Synthetic lung ultrasound data generation using autoencoder with generative adversarial network
JOURNAL OF THE ACOUSTICAL SOCIETY OF AMERICA(2023)
摘要
The goal of this study is to test the applicability of a generative adversarial network (GAN) to solve the class unbalancing problem in lung ultrasound (LUS) data. We introduce a supervised autoencoder with conditional latent space. During training, the generator utilizes the weights of the decoder and conditional latent space to generate synthetic LUS images, whereas the discriminator utilizes the weights of the encoder together with the class labels, which also allows classifying each synthetic image into the different classes. A customized gradient penalty loss function was utilised. This approach is tested on a dataset costing of 6500 LUS images, collected from 35 COVID-19 patients and labelled according to a validated 4 level scoring system [10.1109/TMI.2020.2994459]. 1000 synthetic images were generated to balance this dataset. The quality of the synthetic images was evaluated through similarity measures, computed with respect to training and unseen data. Moreover, the synthetic images were also evaluated by expert clinicians concerting their capability to mimic a realistic LUS image. In conclusion, the proposed approach appears to be capable to solve the class unbalancing problem by generating LUS images carrying novel information content, comparable to that of real data from new patients.
更多查看译文
关键词
autoencoder,ultrasound,lung
AI 理解论文
溯源树
样例
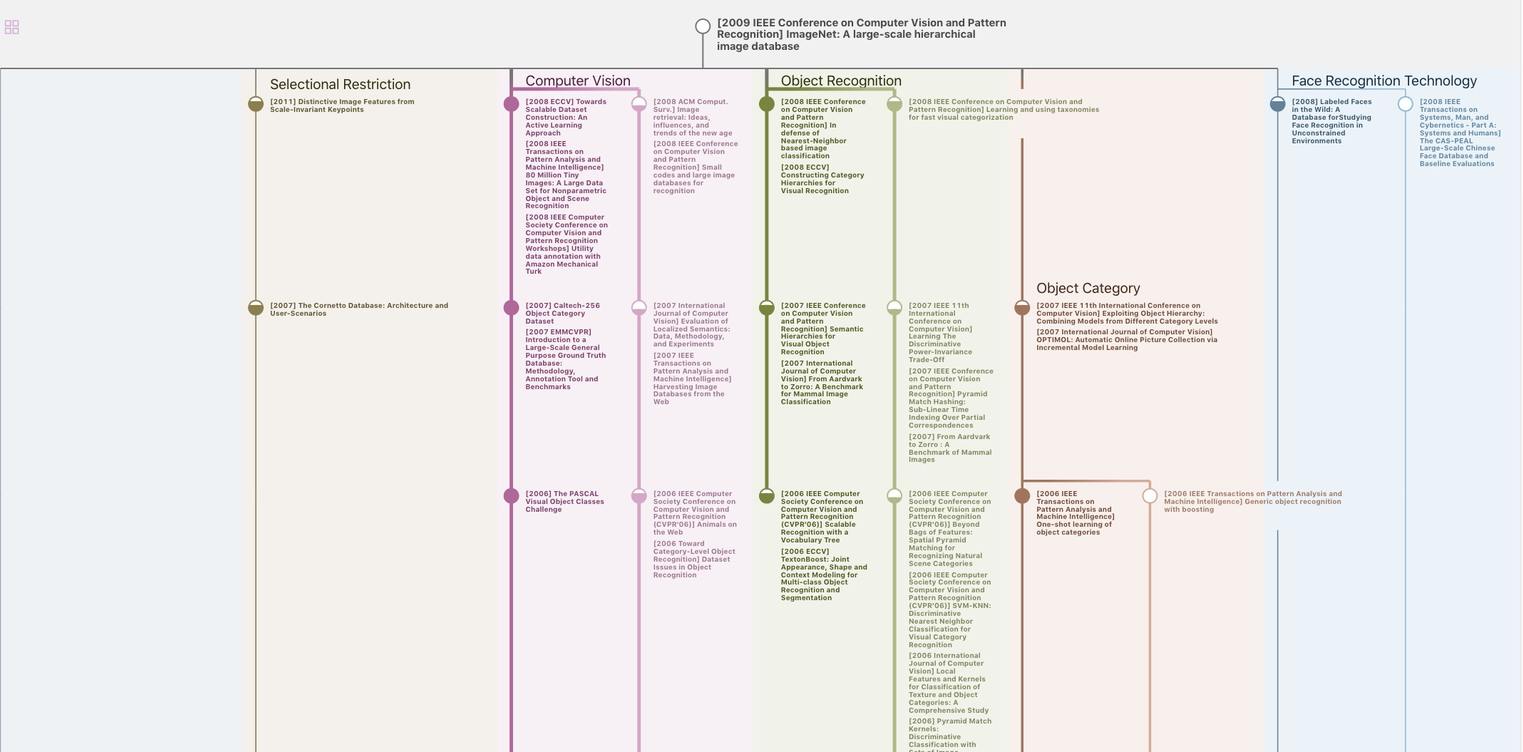
生成溯源树,研究论文发展脉络
Chat Paper
正在生成论文摘要