Imbalanced Learning in Heart Disease Categorization: Improving Minority Class Prediction Accuracy Using the SMOTE Algorithm
INTERNATIONAL JOURNAL OF FUZZY LOGIC AND INTELLIGENT SYSTEMS(2023)
摘要
In the field of medical data mining, imbalanced data categorization occurs frequently, which typically leads to classifiers with low predictive accuracy for the minority class. This study aims to construct a classifier model for imbalanced data using the SMOTE oversampling algorithm and a heart disease dataset obtained from Harapan Kita Hospital. The categorization model utilized logistic regression, decision tree, random forest, bagging logistic regression, and bagging decision tree. SMOTE improved the model prediction accuracy with imbalanced data, particularly for minority classes.
更多查看译文
关键词
Heart disease,Prediction,Imbalanced,Accuracy,SMOTE
AI 理解论文
溯源树
样例
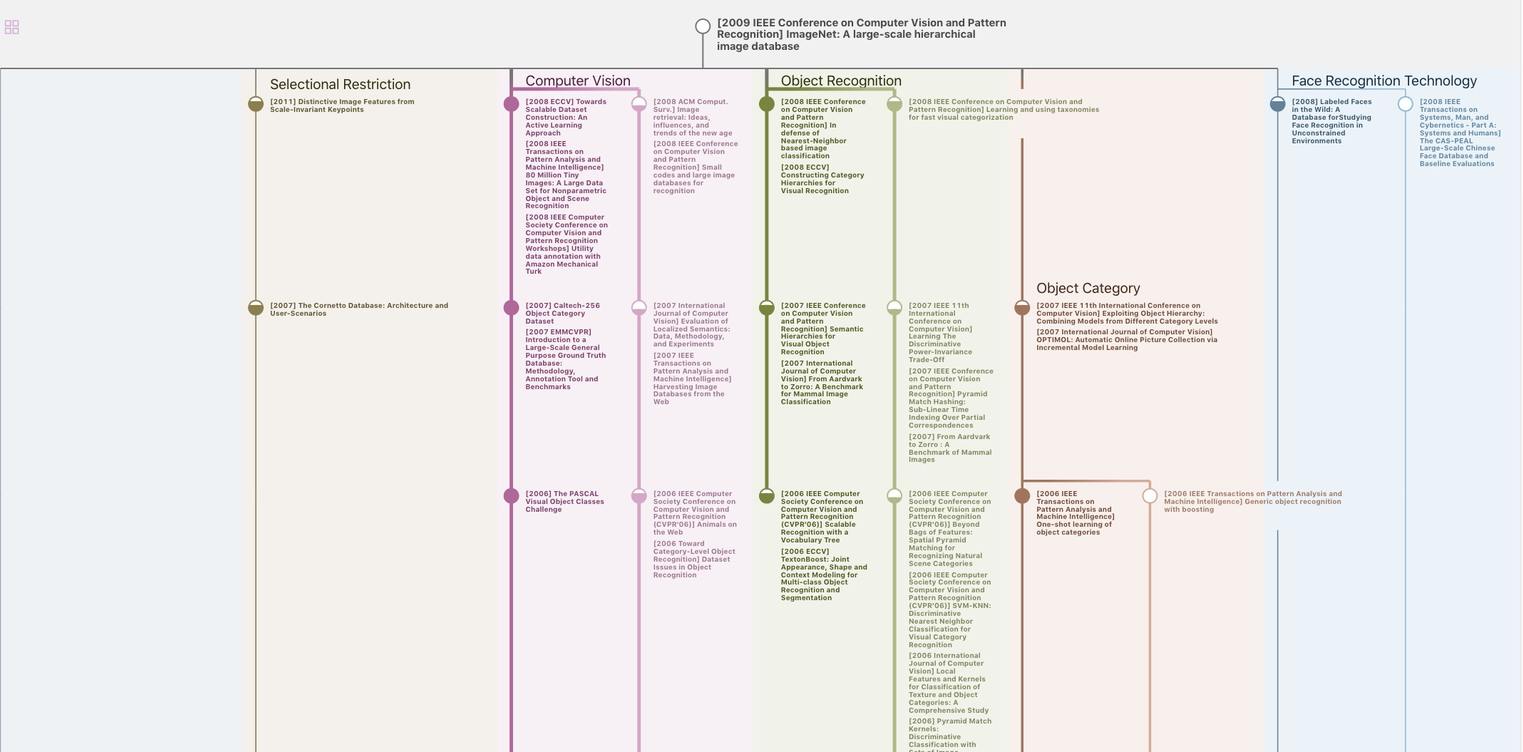
生成溯源树,研究论文发展脉络
Chat Paper
正在生成论文摘要