Developing a deep canonical correlation-based technique for seizure prediction
EXPERT SYSTEMS WITH APPLICATIONS(2023)
摘要
Proof-of-principle studies suggest that seizure prediction from non-invasive device recordings may be feasible. However, the discovery of optimal biomarkers is an ongoing research task. We aim to evaluate seizure-related differences in peripheral neurophysiological recordings by proposing a biomarker that allows classifying between pre-ictal and time-matched inter-ictal data from epilepsy patients. We include 42 pediatric epilepsy patients admitted for continuous video-EEG monitoring at Boston Children’s Hospital (21 with tonic-clonic seizures and 21 controls). With a wearable biosensor, we select 45 min of pre-ictal data from seizure patients and 45 min of time-matched data from control patients. We analyze electrodermal activity and heart rate recordings by three different architectures of deep canonical correlation analysis (DCCA)-based autoencoder. The clustering accuracy and improvement over chance are reported. The best DCCA technique is DCCA with a gated recurrent unit with a clustering accuracy of 68.9% and a relative improvement over chance of 37.8%. Clustering accuracy is better than chance for 71% of patients. Seizure prediction utilizing unsupervised clustering based on DCCA analysis of coupled changes in heart rate and electrodermal activity is feasible in a majority of patients with seizures.
更多查看译文
关键词
Deep canonical correlations,Machine learning,Wearables,Seizure monitoring,Epilepsy
AI 理解论文
溯源树
样例
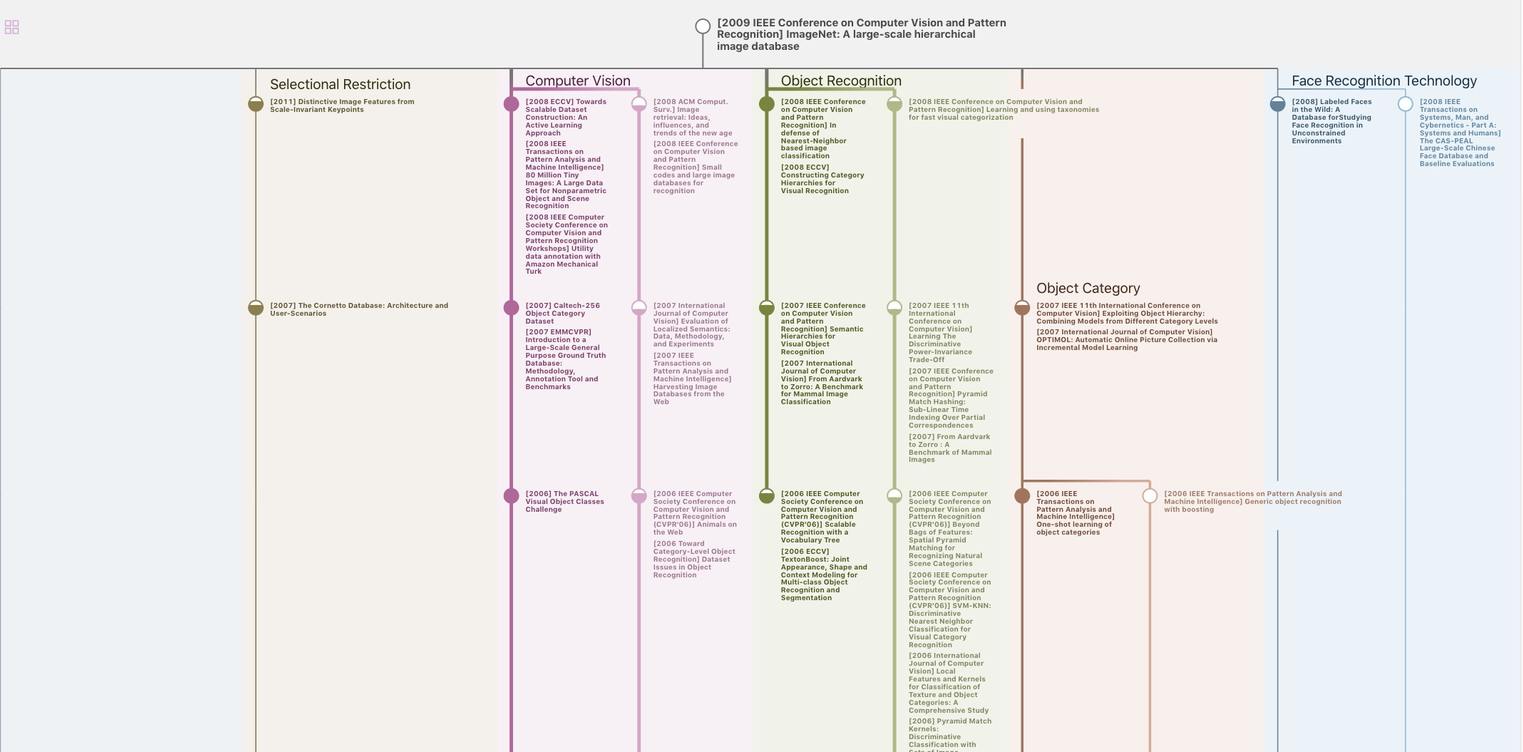
生成溯源树,研究论文发展脉络
Chat Paper
正在生成论文摘要