Navigating autonomous vehicles in uncertain environments with distributional reinforcement learning
PROCEEDINGS OF THE INSTITUTION OF MECHANICAL ENGINEERS PART D-JOURNAL OF AUTOMOBILE ENGINEERING(2023)
Abstract
Navigation in complex urban environments is very difficult, mainly due to perceptual uncertainty caused by obstructions in the field of view. The perceptual uncertainty is mainly due to sensors obstructed by obstacles. This has a significant impact on the safety of autonomous vehicles. Existing approaches based on partially observable Markov decision processes or reinforcement learning for the uncertainty problem may lead to conservative planning and expensive computation. We propose caution-driven networks combined with distributional reinforcement learning fully parameterized quantile function, which termed Intrinsic caution module - fully parameterized quantile function (ICM-FQF). The method is also applied in the continuous action space. The method is used to evaluate two challenging scenarios, pedestrians crossing with occlusion and a unsignalized intersection with a limited field of view. The algorithm is trained and evaluated using the CARLA simulator. Compared to conventional RL algorithms, the method makes smarter decisions and reduces the rate of collisions.
MoreTranslated text
Key words
distributional reinforcement learning,autonomous vehicles,uncertain environments
AI Read Science
Must-Reading Tree
Example
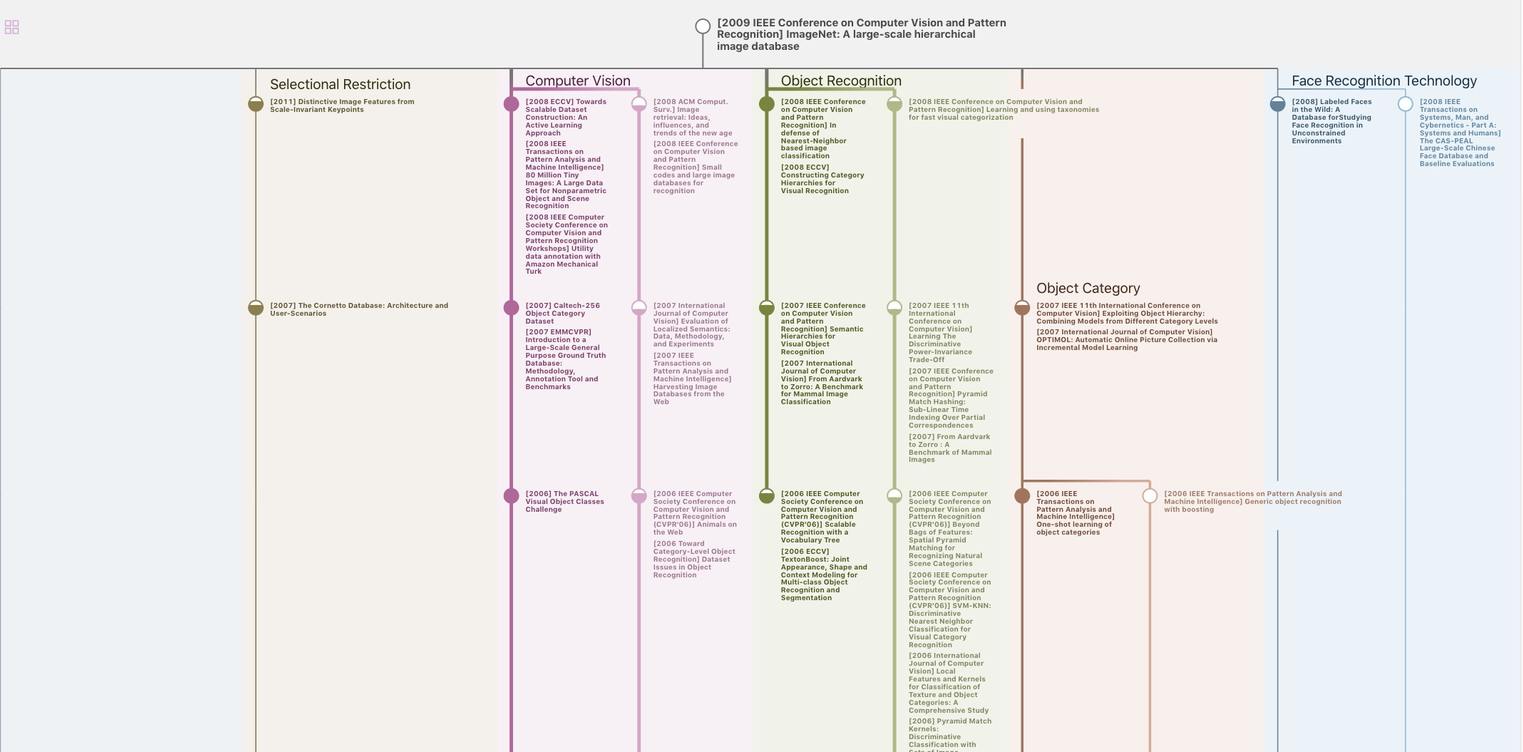
Generate MRT to find the research sequence of this paper
Chat Paper
Summary is being generated by the instructions you defined