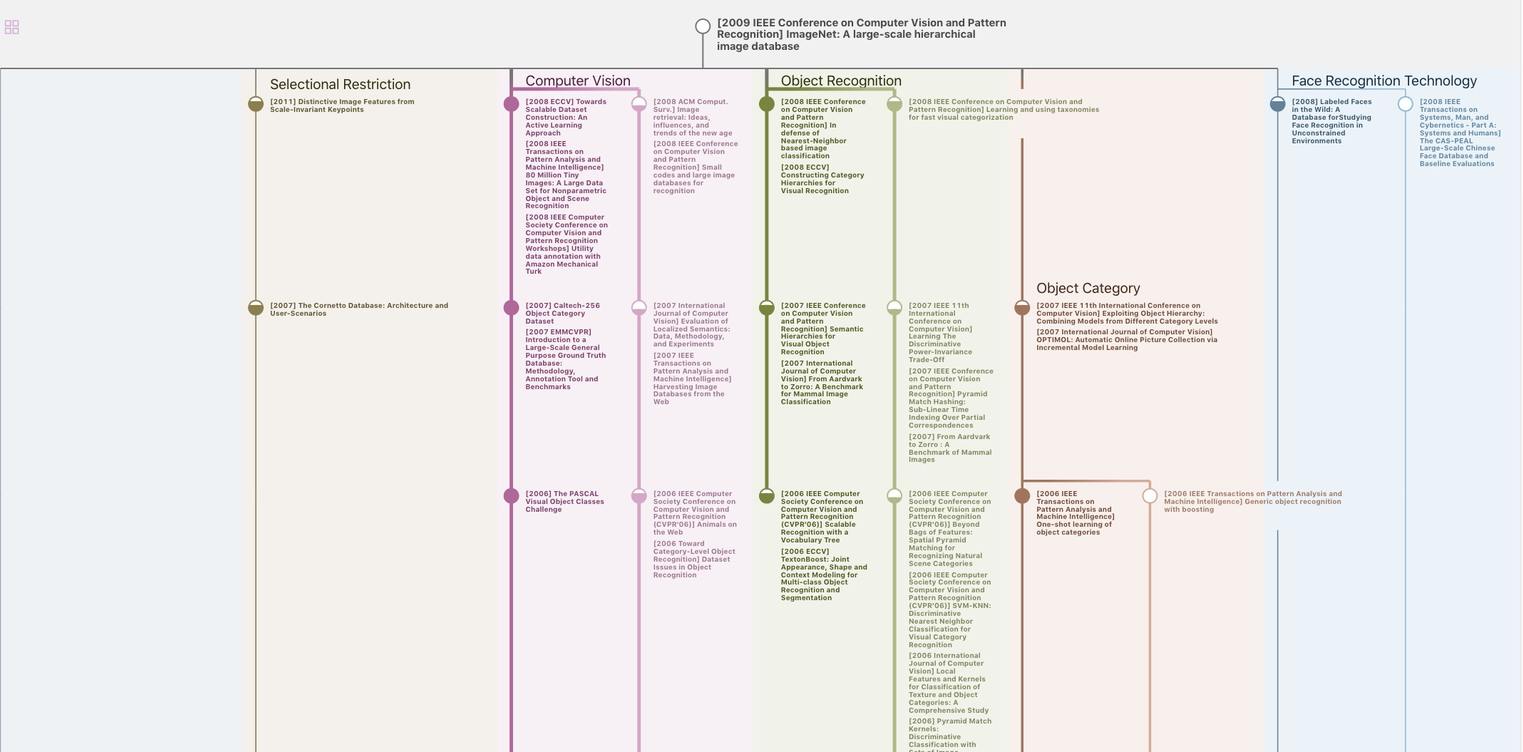
生成溯源树,研究论文发展脉络
Chat Paper
正在生成论文摘要