DIDR-Net: a sparse-view CT deep iterative reconstruction network with an independent detail recovery network
JOURNAL OF INSTRUMENTATION(2023)
摘要
Sparse-view CT is an effective method to reduce X-ray radiation dose in clinical CT imaging. However, sparse view image reconstruction is still challenging due to highly undersampled data. To this end, we propose a new deep learning-based reconstruction model of CT images, called DIDR-Net. Unlike existing methods, DIDR-Net employs a dual network structure, including an iterative reconstruction sub-network and a detail recovery sub-network. The iterative reconstruction sub-network expands the FISTA (Fast Iterative Soft Thresholding Algorithm) into a deep network, utilizing learnable nonlinear sparse transform and shrinkage thresholding to improve reconstruction performance. To avoid loss of image details while removing artifacts, we design a detail recovery sub-network. Specifically, this sub-network captures the local details and global information of the initial image through local and global branches, and adaptively fuses the results of these two branches through a fusion module. DIDR-Net generates both the initial reconstruction map and the detail feature map in a parallel manner, and finally fuses the two sides to reconstruct a high-quality CT image. Experimental results on the AAPM public dataset show that DIDR-Net exhibits better performance in both streak artifact removal and detail structure preservation compared to other advanced reconstruction algorithms.
更多查看译文
关键词
Computerized Tomography (CT) and Computed Radiography (CR), Image reconstruc-tion in medical imaging, Medical-image reconstruction methods and algorithms, computer-aided diagnosis
AI 理解论文
溯源树
样例
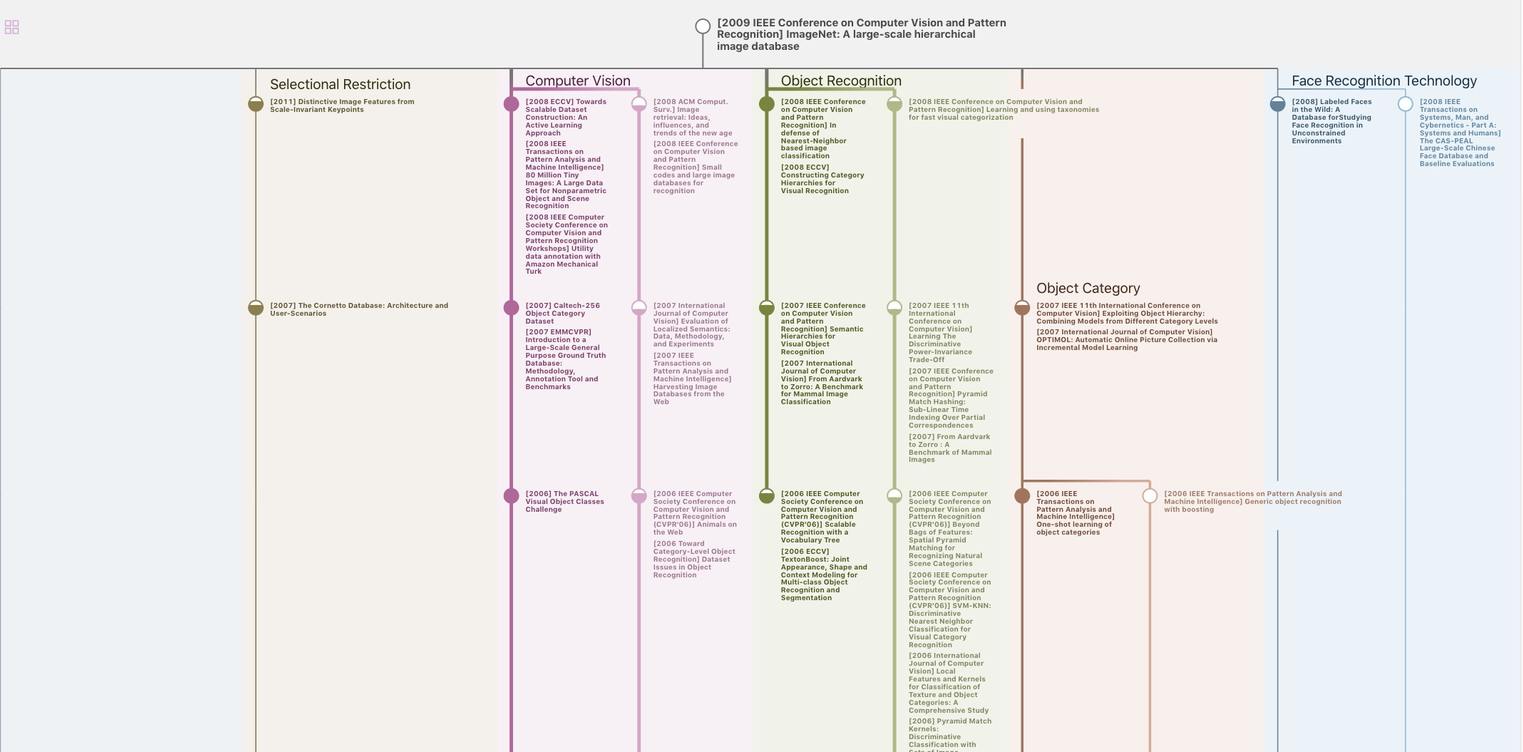
生成溯源树,研究论文发展脉络
Chat Paper
正在生成论文摘要