Topic-Clustering Model with Temporal Distribution for Public Opinion Topic Analysis of Geospatial Social Media Data
ISPRS INTERNATIONAL JOURNAL OF GEO-INFORMATION(2023)
摘要
Analysis of the spatiotemporal distribution of online public opinion topics can help understand the hotspots of public concern. The topic model is employed widely in public opinion topic clustering for social media data. In order to handle topic-clustering of low-quality geospatial social media data, such as microblog data, with short text and timeliness characteristics, this study proposed a Dirichlet multinomial mixture over time (DMMOT) model to cluster microblog topic for public opinion analysis. The DMMOT model assumes that a single document belongs to a single topic, in line with the characteristics of a short text, and it introduces the probability distribution of "topic-time" in the process of topic generation. The model parameter inference process was presented in detail by exploring the Gibbs sampling method. Results generated using the DMMOT model in case study show that the "topic-word" distribution is semantically aggregated within various topics, and "topic-time" distribution clustered within a time window under each topic. Furthermore, the characteristics of the trend of each topic over time are basically consistent with the corresponding trend of topic in reality in terms of content. These indicate that the DMMOT model improves topic clustering for short text to some extent. Furthermore, the DMMOT model performed well in both temporal and spatial analysis of public opinion topics based on microblog data.
更多查看译文
关键词
public opinion topic-clustering analysis,social media,public opinion
AI 理解论文
溯源树
样例
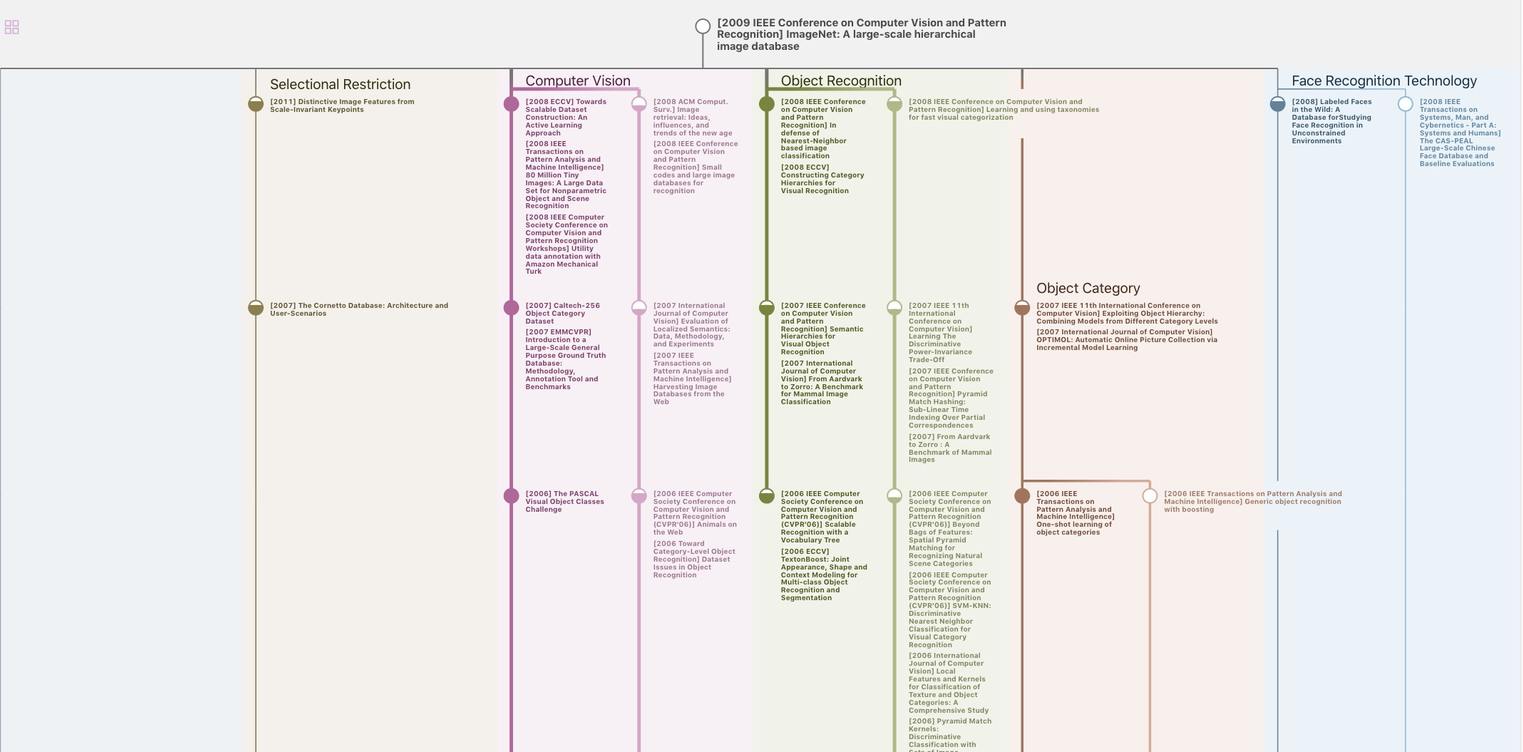
生成溯源树,研究论文发展脉络
Chat Paper
正在生成论文摘要