Utilising physics-informed neural networks for optimisation of diffusion coefficients in pseudo-binary diffusion couples
PHILOSOPHICAL MAGAZINE(2023)
摘要
We propose a numerical inverse method based on physics-informed neural networks (PINN) for calculating composition-dependent diffusion coefficients in pseudo-binary (PB) diffusion couples in multicomponent alloys. Traditional methods rely solely on experimental diffusion profiles as design targets, which can lead to unreliable estimates. In contrast, PINN uses a combination of available data and physics-based constraints to obtain optimised design parameters and exact solutions for constrained governing differential equations. The constraints in PINN include governing partial differential equations, initial and boundary conditions, and any other equality/inequality relations obeyed by physical parameters. Our study shows the necessity of experimentally estimated intrinsic diffusion coefficients for the prediction of reliable composition-dependent mobility data. In the absence of such data, different combinations of unknown intrinsic diffusion coefficients can also produce reasonable approximations of diffusion profiles and interdiffusion coefficients while wrongly predicting more fundamental quantities (i.e. intrinsic diffusivities). Our method utilises PINN to simultaneously obtain optimised design parameters (diffusion coefficients) and exact solutions for governing diffusion equations. The implementation of PINN uses experimentally estimated diffusion coefficients obtained by the PB diffusion couple method, in addition to diffusion profiles, as design targets. The method is extended to non-ideal PB diffusion profiles, such as conventional diffusion couples in which all the components develop diffusion profiles, by incorporating additional constraints such as zero composition gradient of certain component(s) and experimentally estimated interdiffusion coefficients at extrema in the composition profiles. PINN is found to be a promising approach for obtaining reliable estimates of diffusion coefficients in multicomponent alloys.
更多查看译文
关键词
diffusion coefficients,neural networks,optimisation,physics-informed,pseudo-binary
AI 理解论文
溯源树
样例
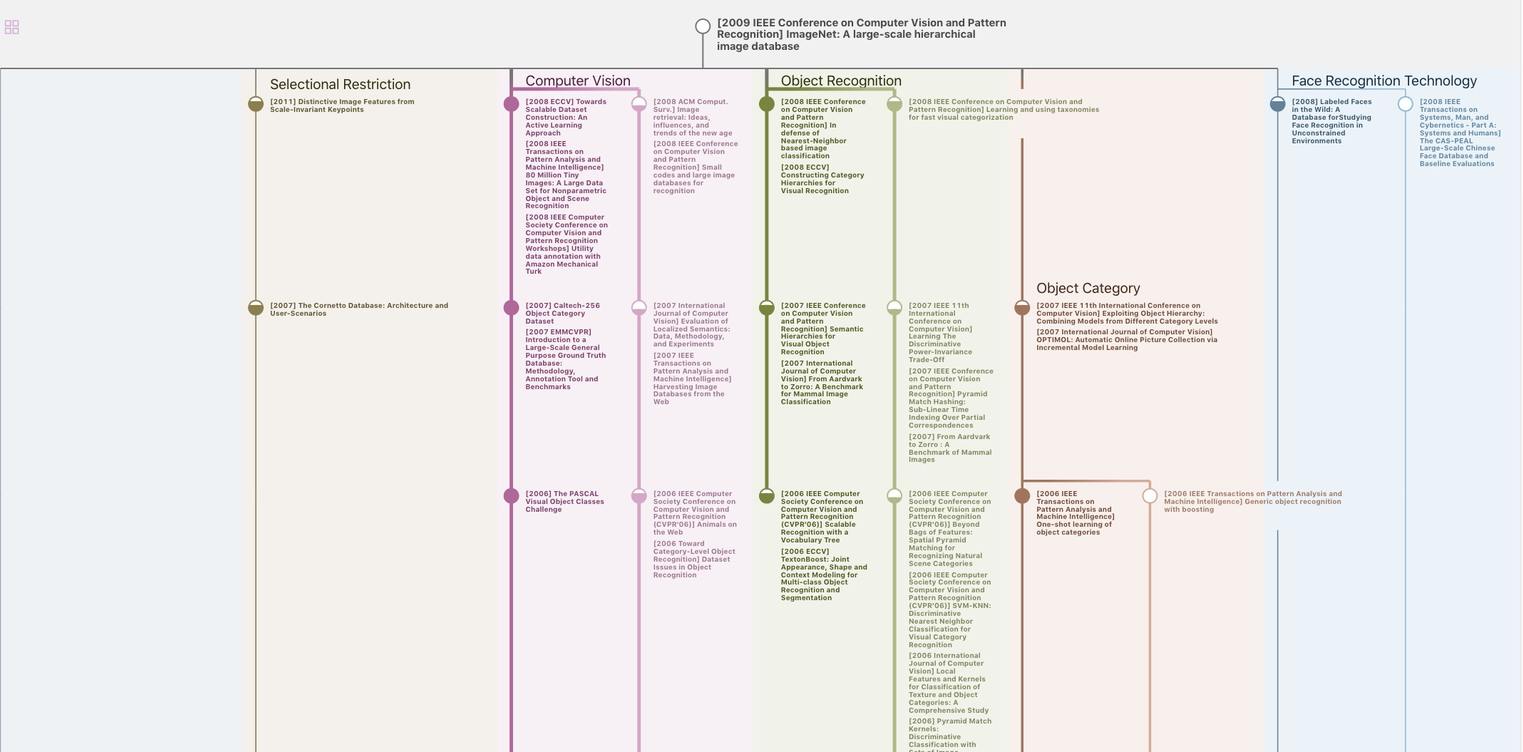
生成溯源树,研究论文发展脉络
Chat Paper
正在生成论文摘要