MFGCN: an efficient graph convolutional network based on multi-order feature information for human skeleton action recognition.
Neural Comput. Appl.(2023)
Abstract
With the development of depth sensors and pose estimation algorithms, human skeleton action recognition based on graph convolutional networks has acquired widespread attention and application. The latest methods achieve dynamically learning different topologies for modeling and use first-order, second-order, and third-order features, i.e., joint, bone, and motion representations, which has led to high accuracy. However, many models are still confused by actions that have similar motion trajectories, and most of the existing methods model the spatial dimension before the temporal dimension, whereas in fact, spatial and temporal information should be interrelated. In this paper, we propose an efficient graph convolutional network based on multi-order feature information (MFGCN) for human skeleton action recognition. Firstly, our method introduces angle features (noted as fourth-order features), which are implicitly embedded in other third-order features by encoding angular features, to powerfully capture detailed features in the spatio-temporal dimension and enhance the ability to distinguish similar actions. Secondly, we use a content-adaptive approach to construct the adjacency matrix and dynamically learn the topology between the skeleton joints. Finally, we develop a spatio-temporal information sliding extraction module (STISE) to improve the inter-correlation of spatial and temporal information. The proposed method has extensively experimented on the NTU-RGB D, NTU-RGB D 120, and Northwestern-UCLA datasets, and the experimental results show that our method can achieve superior performance compared to the current state-of-the-art methods.
MoreTranslated text
Key words
Human skeleton,Action recognition,Graph convolutional networks,Angle feature
AI Read Science
Must-Reading Tree
Example
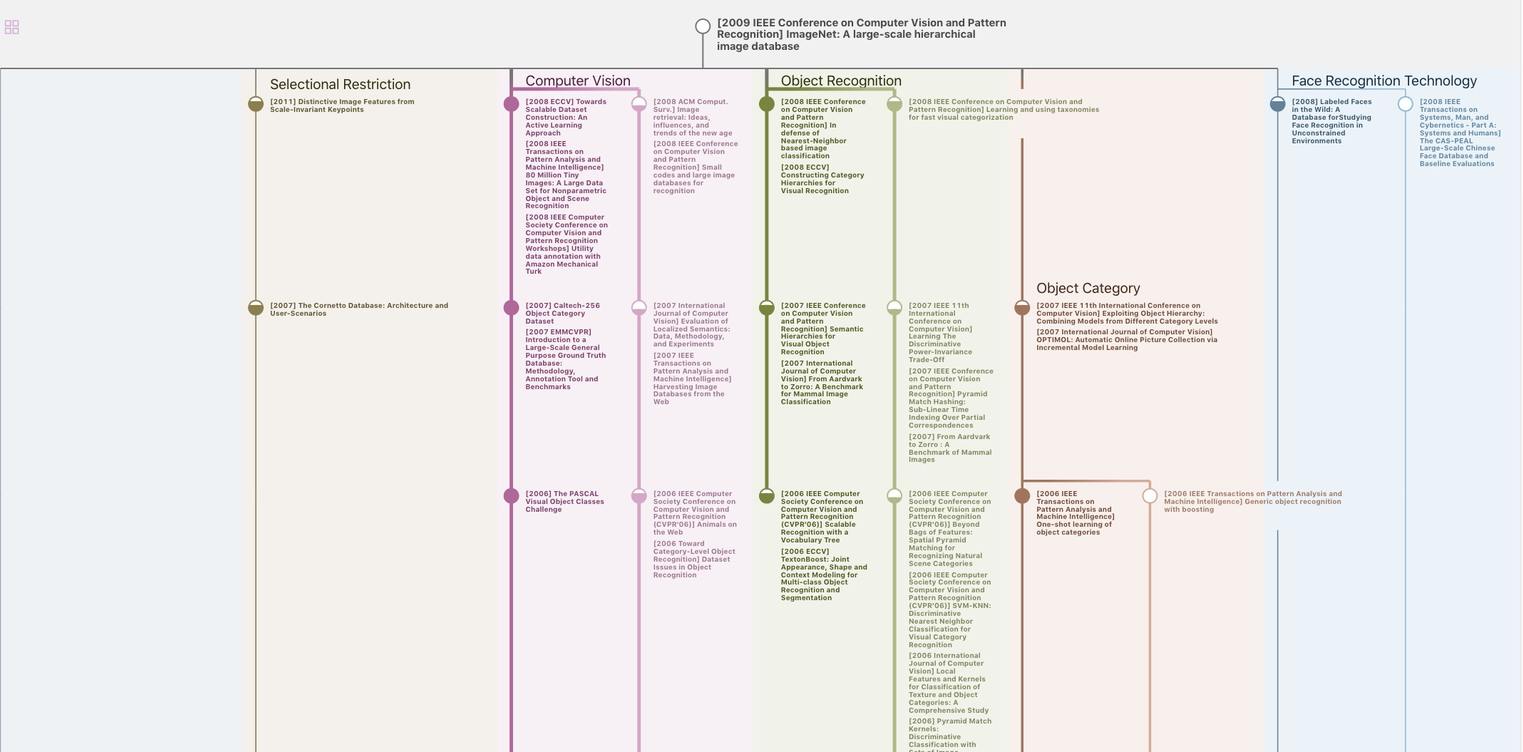
Generate MRT to find the research sequence of this paper
Chat Paper
Summary is being generated by the instructions you defined