Efficient and Accurate Beach Litter Detection Method Based on QSB-YOLO
INTERNATIONAL JOURNAL OF ADVANCED COMPUTER SCIENCE AND APPLICATIONS(2023)
摘要
Because of the potential threats it presents to marine ecosystems and human health, beach litter is becoming a major global environmental issue. The traditional manual sampling survey of beach litter is poor in real-time, poor in effect, and limited in the detection area, so it is extremely difficult to quickly clean up and recycle beach litter. Deep learning technology is quickly advancing, opening up a new method for monitoring beach litter. A QSB-YOLO beach litter detection approach based on the improved YOLOv7 is proposed for the problem of missed and false detection in beach litter detection. First, YOLOv7 is combined with the quantization-friendly Quantization-Aware RepVGG (QARepVGG) to reduce the model's parameters while maintaining its performance advantage. Secondly, A Simple, Parameter-Free Attention Module (SimAM) is used in YOLOv7 to enhance the feature extraction capacity of the network for the image region of interest. Finally, improving the original neck by combining the concept of the Bidirectional Feature Pyramid Network (BiFPN) allows the network to better learn features of various sizes. The test results on the self-built dataset demonstrate that: (1) QSBYOLO has a good detection effect for six types of beach litter; (2) QSB-YOLO has a 5.8% higher mAP compared to YOLOv7, with a 43% faster detection speed, and QSB-YOLO has the highest detection accuracy for styrofoam, plastic products, and paper products; (3) QSB-YOLO has the greatest detection accuracy and detection efficiency when comparing the detection effects in various models. The results of the experiments demonstrate that the suggested model satisfies the need for beach litter identification in real-time.
更多查看译文
关键词
-Beach litter detection,QSB-YOLO,YOLOv7,Quantization-Aware RepVGG,a simple,parameter-free attention module,bidirectional feature pyramid network
AI 理解论文
溯源树
样例
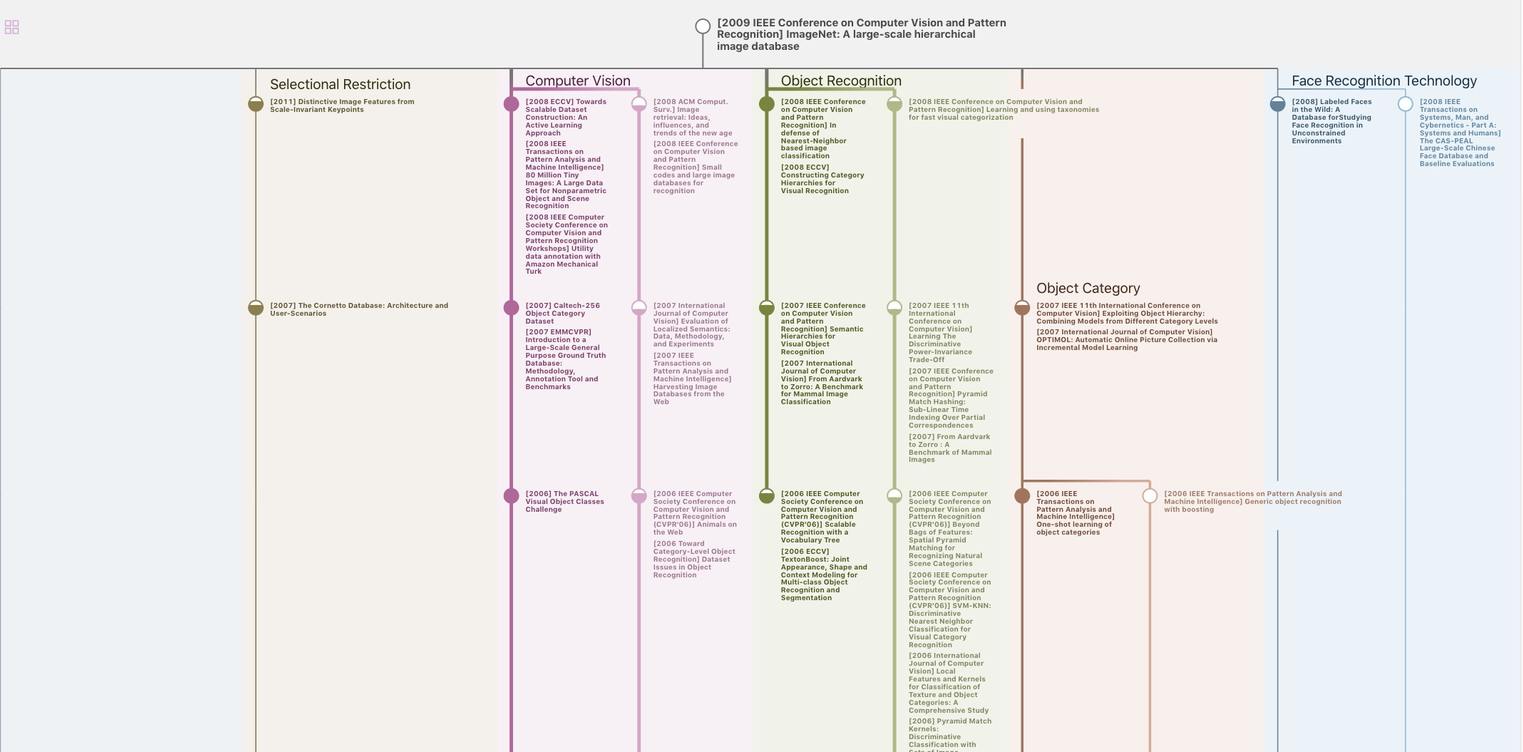
生成溯源树,研究论文发展脉络
Chat Paper
正在生成论文摘要