Fundus Image Enhancement via Semi-Supervised GAN and Anatomical Structure Preservation
IEEE TRANSACTIONS ON EMERGING TOPICS IN COMPUTATIONAL INTELLIGENCE(2024)
摘要
The fundus image is often used in clinical diagnosis due to ease and safety of acquisition, but the quality may be affected by environment and onsite operations. As low-quality medical images may lead to misinterpretation in diagnosis and analysis, it is important to improve quality of the improperly acquired fundus images. Unfortunately, the existing fundus image enhancement methods require task-specific prior knowledge or suffer from insufficient generalization ability. To cope with this issue, a generative adversarial network (GAN) based model is proposed, namely the semi-supervised GAN with anatomical structure preservation (SSGAN-ASP). Specifically, an anatomical structure extraction component is employed in the generator to guide the enhancement process by preserving both retinal and lesion structures, while color information in the fundus image is also preserved. The SSGAN-ASP model is evaluated and compared with the state-of-the-art methods for medical image enhancement on three popular datasets. In addition, it is applied in the pre-processing of retinal vessel segmentation and diabetic retinopathy grading tasks to show efficacy in computer-aided diagnosis. Experimental results demonstrate that visual quality of the enhanced image can be improved while better performance in clinical diagnosis is achieved with our proposed model by adopting the anatomical structure extraction component and preserving color information as well.
更多查看译文
关键词
Fundus image enhancement,anatomical structure,generative adversarial network,semi-supervised learning
AI 理解论文
溯源树
样例
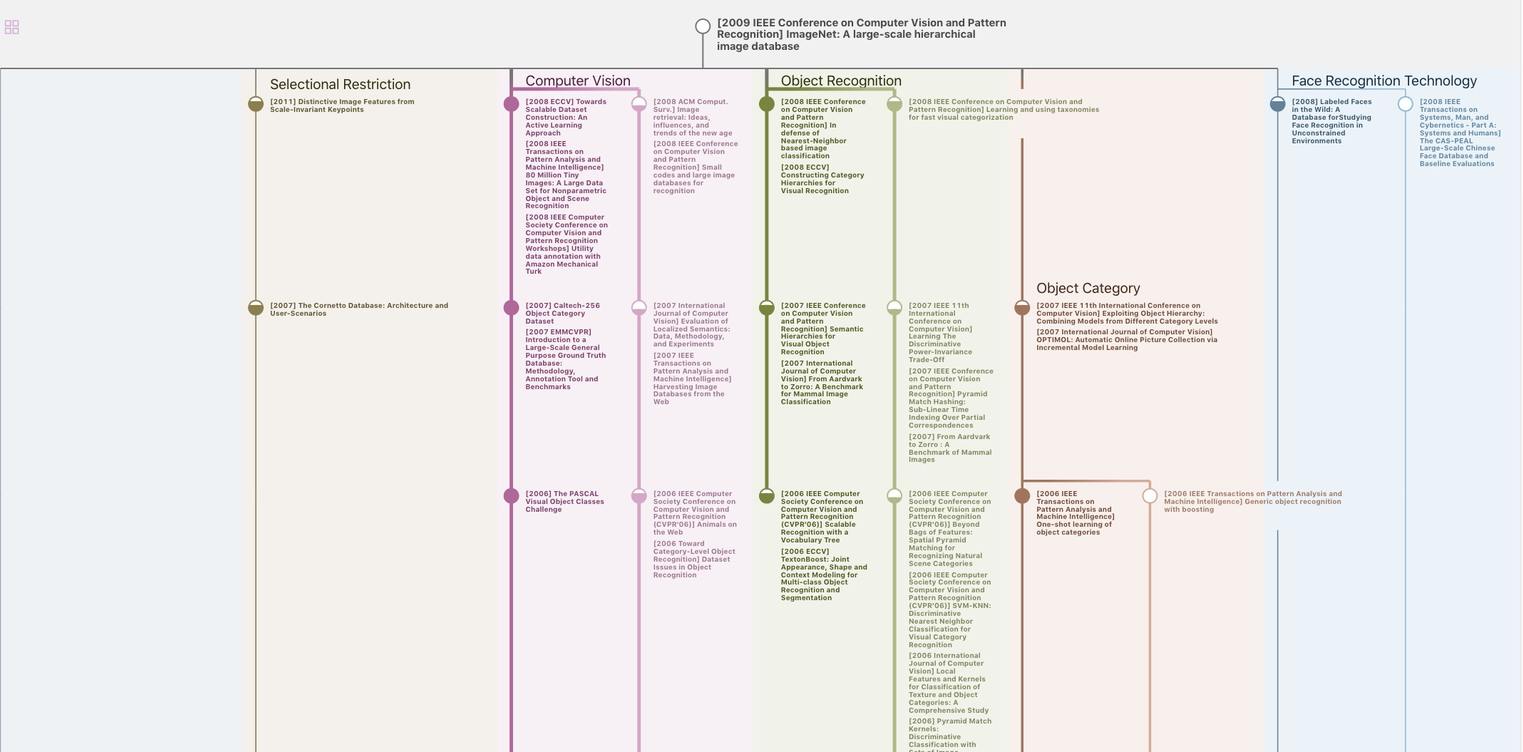
生成溯源树,研究论文发展脉络
Chat Paper
正在生成论文摘要