Crots: Cross-Domain Teacher-Student Learning for Source-Free Domain Adaptive Semantic Segmentation
INTERNATIONAL JOURNAL OF COMPUTER VISION(2024)
Abstract
Source-free domain adaptation (SFDA) aims to transfer source knowledge to the target domain from pre-trained source models without accessing private source data. Existing SFDA methods typically adopt the self-training strategy employing the pre-trained source model to generate pseudo-labels for unlabeled target data. However, these methods are subject to strict limitations: (1) The discrepancy between source and target domains results in intense noise and unreliable pseudo-labels. Overfitting noisy pseudo-labeled target data will lead to drastic performance degradation. (2) Considering the class-imbalanced pseudo-labels, the target model is prone to forget the minority classes. Aiming at these two limitations, this study proposes a CROss domain Teacher-Student learning framework (namely CROTS) to achieve source-free domain adaptive semantic segmentation. Specifically, with pseudo-labels provided by the intra-domain teacher model, CROTS incorporates Spatial Aware Data Mixing to generate diverse samples by randomly mixing different patches respecting to their spatial semantic layouts. Meanwhile, during inter-domain teacher-student learning, CROTS fosters Rare-Class Patches Mining strategy to mitigate the class imbalance phenomenon. To this end, the inter-domain teacher model helps exploit long-tailed rare classes and promote their contributions to student learning. Extensive experimental results have demonstrated that: (1) CROTS mitigates the overfitting issue and contributes to stable performance improvement, i.e., + 16.0% mIoU and + 16.5% mIoU for SFDA in GTA5?Cityscapes and SYNTHIA?Cityscapes, respectively; (2) CROTS improves task performance for longtailed rare classes, alleviating the issue of class imbalance; (3) CROTS achieves superior performance comparing to other SFDA competitors; (4) CROTS can be applied under the black-box SFDA setting, even outperforming many white-box SFDA methods. Our codes will be publicly available at https://github.com/luoxin13/CROTS.
MoreTranslated text
Key words
Domain adaptation,Transfer learning,Semantic segmentation
AI Read Science
Must-Reading Tree
Example
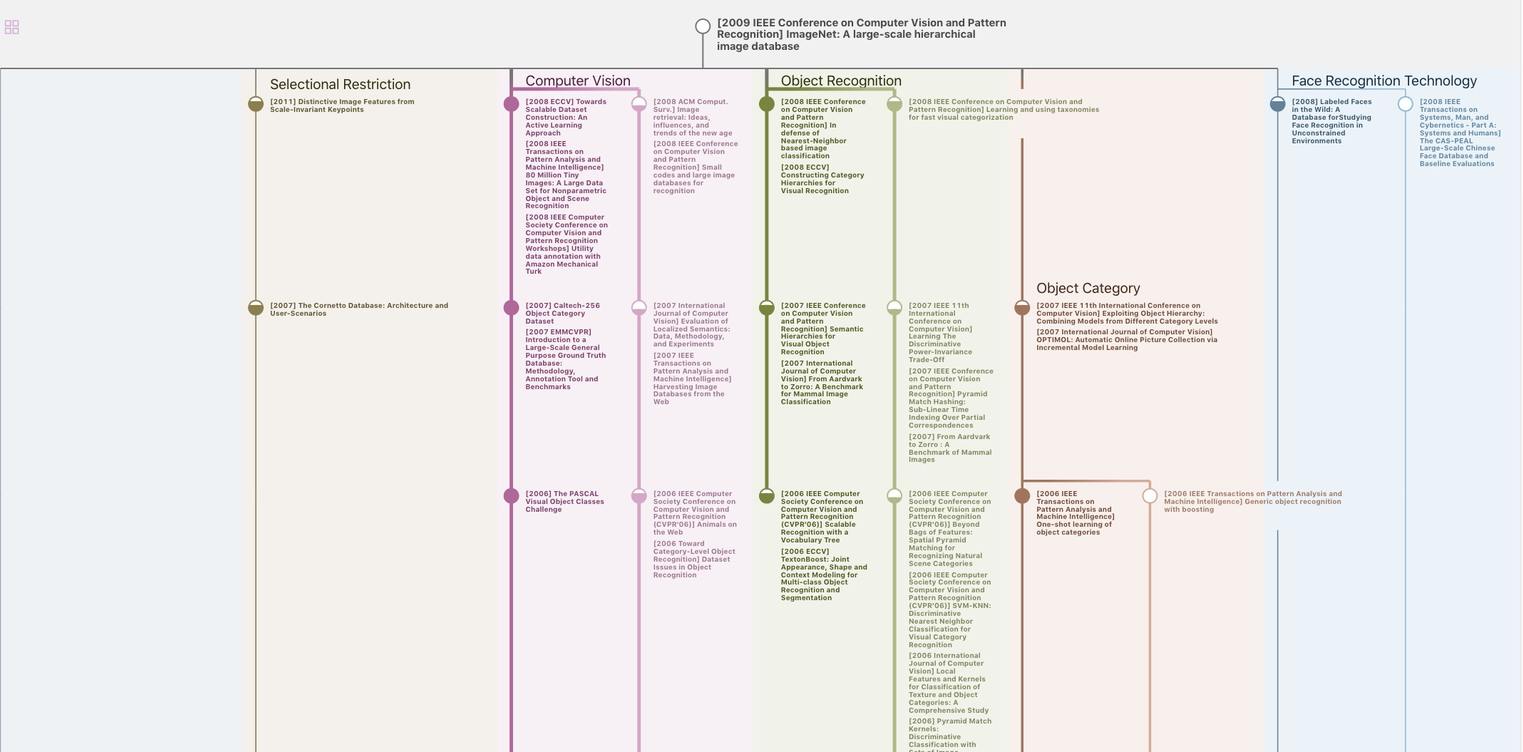
Generate MRT to find the research sequence of this paper
Chat Paper
Summary is being generated by the instructions you defined