Dynamic artificial neural network model for ultralow temperature prediction in hydrogen storage tank
JOURNAL OF ENERGY STORAGE(2023)
摘要
Hydrogen fuel cell vehicles are gaining popularity as an alternative to electric vehicles. To support their use, research on hydrogen-storage devices and charging stations is being conducted actively. However, high-pressure hydrogen dispensing can lead to a temperature increase and potential explosion hazards. Therefore, maintaining safe hydrogen temperatures during charging is crucial. However, accurately predicting temperature changes in hydrogen heat exchangers is challenging owing to rapidly changing operating conditions and large temperature differences. In this study, we adopt a deep-learning-based artificial neural network for predicting temporal temperature variations in low-temperature hydrogen heat exchangers. Two networks, namely, the deep neural network (DNN) and recurrent neural network (RNN), are constructed with Bayesian optimization based on the experimentally measured data from an ultralow-temperature hydrogen heat exchanger to predict temperature. Both models showed superior prediction accuracies of temperature within 3.11 % of mean absolute error (MAE) and heat transfer rates of the heat exchanger within 2.53 % of MAE. In particular, DNN modeling is recommended as a universal method for predicting the temperature when previous data are known, and RNN modeling is recommended for predicting the temperature for successive actions in a compressed-hydrogen system.
更多查看译文
关键词
Deep-learning model,Dynamic artificial neural network,Recurrent neural network,Hydrogen storage,Compressed hydrogen
AI 理解论文
溯源树
样例
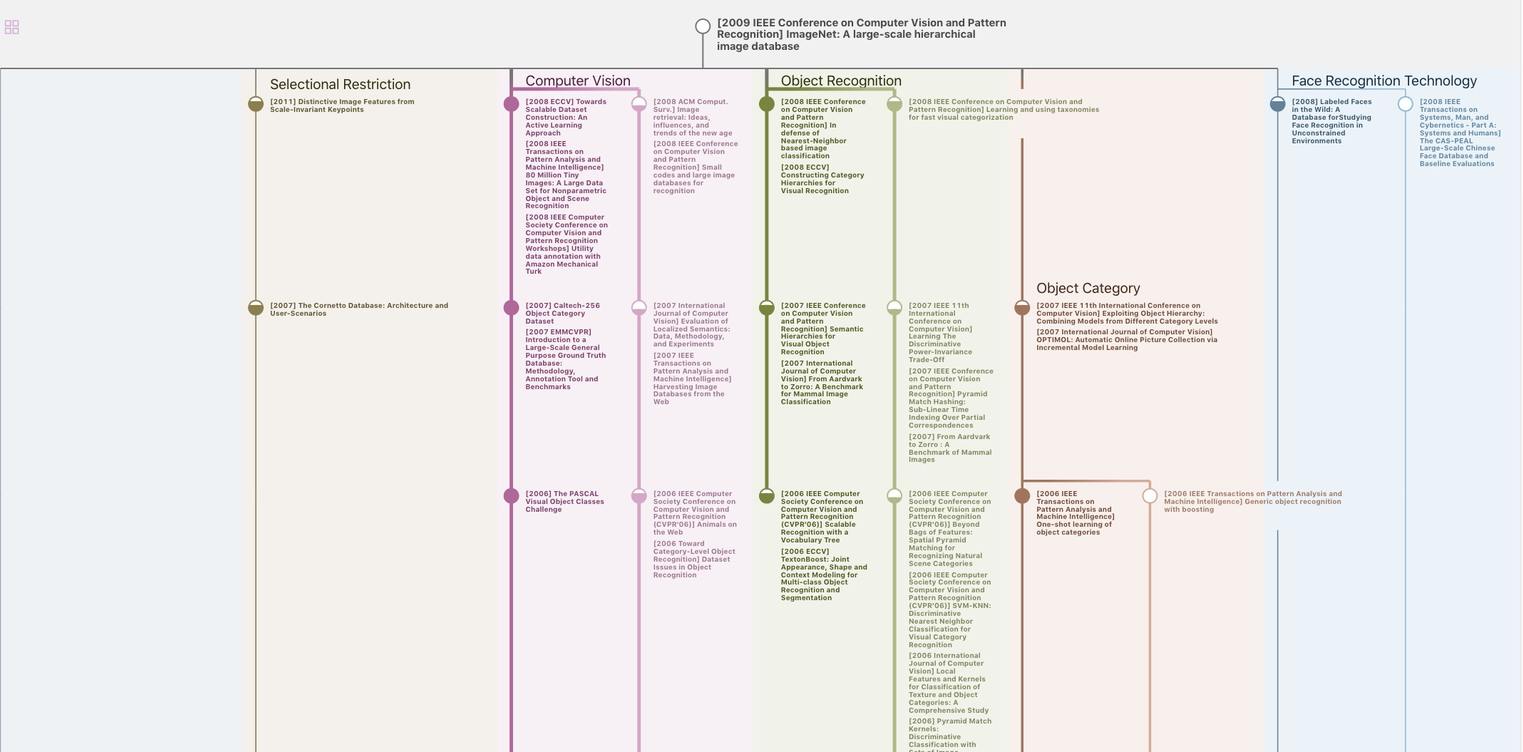
生成溯源树,研究论文发展脉络
Chat Paper
正在生成论文摘要