Enhancing PINNs for solving PDEs via adaptive collocation point movement and adaptive loss weighting
NONLINEAR DYNAMICS(2023)
摘要
Physics-informed neural networks (PINNs) are an emerging method for solving partial differential equations (PDEs) and have been widely applied in the field of scientific computing. In this paper, we introduce a novel adaptive PINN model for solving PDEs. The model draws on the idea of traditional adaptive methods and incorporates the adaptive collocation point movement method into the PINNs model. It can use residual information from the PDE or characteristics of the solution function itself to guide the movement of collocation points, giving an appropriate distribution of collocation points for specific problems, improving the predictive accuracy of the model, and avoiding overfitting. Additionally, the model introduces an adaptive loss weighting strategy, which updates adaptive weights continuously by minimizing negative log-likelihood estimation to achieve adaptive weighting of the loss function, thereby improving the convergence rate and accuracy of the model. Finally, we conduct extensive experiments, including the one-dimensional Poisson equation, two-dimensional Poisson equation, Burgers equation, Klein–Gordon equation, Helmholtz equation, and Lid-Driven problem, to demonstrate the effectiveness and accuracy of the proposed model. The experimental results show that the model can significantly improve predictive accuracy and generalization ability. The data and code can be found at https://github.com/hsbhc/AMAW-PINN .
更多查看译文
关键词
Physics-informed neural networks,PDEs,Adaptive collocation points,Loss weighting
AI 理解论文
溯源树
样例
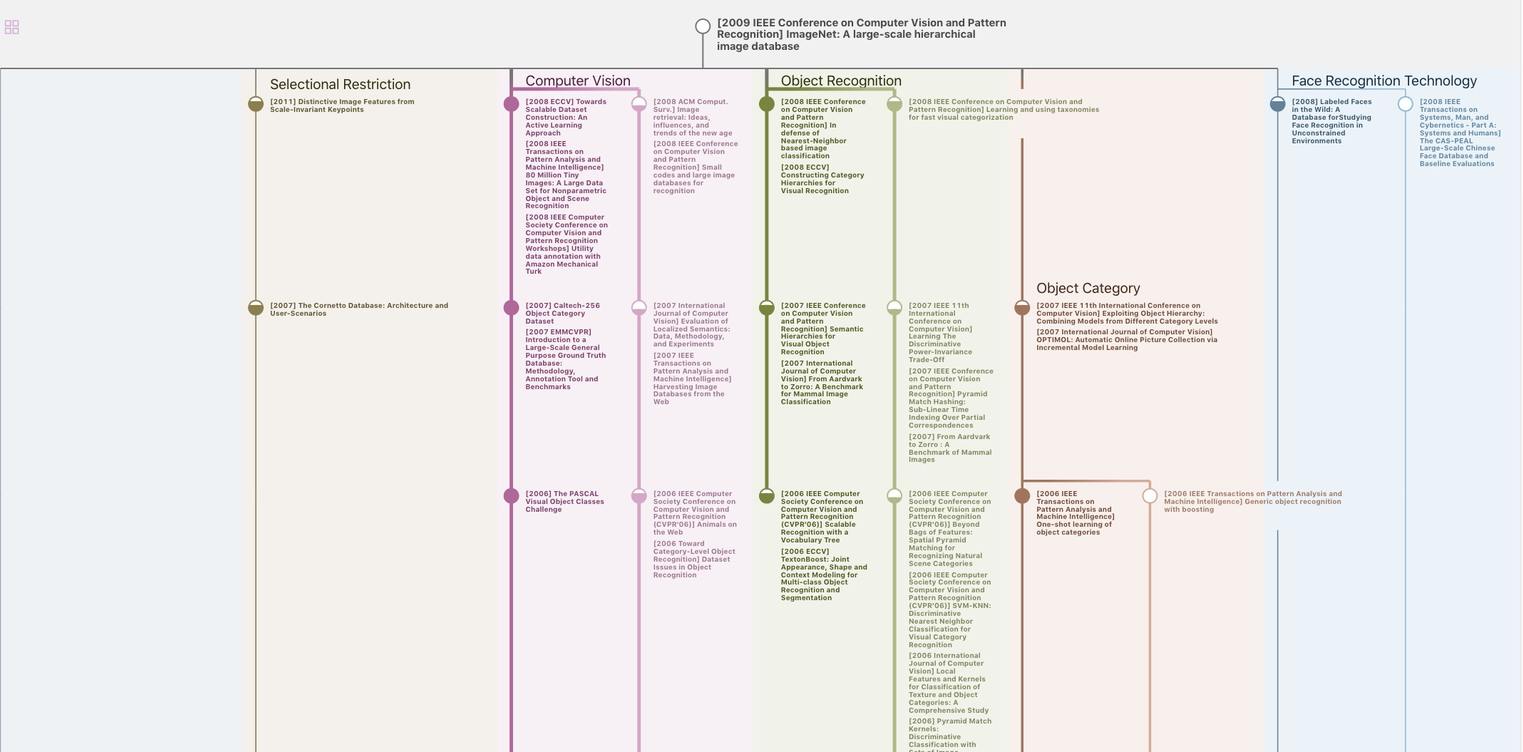
生成溯源树,研究论文发展脉络
Chat Paper
正在生成论文摘要