Approximate Gaussian variance inference for state-space models
INTERNATIONAL JOURNAL OF ADAPTIVE CONTROL AND SIGNAL PROCESSING(2023)
摘要
State-space models require an accurate knowledge of the process error (Q) and measurement error (R) covariance matrices for exact state estimation. Even though the matrix R can be, in many situations, considered to be known from the measuring instrument specifications, it is still a challenge to infer the Q matrix online while providing reliable estimates along with a low computational cost. In this article, we propose an analytically tractable online Bayesian inference method for inferring the Q matrix in state-space models. We refer to this method as approximate Gaussian variance inference (AGVI) using which we are able to treat the error variance and covariance terms in the full Q matrix as Gaussian hidden states and infer them simultaneously with the other hidden states in a closed-form manner. The two case studies show that the method is able to provide statistically consistent estimates for the mean and uncertainties of the error variance terms for univariate and multivariate cases. The method also exceeds the performance of the existing adaptive Kalman filter methods both in terms of accuracy and computational efficiency.
更多查看译文
关键词
Bayesian inference, closed-form inference, Gaussian multiplicative approximation, online parameter estimation, process error covariance matrix, state-space models
AI 理解论文
溯源树
样例
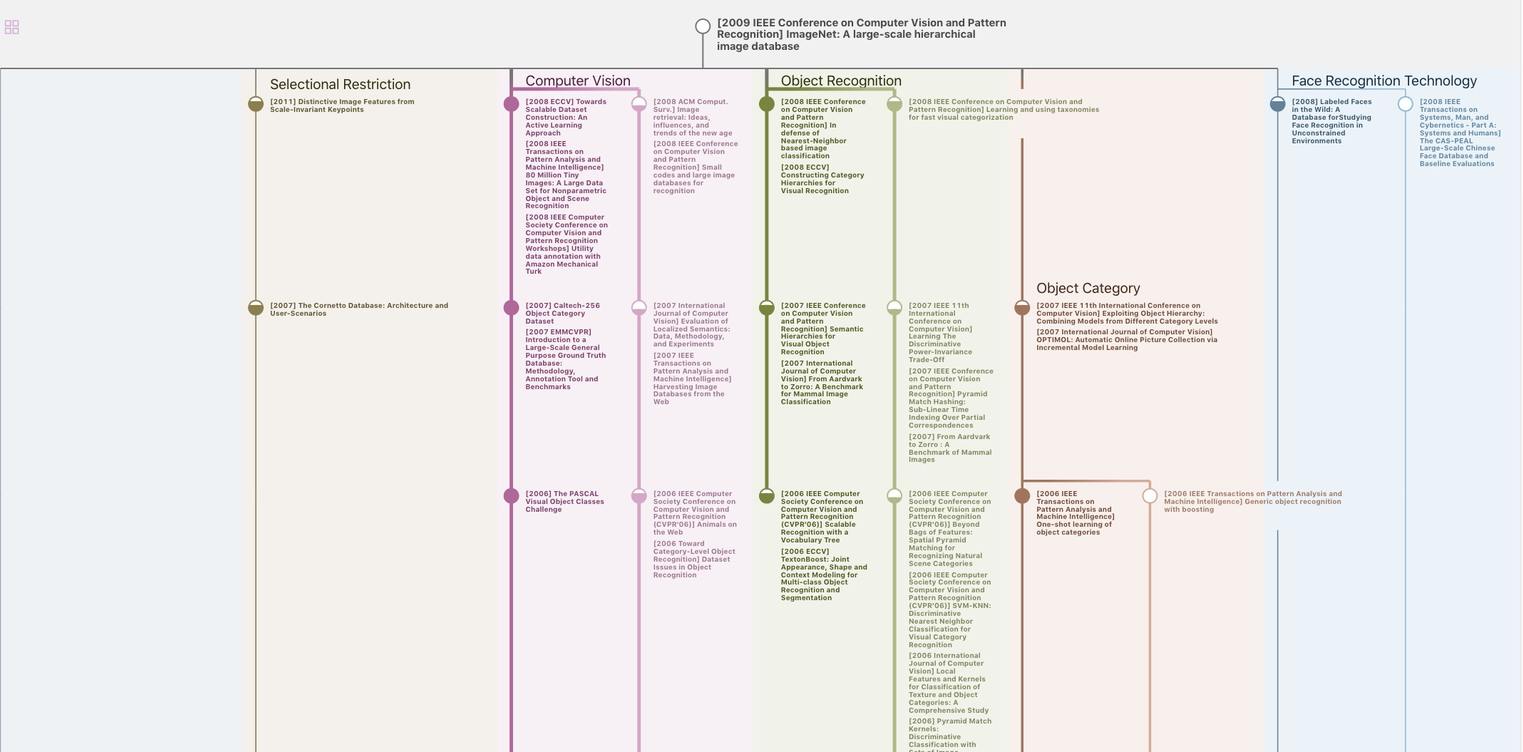
生成溯源树,研究论文发展脉络
Chat Paper
正在生成论文摘要