MG-GCN: Multi-Granularity Graph Convolutional Neural Network for Multi-Label Classification in Multi-Label Information System
IEEE TRANSACTIONS ON EMERGING TOPICS IN COMPUTATIONAL INTELLIGENCE(2024)
摘要
Recently, many researchers have applied Graph Convolutional Neural Networks (GCN) to multi-label learning tasks by establishing relations among labels. However, these researchers have only used GCN as an auxiliary module and have lacked exploration of object relations, which has limited the inference capability of GCN. Applying GCN to multi-label classification in information systems poses significant challenges to address because information systems are considered as a graph with only nodes and no edges. This article proposes a multi-granularity graph convolutional neural network (MG-GCN) based on object relations to enhance the versatility of GCN and demonstrate its reasoning ability. The MG-GCN efficiently solves complex multi-label classification problems in information systems. Firstly, based on the multi-granularity thinking, the attribute set is divided into several attribute subsets based on granularity. Secondly, a graph of differences representing the relationship between objects is established under each attribute subset. Finally, based on the attention mechanism and multiple difference matrices of objects, MG-GCN is proposed and applied to multi-label classification within information systems. The effectiveness and significance of performance of MG-GCN has been verified through ranking-based indicators and hypothesis tests, respectively.
更多查看译文
关键词
Difference graph,MG-GCN,multi-label classification,multi-granularity,multi-label information system
AI 理解论文
溯源树
样例
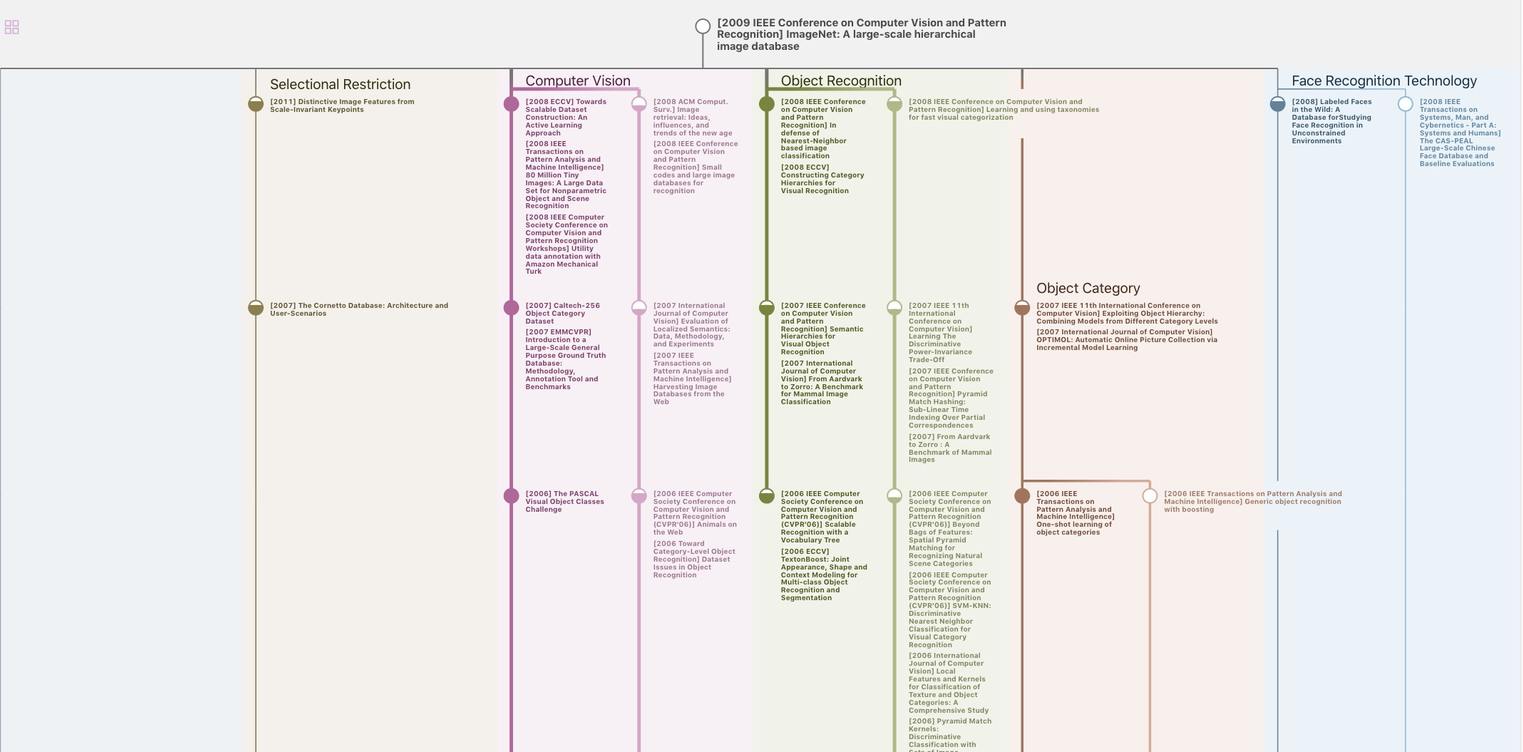
生成溯源树,研究论文发展脉络
Chat Paper
正在生成论文摘要