Optimal Control Algorithm for Subway Train Operation by Proximal Policy Optimization
APPLIED SCIENCES-BASEL(2023)
摘要
With the increasing scale of the urban subway, the total energy consumption of the subway has increased dramatically and poses a great challenge to the comfort of passengers and the punctuality of train operation. In order to ensure on-time train operation and passenger comfort, and at the same time reduce the energy consumption of subway operation, this paper proposes a Proximal Policy Optimization (PPO)-based optimization algorithm for the optimal control of subway train operation. Firstly, a reinforcement learning architecture for optimal control of subway train operation is constructed with the position and speed of train operation as the reinforcement learning state, energy consumption and comfort as the optimization objectives, and train operation time as the constraint. The proposed reinforcement learning model is trained by the PPO algorithm, and the reward scaling is added to the training process to accelerate the training speed and improve the efficiency of the algorithm. The experimental results show that the proposed PPO with reward scaling algorithm can effectively reduce train energy consumption and improve passenger comfort while ensuring on-time train operation.
更多查看译文
关键词
proximal policy optimization,subway train operation,optimal control
AI 理解论文
溯源树
样例
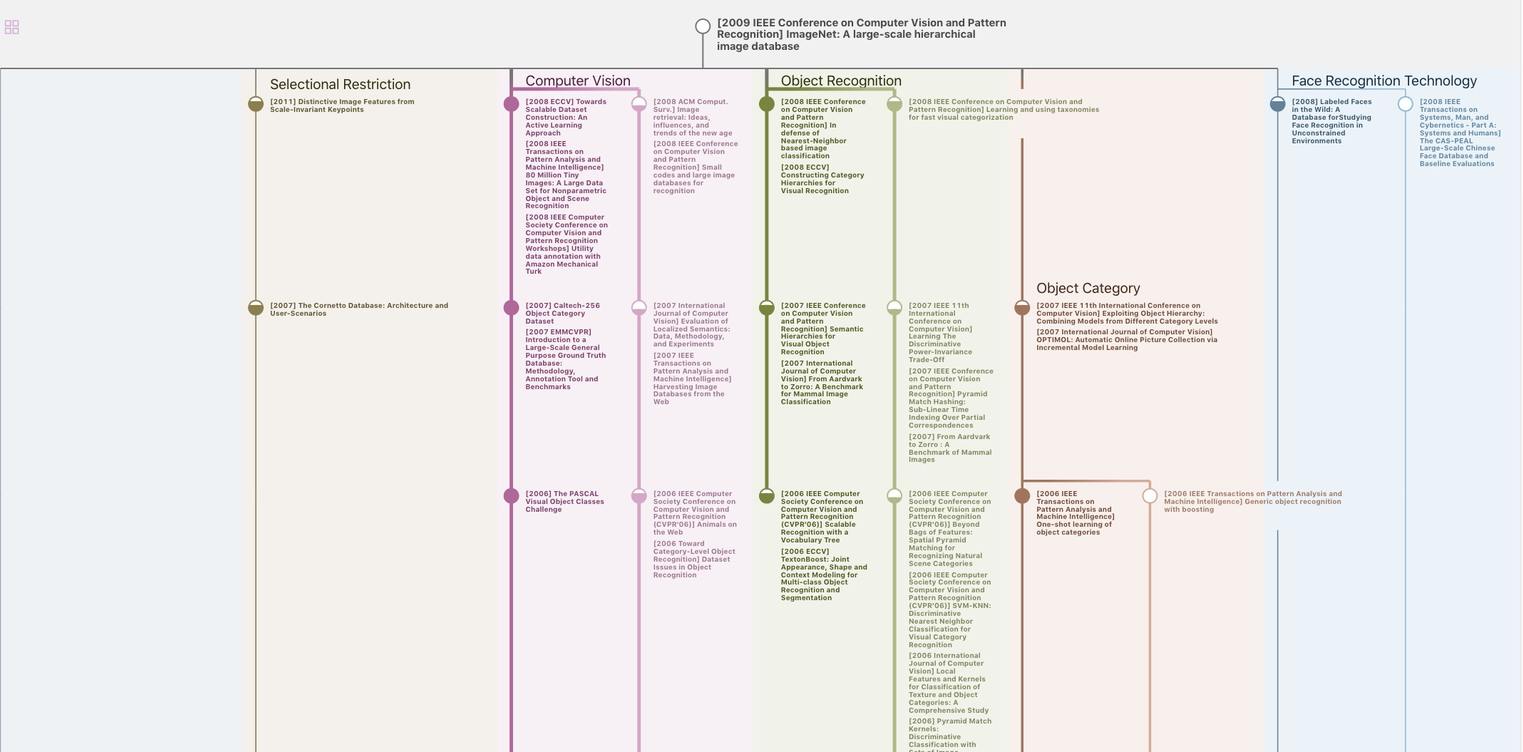
生成溯源树,研究论文发展脉络
Chat Paper
正在生成论文摘要