Improving passive acoustic target detection using machine learning classifiers
JOURNAL OF THE ACOUSTICAL SOCIETY OF AMERICA(2023)
摘要
The ocean covers 70% of the Earth surface area, yet over 80% of it remains unexplored despite the advances in underwater acoustics and oceanography. Ocean exploration is critical for accurate climate model development, renewable energy applications, and in understanding the marine habitat. Further exploration necessitates improvements in underwater navigation with Autonomous Underwater Vehicles (AUVs). Utilizing acoustic landmarks can enhance AUV localization performance [Fula et al., Oceans IEEE/MTS (2018)]. Passive markers called Acoustic Identification (AID) Tags have unique and identifiable acoustic reflection signatures designed to function as landmarks [Satish et al., JASA 149 (2021)]. These targets can be detected by Match Filtering returns with template signals. Match Filtering performance is limited in the presence of strong interfering signals, and with changes in sound speeds that alters the temporal structure of these signatures. The application of a Machine Learning classifiers for detecting AID tag signatures can improve the localization performance. Through implementation of Logistic Regression, Deep Neural Networks, and Convolutional Neural Networks, the generalizability and superiority of these models is demonstrated. We report high accuracies (>90 %) in a multilabel (8 classes) classification task with signals with low SNR (–6 dB) and strong interference (+12 dB).
更多查看译文
关键词
passive acoustic target detection,machine learning
AI 理解论文
溯源树
样例
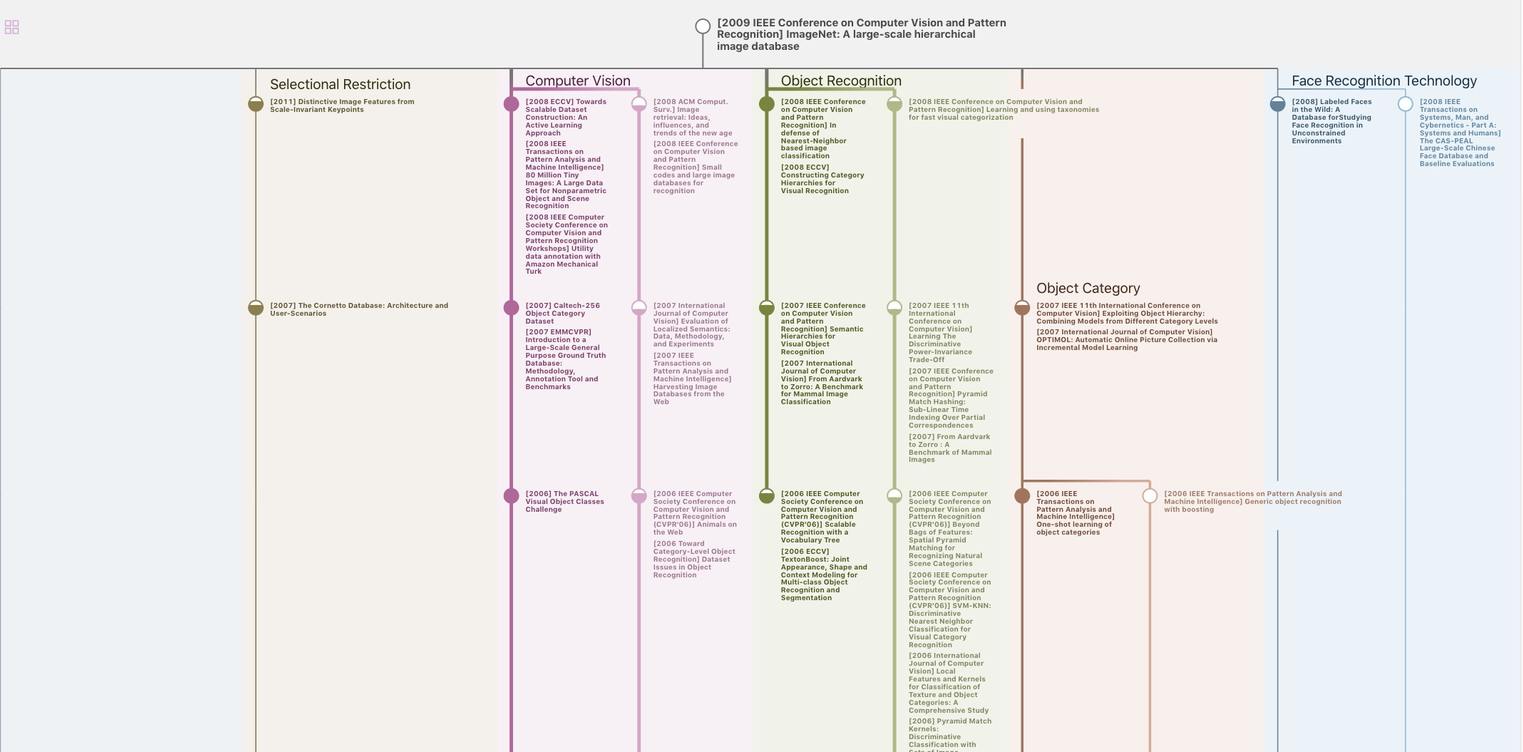
生成溯源树,研究论文发展脉络
Chat Paper
正在生成论文摘要