A patent recommendation method based on KG representation learning
ENGINEERING APPLICATIONS OF ARTIFICIAL INTELLIGENCE(2023)
摘要
The number of patents is constantly increasing, providing an invaluable knowledge base. But retrieving this knowledge becomes ever more challenging with the increase in data. In this context, this study proposes a patent recommendation method based on knowledge graph (KG) representation. Although KG based recommendation systems received broad attention in the literature, there is still a lack of literature that explores how it can be used for patent recommendations. First, and in terms of KG construction, the existing methods encounter issues when it comes to simultaneously accounting for accuracy and recall rate. This study proposes a combination of rule-based and deep learning methods for KG construction to solve this problem. Second, existing methods have performance and semantic association limitations when learning KG representations. This study therefore uses high-performance graph neural networks for encoding and models the semantic association limitations in graphs using traditional KG representation learning methods. Finally, existing recommendation systems usually focus on the semantic correlation between interaction items in recommendations, ignoring their direct relationships. This study therefore applies the KG representation learning method for the patent recommendation task, using KG representation learning to model the semantic association and constraints in the product-patent heterogeneous graph. Experiments based on a practical case example demonstrate that the proposed method outperforms baseline methods, and that it can guide innovative design processes. Findings have important implications for research and practice.
更多查看译文
关键词
Knowledge graph,Representation learning,Patent recommendation,Graph neural network
AI 理解论文
溯源树
样例
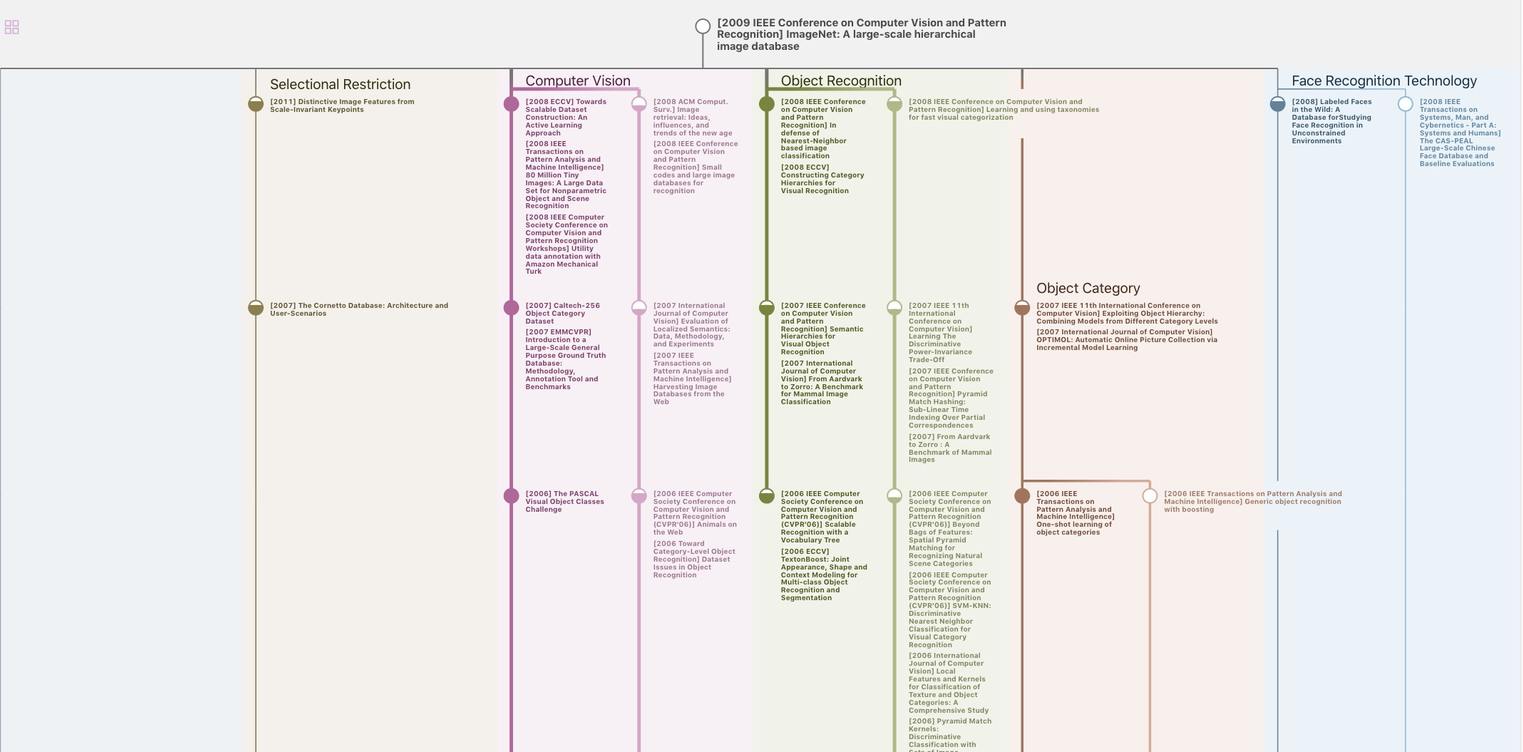
生成溯源树,研究论文发展脉络
Chat Paper
正在生成论文摘要