Simulated Gold-Standard for Quantitative Evaluation of Monocular Vision Algorithms
GEOSPATIAL INFORMATICS XIII(2023)
摘要
In the physical universe, truth for computer vision (CV) is impractical if not impossible to obtain. As a result, the CV community has resorted to qualitative practices and sub-optimal quantitative measures. This is problematic because it limits our ability to train, evaluate, and ultimately understand algorithms such as single image depth estimation (SIDE) and structure from motion (SfM). How good are these algorithms, individually and relatively, and where do they break? Herein, we discuss that while truth evades both the real and simulated (SIM) universes, a SIM CV gold-standard can be achieved. We outline an extensible SIM framework and data collection workflow using Unreal Engine with the Robot Operating System (ROS) for three dimensional mapping on low altitude aerial vehicles. Furthermore, voxel-based mapping measures from algorithm output to a SIM gold-standard are discussed. The proposed metrics are demonstrated by analyzing performance across changes in platform context. Ultimately, the current article is a step towards an improved process for comparing algorithms, evaluating their strengths and weaknesses, and automating algorithm design.
更多查看译文
关键词
point cloud, voxel, structure from motion, monocular depth estimation, single image depth estimation, unreal engine, simulation, ground-truth, gold-standard, XAI, evaluation
AI 理解论文
溯源树
样例
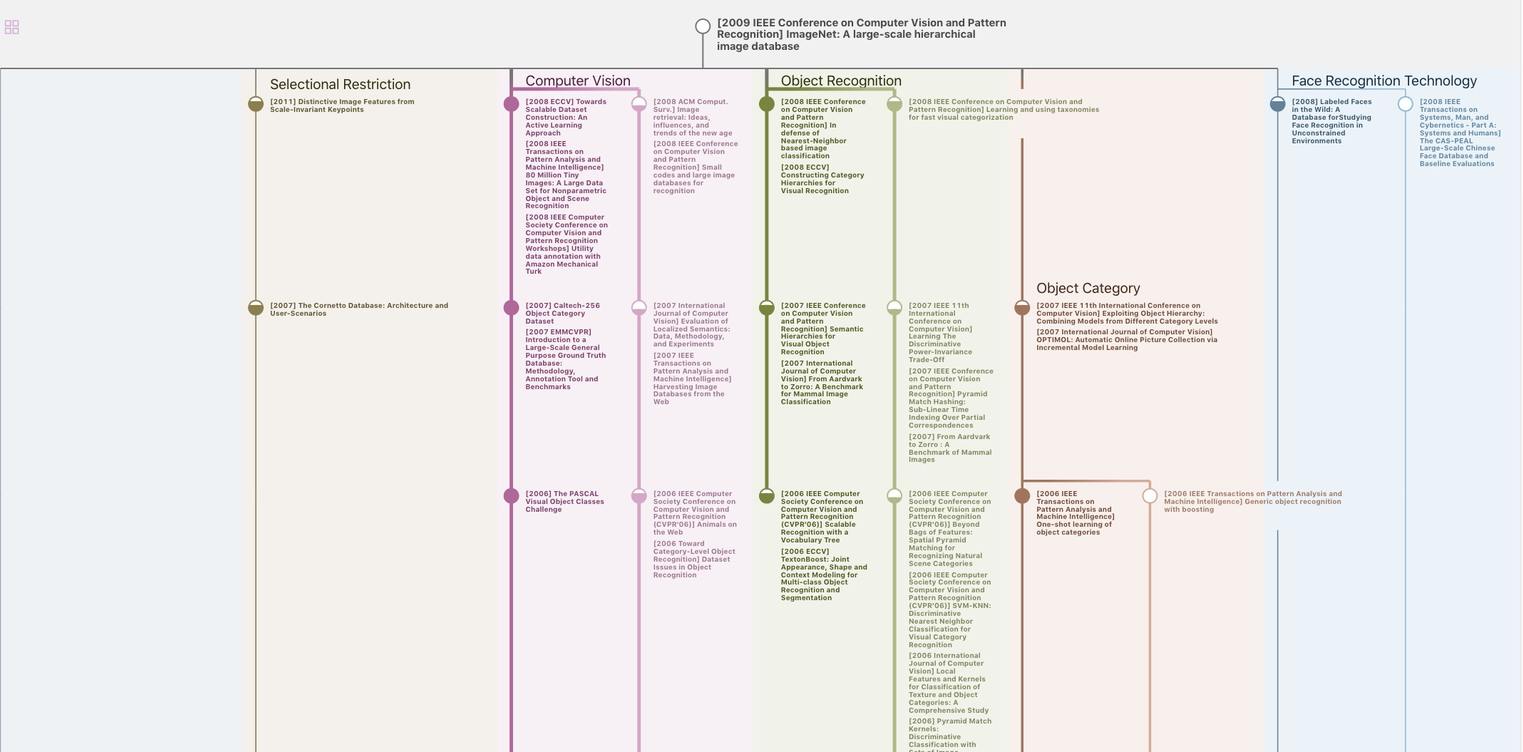
生成溯源树,研究论文发展脉络
Chat Paper
正在生成论文摘要