Theoretical Exploration of Flexible Transmitter Model
IEEE TRANSACTIONS ON NEURAL NETWORKS AND LEARNING SYSTEMS(2024)
摘要
Neural network models generally involve two important components, i.e., network architecture and neuron model. Although there are abundant studies about network architectures, only a few neuron models have been developed, such as the MP neuron model developed in 1943 and the spiking neuron model developed in the 1950s. Recently, a new bio-plausible neuron model, Flexible Transmitter (FT) model, has been proposed. It exhibits promising behaviors, particularly on temporal-spatial signals, even when simply embedded into the common feedforward network architecture. This paper attempts to understand the properties of the FT network (FTNet) theoretically. Under mild assumptions, we show that: i) FTNet is a universal approximator; ii) the approximation complexity of FTNet can be exponentially smaller than those of commonly-used real-valued neural networks with feedforward/recurrent architectures and is of the same order in the worst case; iii) any local minimum of FTNet is the global minimum, implying that it is possible to identify global minima by local search algorithms.
更多查看译文
关键词
Approximation complexity,flexible transmitter (FT) model,local minimum,neural networks.
AI 理解论文
溯源树
样例
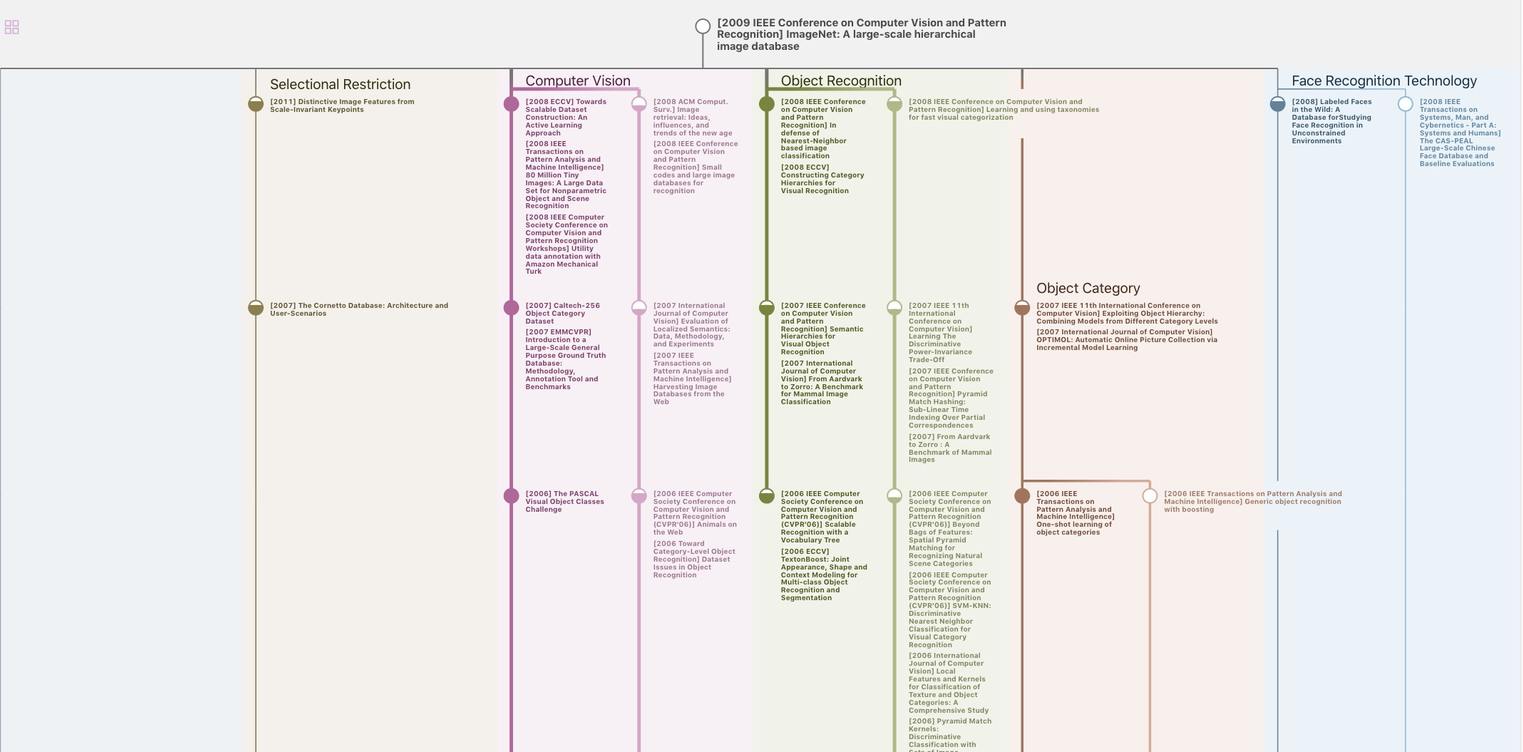
生成溯源树,研究论文发展脉络
Chat Paper
正在生成论文摘要