Research progress and application of deep learning in remaining useful life, state of health and battery thermal management of lithium batteries
JOURNAL OF ENERGY STORAGE(2023)
摘要
Lithium batteries are considered to be one of the most promising green energy sources in the future. However, the problems of prognostic and health management are the main factors restricting the application and development of lithium batteries. Therefore, an efficient and intelligent battery management system (BMS) is very important. In recent years, with the continuous development of deep learning (DL), it has shown a good research prospect in the BMS. In this paper, the application of DL in the prediction the of remaining useful life (RUL), state of health (SOH) and battery thermal management (BTM) of lithium batteries of different methods are systematically reviewed. This review evaluates different deep learning approaches to battery estimation and prediction in terms of predictive performance, advantages, and disadvantages. In addition, the review discusses the characteristics, achievements, limitations, and directions for improvement of different algorithms in the above applications for factors affecting charge and discharge cycles, complex environments, dynamic conditions, and different battery types. Key issues and challenges in terms of computational complexity and various internal and external factors are identified. Finally, the future opportunities and directions are discussed to design a more efficient and intelligent algorithm model, which can adapt to more advanced BMS.
更多查看译文
关键词
Lithium battery, Deep learning, Remaining useful life, State of health, Battery thermal management
AI 理解论文
溯源树
样例
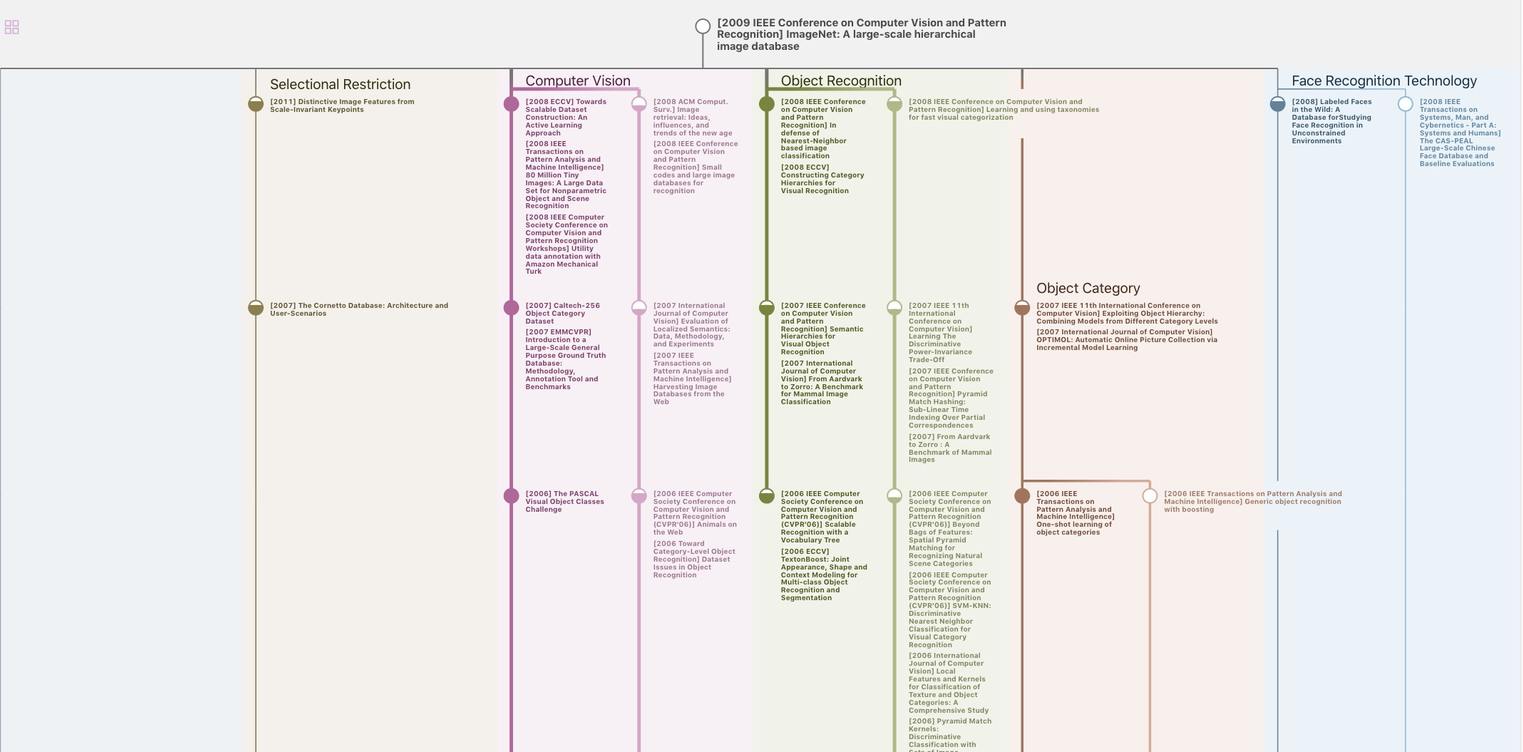
生成溯源树,研究论文发展脉络
Chat Paper
正在生成论文摘要