High-dimensional model averaging for quantile regression
CANADIAN JOURNAL OF STATISTICS-REVUE CANADIENNE DE STATISTIQUE(2023)
摘要
This article considers robust prediction issues in ultrahigh-dimensional (UHD) datasets and proposes combining quantile regression with sequential model averaging to arrive at a quantile sequential model averaging (QSMA) procedure. The QSMA method is made computationally feasible by employing a sequential screening process and a Bayesian information criterion (BIC) model averaging method for UHD quantile regression and provides a more accurate and stable prediction of the conditional quantile of a response variable. Meanwhile, the proposed method shows effective behaviour in dealing with prediction in UHD datasets and saves a great deal of computational cost with the help of the sequential technique. Under some suitable conditions, we show that the proposed QSMA method can mitigate overfitting and yields reliable predictions. Numerical studies, including extensive simulations and a real data example, are presented to confirm that the proposed method performs well.
更多查看译文
关键词
Bayesian information criterion, linear quantile regression, model averaging, quantile prediction error, ultrahigh-dimensional data
AI 理解论文
溯源树
样例
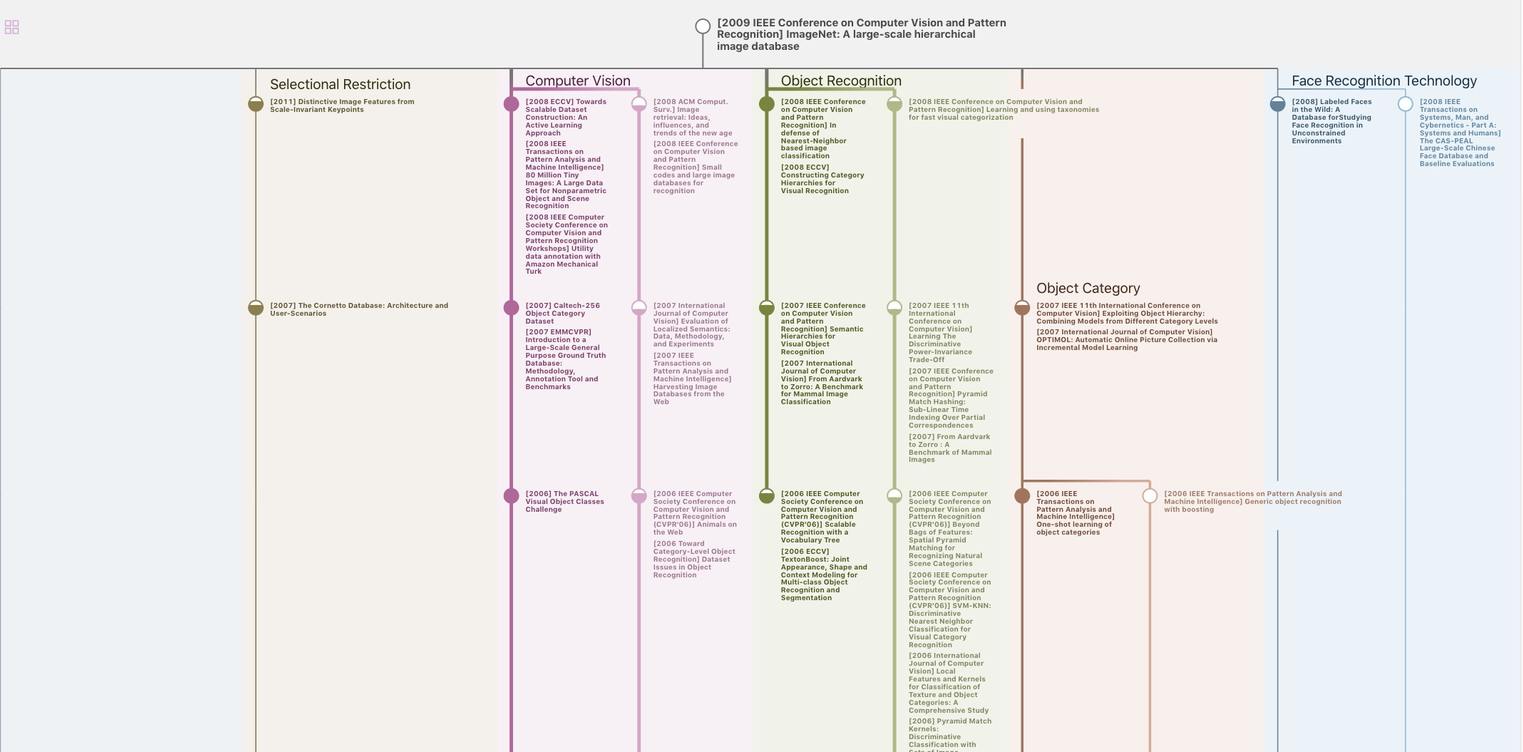
生成溯源树,研究论文发展脉络
Chat Paper
正在生成论文摘要