The Prediction of Supercooled Large Drops by a Microphysics and a Machine Learning Model for the ICICLE Field Campaign
WEATHER AND FORECASTING(2023)
摘要
The prediction of supercooled large drops (SLD) from the Thompson-Eidhammer (TE) microphysics scheme-run as part of the High-Resolution Rapid Refresh (HRRR) model-is evaluated with observations from the In-Cloud Icing and Large drop Experiment (ICICLE) field campaign. These observations are also used to train a random forest machine learning (ML) model, which is then used to predict SLD from several variables derived from HRRR model output. Results provide insight on the limitations and benefits of each model. Generally, the ML model results in an increase in the probability of detection (POD) and false alarm rate (FAR) of SLD compared to prediction from TE micro-physics. Additionally, the POD of SLD increases with increasing forecast lead time for both models, likely since clouds and precipitation have more time to develop as forecast length increases. Since SLD take time to develop in TE microphysics and may be poorly represented in short-term (<3 h) forecasts, the ML model can provide improved short-term guidance on supercooled large-drop icing conditions. Results also show that TE microphysics predicts a frequency of SLD in cold (<-108C) or high ice water content (IWC) environments that is too low compared to observations, whereas the ML model better captures the relative frequency of SLD in these environments.
更多查看译文
关键词
supercooled large drops,icicle field campaign,microphysics
AI 理解论文
溯源树
样例
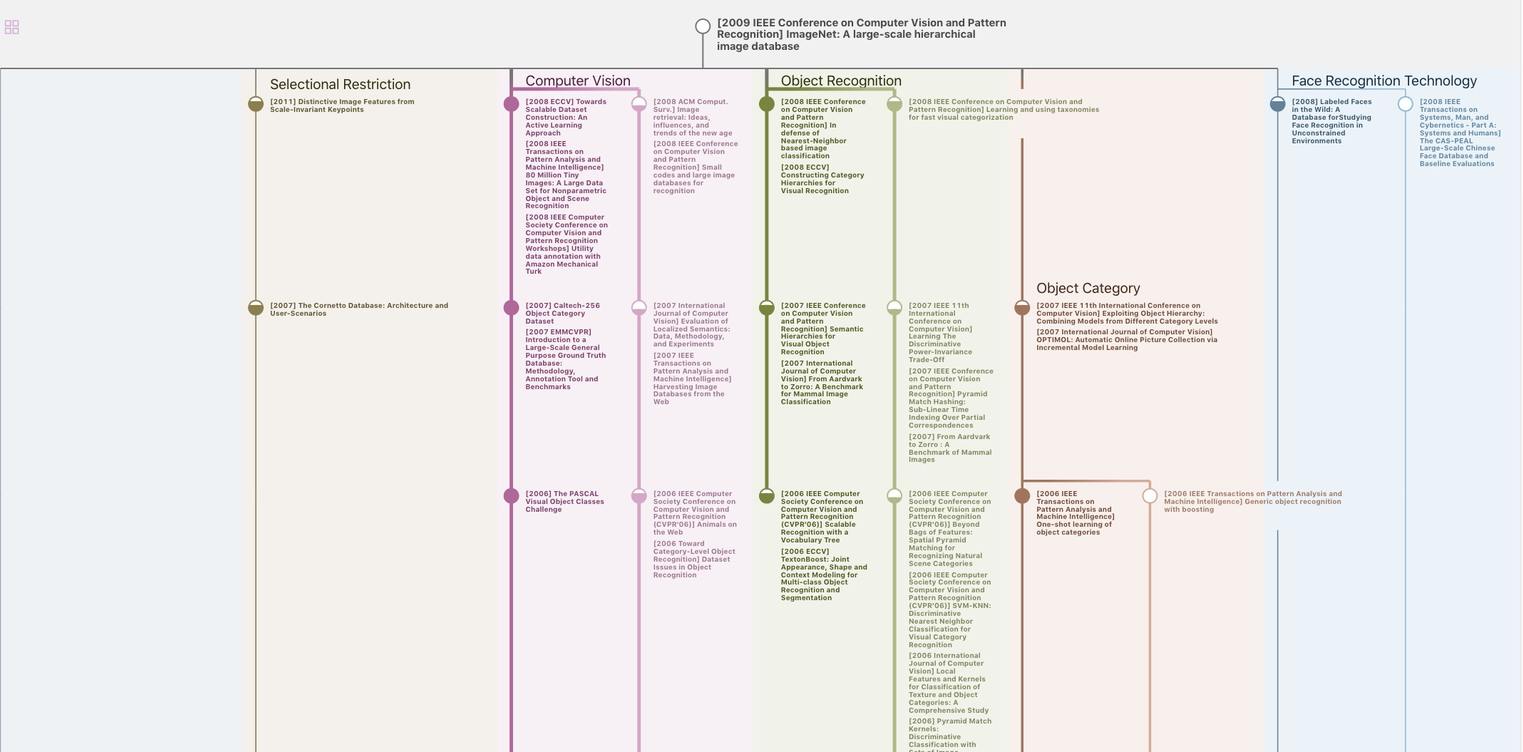
生成溯源树,研究论文发展脉络
Chat Paper
正在生成论文摘要