Fusing time-varying mosquito data and continuous mosquito population dynamics models
FRONTIERS IN APPLIED MATHEMATICS AND STATISTICS(2023)
摘要
Climate change is arguably one of the most pressing issues affecting the world today and requires the fusion of disparate data streams to accurately model its impacts. Mosquito populations respond to temperature and precipitation in a nonlinear way, making predicting climate impacts on mosquito-borne diseases an ongoing challenge. Data-driven approaches for accurately modeling mosquito populations are needed for predicting mosquito-borne disease risk under climate change scenarios. Many current models for disease transmission are continuous and autonomous, while mosquito data is discrete and varies both within and between seasons. This study uses an optimization framework to fit a non-autonomous logistic model with periodic net growth rate and carrying capacity parameters for 15 years of daily mosquito time-series data from the Greater Toronto Area of Canada. The resulting parameters accurately capture the inter-annual and intra-seasonal variability of mosquito populations within a single geographic region, and a variance-based sensitivity analysis highlights the influence each parameter has on the peak magnitude and timing of the mosquito season. This method can easily extend to other geographic regions and be integrated into a larger disease transmission model. This method addresses the ongoing challenges of data and model fusion by serving as a link between discrete time-series data and continuous differential equations for mosquito-borne epidemiology models.
更多查看译文
关键词
data fusion,non-autonomous model,logistic growth,mosquito populations,differential equation
AI 理解论文
溯源树
样例
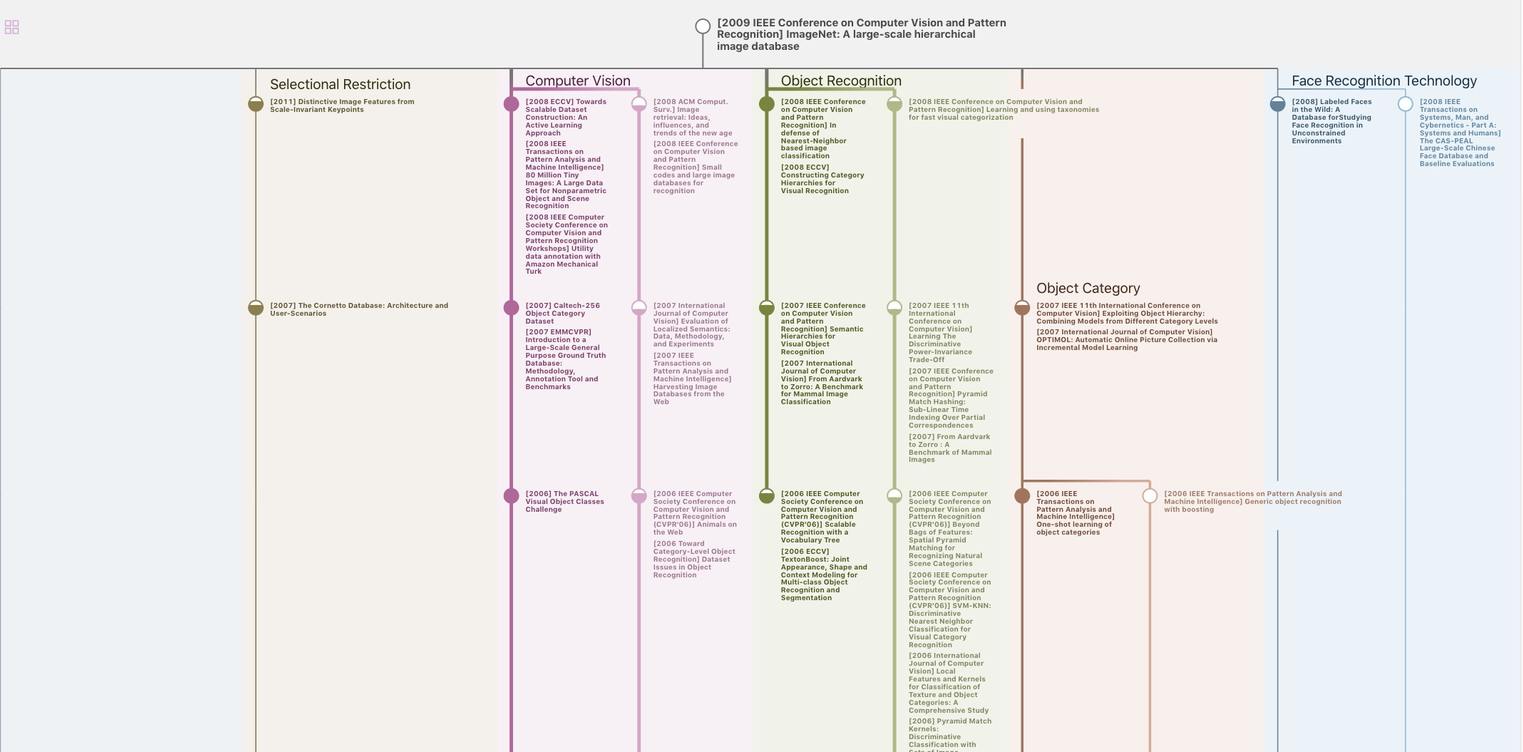
生成溯源树,研究论文发展脉络
Chat Paper
正在生成论文摘要